2014 Ieee Cis Awards
semanticscholar(2014)
摘要
Many real world problems can be formulated as optimization problems with various parameters to be optimized. Some problems only have one objective to be optimized, some may have multiple objectives to be optimized at the same time and some need to be optimized subjecting to one or more constraints. Thus numerous optimization algorithms have been proposed to solve these problems. Particle Swarm Optimizer (PSO) is a relatively new optimization algorithm which has shown its strength in the optimization world. This thesis presents two PSO variants, Comprehensive Learning Particle Swarm Optimizer (CLPSO) and Dynamic Multi-Swarm Particle Swarm Optimizer (DMS-PSO), which have good global search ability and can solve complex multi-modal problems for single objective optimization. The latter one is extended to solve constrained optimization and multi-objective optimization problems successfully with a novel constraint-handling mechanism and a novel updating criterion respectively. Subsequently, DMS-PSO is applied to determine the Bragg wavelengths of the sensors in an FBG sensor network and a tree search structure is designed to improve the accuracy and reduce the computation cost. Outstanding Chapter Award IEEE CIS UKRI Chapter, UK For promoting and supporting the dissemination of computational intelligence within the UKRI Section. IEEE Transactions on Neural Networks Outstanding Paper Award Long Cheng, Zeng-Guang Hou, Yingzi Lin, Min Tan, Wenjun Chris Zhang, Fang-Xiang Wu for their paper entitled “Recurrent Neural Network for Non-Smooth Convex Optimization Problems with Application to the Identification of Genetic Regulatory Networks”, vol. 22, no. 5, pp. 714–726, May 2011. Digital Object Identifier: 10.1109/ TNN.2011.2109735 Abstract—A recurrent neural network is proposed for solving the nonsmooth convex optimization problem with the convex inequality and linear equality constraints. Since the objective function and inequality constraints may not be smooth, the Clarke’s generalized gradients of the objective function and inequality constraints are employed to describe the dynamics of the proposed neural network. It is proved that the equilibrium point set of the proposed neural network is equivalent to the optimal solution of the original optimization problem by using the Lagrangian saddle-point theorem.
更多查看译文
AI 理解论文
溯源树
样例
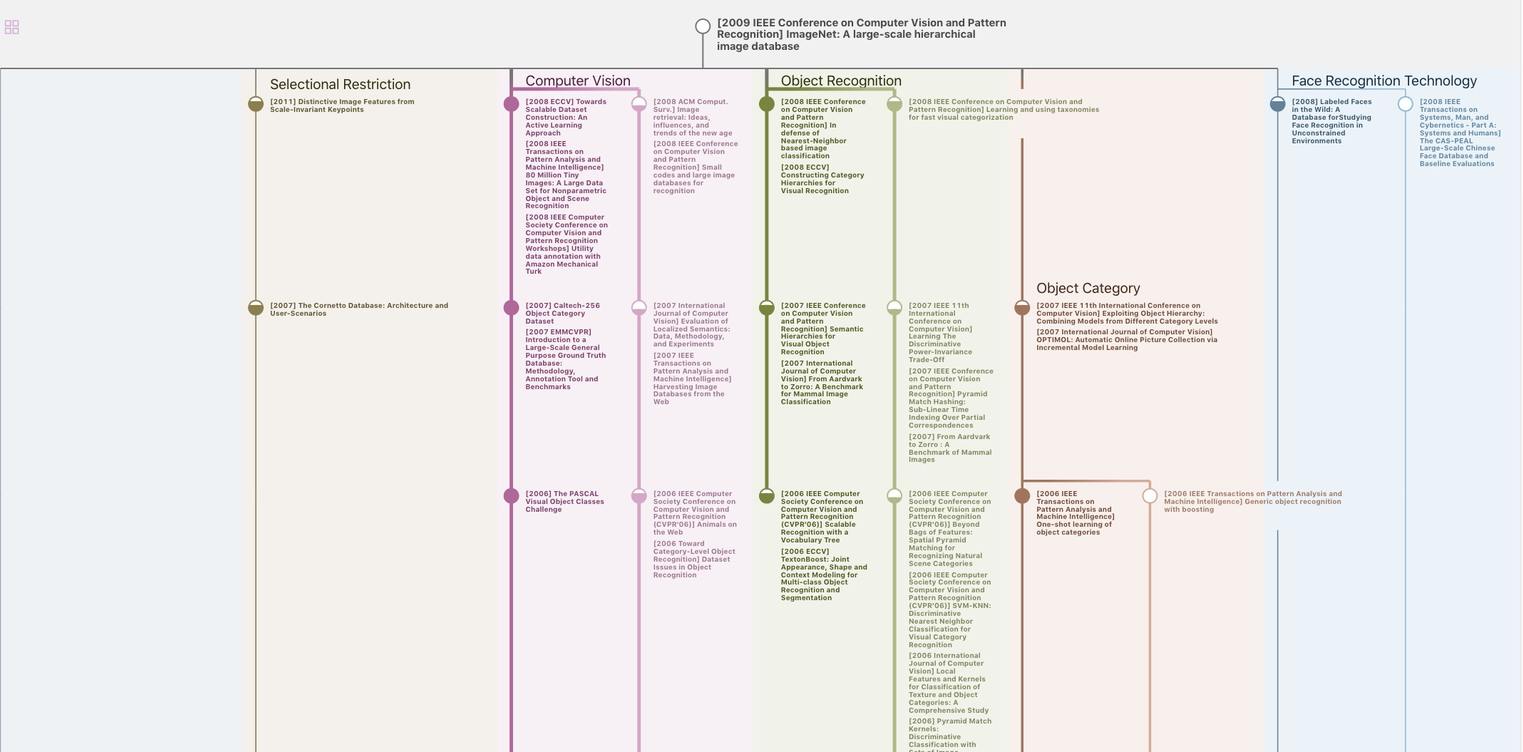
生成溯源树,研究论文发展脉络
Chat Paper
正在生成论文摘要