Towards using transparency mechanisms to build better mental models
semanticscholar(2019)
摘要
Non-expert users often do not have accurate mental models of robots, leading to failures during interactive task learning. We first observe that the information required to identify the cause of interaction failures, can be classified into commonly-defined, uncommonly-defined and hidden features. We then implemented two transparency mechanisms, question-answering and visual explanation capabilities, through which a non-expert user can access the robot’s internal reasoning. We conducted a user study (N=64) where we measured the ability of the user to identify the reason for 12 distinct interaction failures. We first characterize the impact of the identified features of interaction failures, and then test the effect of the two transparency mechanisms on the user’s performance. Results show that transparency mechanisms have the most influence on interaction failures with uncommonly-defined and hidden features. These results confirm that transparency mechanisms are effective in improving the user’s performance, leading to a higher accuracy in the user’s mental model of the robot.
更多查看译文
AI 理解论文
溯源树
样例
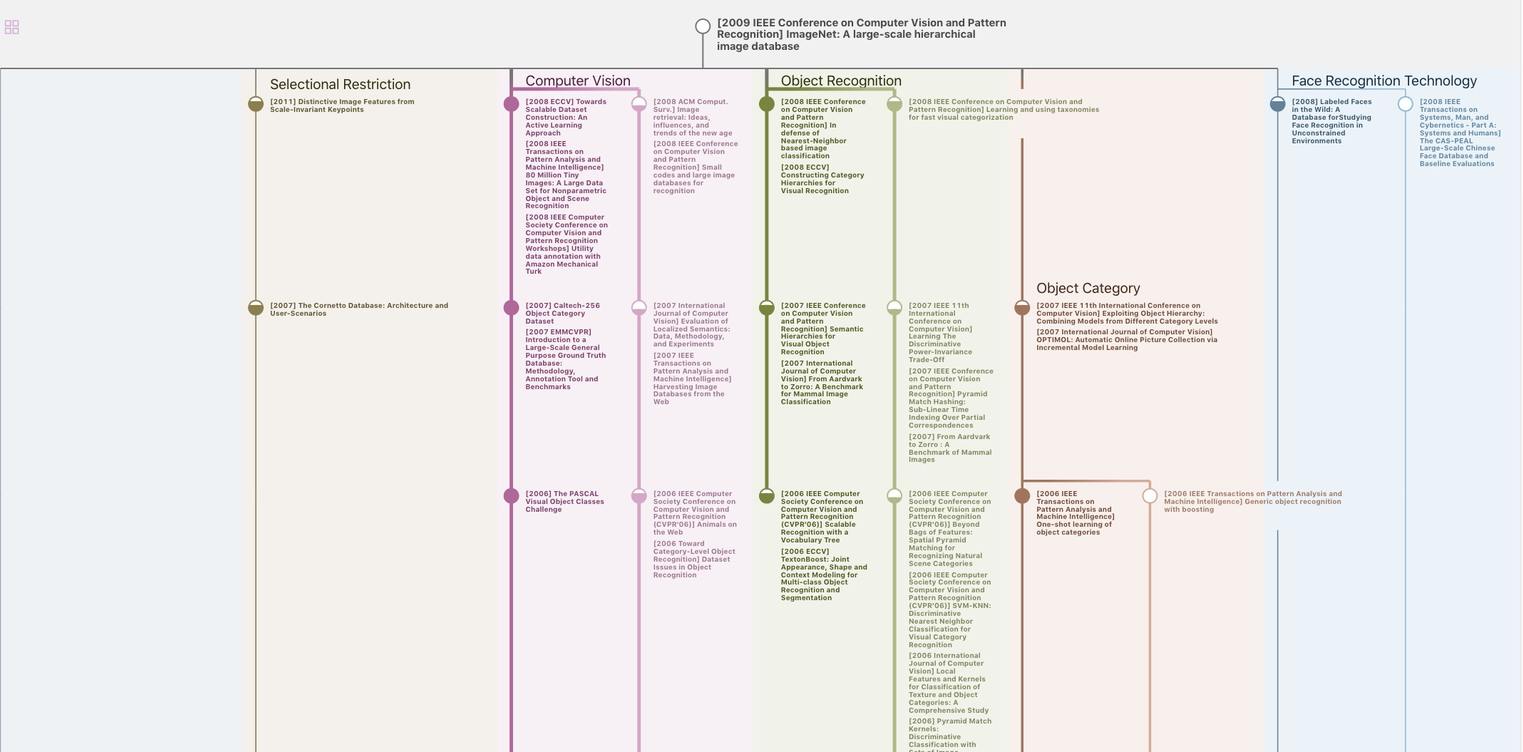
生成溯源树,研究论文发展脉络
Chat Paper
正在生成论文摘要