Quantum Walk Neural Networks
semanticscholar(2017)
摘要
In recent years, neural network approaches have performed extremely well in diverse fields ranging from image recognition and classification [1] to natural language processing [2]. A key reason for the recent successes of neural network methods in the aforementioned domains is due to the statistical properties of the data in these domains, namely stationarity and constitutionality [3]. However, when the data in question is graph-structured, neural network techniques need to be adapted because the aforementioned properties might not hold. Despite the technical difficulties, new neural networks that operate on graph structured data have sprung into prominence [3], [4], [5]. These approaches use the graph Laplacian, random walk matrix, or their corresponding spectra as an operator to diffuse information across the graph and then learn a task-specific weighting on the diffused information. In this work, we propose quantum walk neural networks (QWNN), a new graph neural network architecture based on quantum random walks. A quantum random walk differs from a classical random walk in that the walker’s state is expressed as a superposition rather than a probability distribution. Additionally, a coin operator acts on the walker at each step in the walk. Unlike previous graph neural networks, our approach uses this coin to directly learn a diffusion operator. We show that our quantum walk based neural network approach obtains competitive results when compared to other graph neural network approaches, suggesting that quantum techniques should be investigated further in the domain of graph-structured data.
更多查看译文
AI 理解论文
溯源树
样例
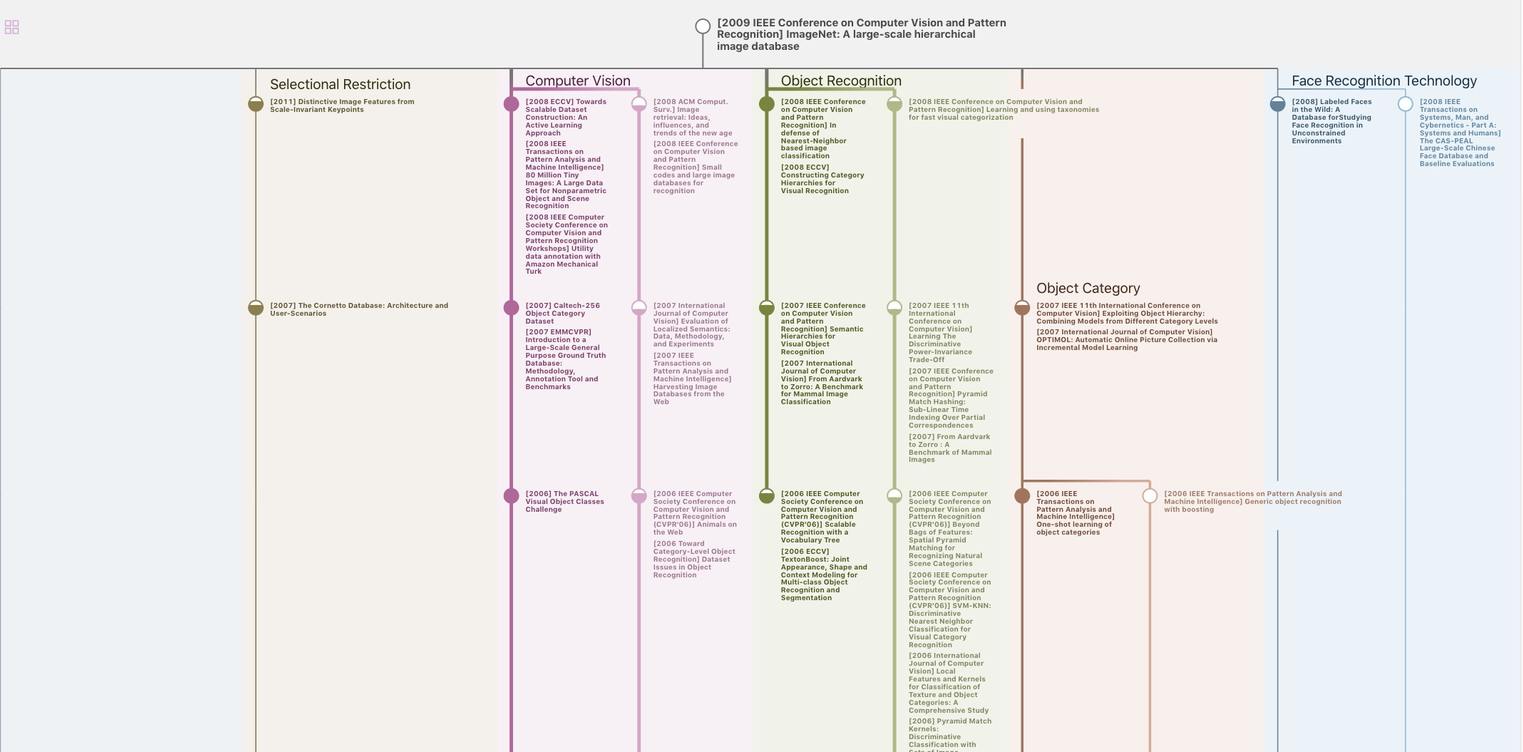
生成溯源树,研究论文发展脉络
Chat Paper
正在生成论文摘要