Learning Rules from Incomplete Examples via Observation Models
semanticscholar(2011)
摘要
We study the problem of learning general rules from concrete facts extracted from natural data sources such as the newspaper stories and medical histories. Natural data sources present two challenges to automated learning, namely, radical incompletenessand systematic bias . In previous work we proposed an approach that combines simultaneous learning of multiple predictive rules with differential scoring of evidence based on implicit observation models to address the above problems. In this paper, we further evaluate our approach empirically on natural datasets based on both textual and non-textual sources. We present a theoretical analysis that elucidates our approach and explains the empirical results. 1
更多查看译文
AI 理解论文
溯源树
样例
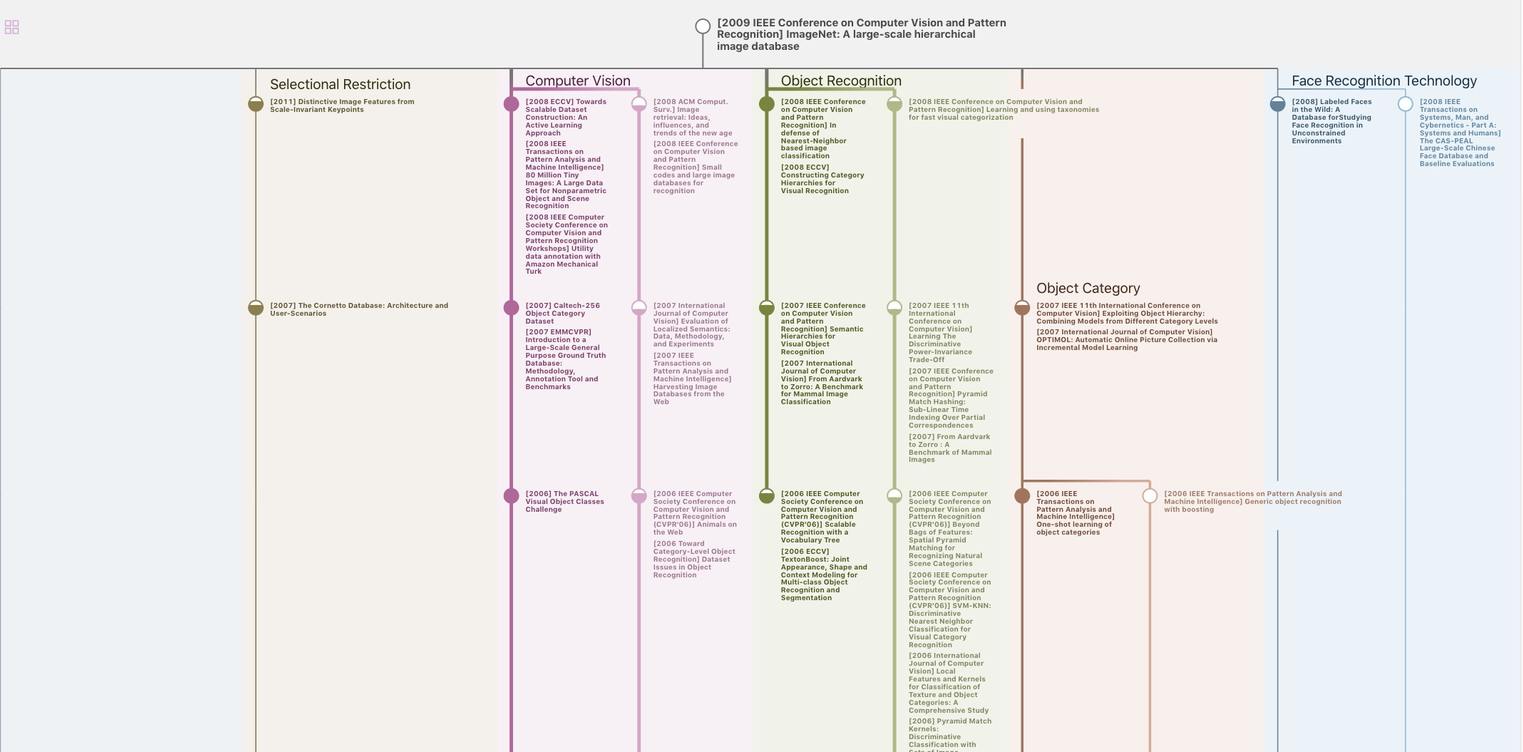
生成溯源树,研究论文发展脉络
Chat Paper
正在生成论文摘要