AM Abstractions from Sensor Data with Complex Event Processing and Machine Learning
semanticscholar(2018)
摘要
ions from Sensor Data with Complex Event Processing and Machine Learning Markus Stocker University of Eastern Finland, markus.stocker@uef.fi Mauno Rönkkö University of Eastern Finland, mauno.ronkko@uef.fi Mikko Kolehmainen University of Eastern Finland, mikko.kolehmainen@uef.fi Follow this and additional works at: https://scholarsarchive.byu.edu/iemssconference Part of the Civil Engineering Commons, Data Storage Systems Commons, Environmental Engineering Commons, Hydraulic Engineering Commons, and the Other Civil and Environmental Engineering Commons This Event is brought to you for free and open access by the Civil and Environmental Engineering at BYU ScholarsArchive. It has been accepted for inclusion in International Congress on Environmental Modelling and Software by an authorized administrator of BYU ScholarsArchive. For more information, please contact scholarsarchive@byu.edu, ellen_amatangelo@byu.edu. Stocker, Markus; Rönkkö, Mauno; and Kolehmainen, Mikko, "Abstractions from Sensor Data with Complex Event Processing and Machine Learning" (2014). International Congress on Environmental Modelling and Software. 8. https://scholarsarchive.byu.edu/iemssconference/2014/Stream-F/8 International Environmental Modelling and Software Society (iEMSs) 7th Intl. Congress on Env. Modelling and Software, San Diego, CA, USA, Daniel P. Ames, Nigel W.T. Quinn and Andrea E. Rizzoli (Eds.) http://www.iemss.org/society/index.php/iemss-2014-proceedings Abstractions from Sensor Data with Complex Event Processing and Machine Learningions from Sensor Data with Complex Event Processing and Machine Learning Markus Stocker, Mauno Rönkkö, Mikko Kolehmainen University of Eastern Finland, P.O. Box 1627, 70211 Kuopio, Finland markus.stocker@uef.fi, mauno.ronkko@uef.fi, mikko.kolehmainen@uef.fi Abstract: Environmental knowledge systems that build on sensor-based environmental monitoring rely on techniques in knowledge acquisition and representation to interpret the numbers obtained in measurement for what they tell about the monitored environment. Languages and systems in knowledge representation and reasoning, specifically Semantic Web technologies, support the formulation and execution of rules, a technique that enables deductive inference in a knowledge base. This technique has been used to demonstrate inference on sensor data. While the approach certainly has its merits, it is often demonstrated for numerical thresholds and, thus, for relatively trivial “semantic enrichment.” In reality, knowledge acquisition tasks of interest to environmental knowledge systems that build on sensor-based environmental monitoring are often more challenging. They rely on advanced computational techniques and models, e.g. in machine learning or complex event processing. In order to ease the formulation and execution of such tasks, systems need to integrate such techniques. Towards this end, we present the integration of machine learning with WEKA and complex event processing with Esper in Wavellite. Environmental knowledge systems that build on sensor-based environmental monitoring rely on techniques in knowledge acquisition and representation to interpret the numbers obtained in measurement for what they tell about the monitored environment. Languages and systems in knowledge representation and reasoning, specifically Semantic Web technologies, support the formulation and execution of rules, a technique that enables deductive inference in a knowledge base. This technique has been used to demonstrate inference on sensor data. While the approach certainly has its merits, it is often demonstrated for numerical thresholds and, thus, for relatively trivial “semantic enrichment.” In reality, knowledge acquisition tasks of interest to environmental knowledge systems that build on sensor-based environmental monitoring are often more challenging. They rely on advanced computational techniques and models, e.g. in machine learning or complex event processing. In order to ease the formulation and execution of such tasks, systems need to integrate such techniques. Towards this end, we present the integration of machine learning with WEKA and complex event processing with Esper in Wavellite.
更多查看译文
AI 理解论文
溯源树
样例
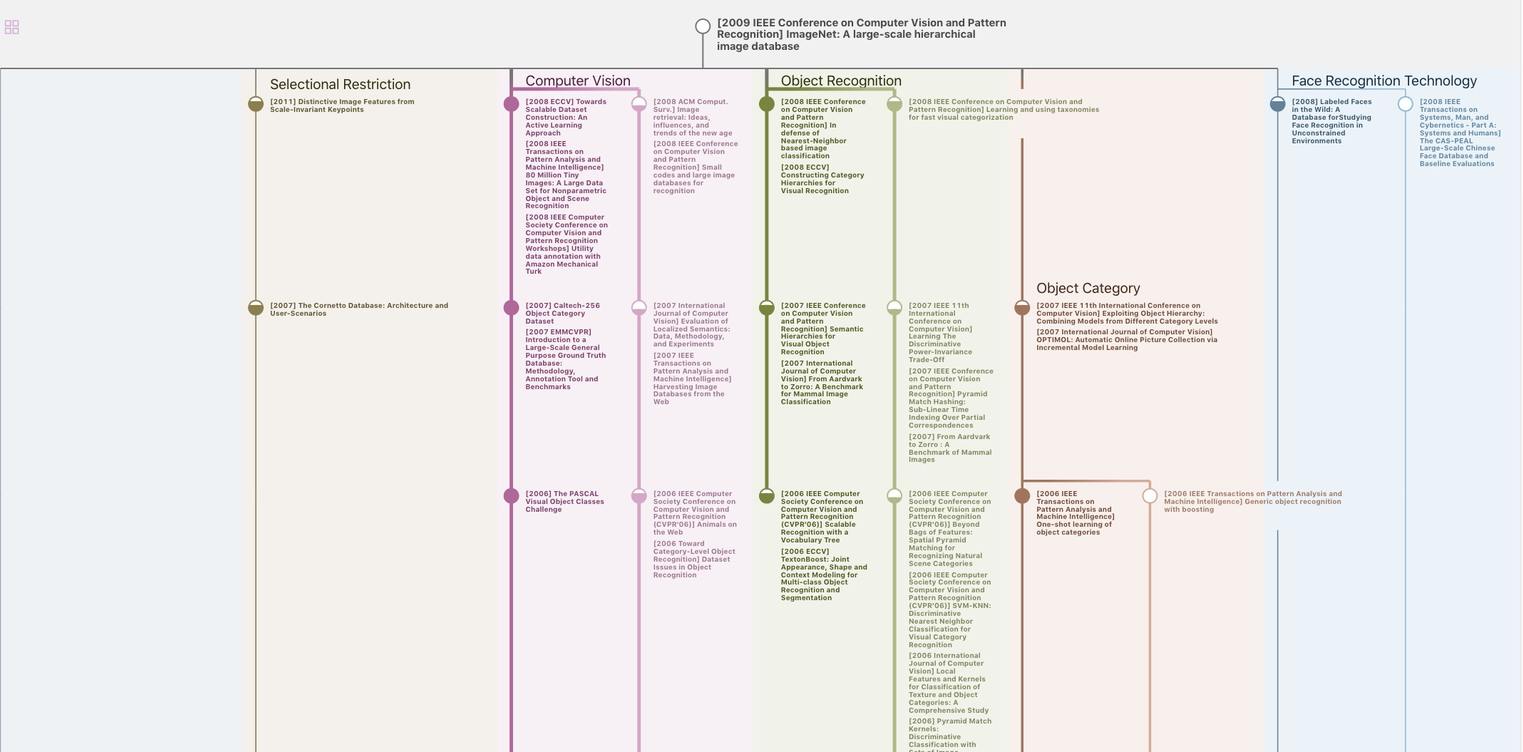
生成溯源树,研究论文发展脉络
Chat Paper
正在生成论文摘要