Attributes Correspondence Discovery in Ontology Instance-based Matching and RDF Data Linkage using Clustering Method
semanticscholar(2018)
摘要
One important aspect of ontology instance matching process is elements or attributes discovery. It specifies element correspondences in order to produce potential matching elements; otherwise, all elements of a class in the source ontology have to be compared with all elements of class in the target ontology. This heavy comparison is time-consuming and resulted in the poor performance of the matching system and makes the matching incomplete. Matching two or more ontologies and RDF datasets requires complete instance matching so as to establish logically equivalent relation among semantically related entities of the data sources. This deems challenging because of the existence of semantic heterogeneity and presence of irregular data in the RDF data sources which makes elements discovery and feature value extractions difficult. Thus, we proposed a four-step elements discovery method that utilizes unsupervised K-Medoids clustering algorithm in discovering potential matching elements pairs. To ensure generalization, we take unsupervised Canopy Clustering method to be the baseline for our evaluation. In terms of scalability, our method outperforms the baseline method with approximately 99% in both Pair Completeness and Reduction Ratio as against 60% and 86% respectively in the baseline. In mapping pattern generation, our method also outperforms the baseline algorithm with the overall F-Measure of ~91% against ~85%. The result of comparism with other methods justifies the significance effect of clustering attributes in the initial stage of the instance matching which can save about 50% of the comparism.
更多查看译文
AI 理解论文
溯源树
样例
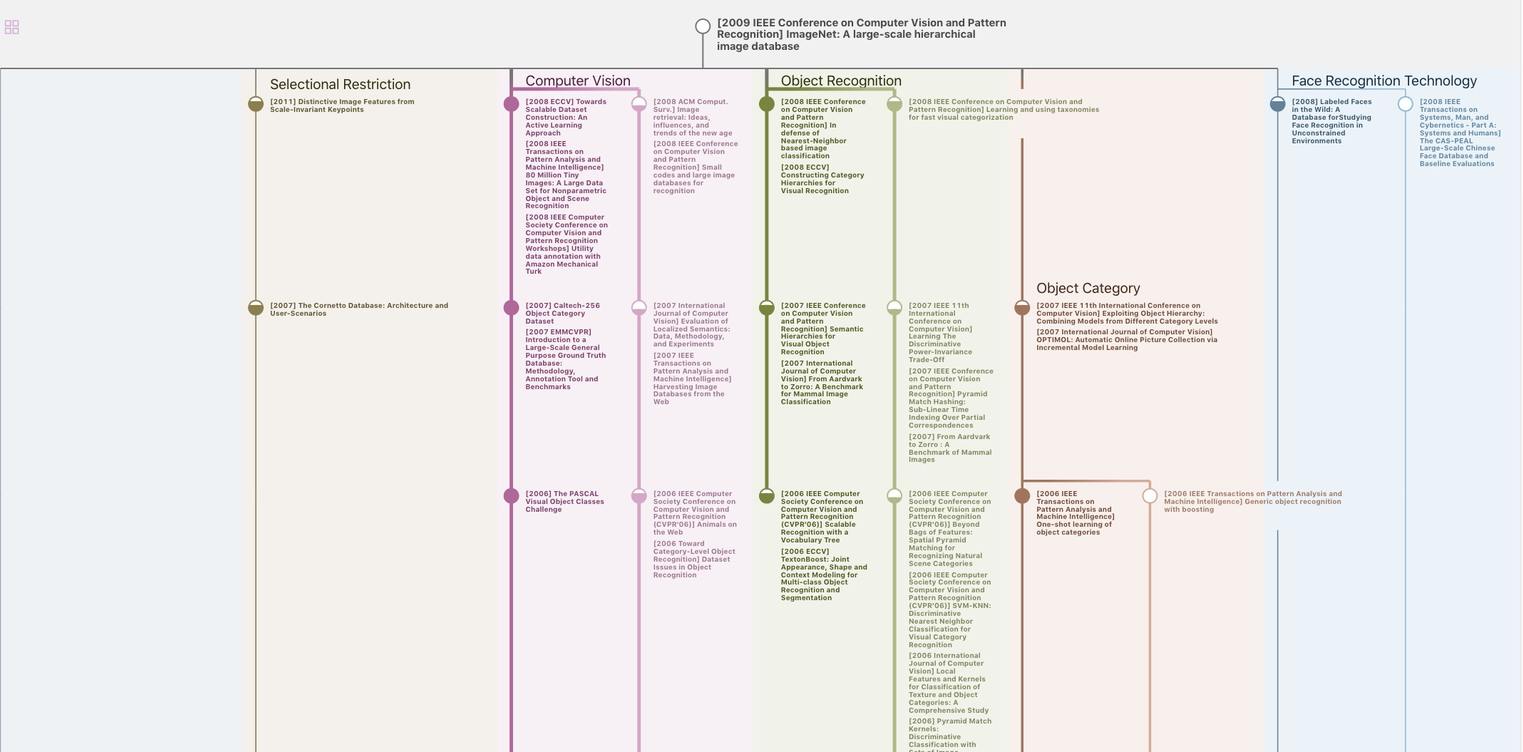
生成溯源树,研究论文发展脉络
Chat Paper
正在生成论文摘要