Visual Analysis of Volume Ensembles Based on Local Features
semanticscholar(2016)
摘要
Ensemble datasets describe a specific phenomenon (e.g., a simulation scenario or a measurements series) through a large set of individual ensemble members. These individual members typically do not differ too much from each other but rather feature slightly changing characteristics. In many cases, the ensemble members are defined in 3D space, which implies severe challenges when exploring the complete ensembles such as handling occlusions, focus and context or its sheer datasize. In this paper we address these challenges and put our focus on the exploration of local features in 3D volumetric ensemble datasets, not only by visualizing local characteristics, but also by identifying connections to other local features with similar characteristics in the data. We evaluate the variance in the dataset and use the the spatial median (medoid) of the ensemble to visualize the differences in the dataset. This medoid is subsequently used as a representative of the ensemble in 3D. The variance information is used to guide users during the exploration, as regions of high variance also indicate larger changes within the ensemble members. The local characteristics of the regions can be explored by using our proposed 3D probing widgets. These widgets consist of a 3D sphere, which can be positioned at any point in 3D space. While moving a widget, the local data characteristics at the corresponding position are shown in a separate detail view, which depicts the local outliers and their surfaces in comparison to the medoid surface. The 3D probing widgets can also be fixed at a user-defined position of interest. The fixed probing widgets are arranged in a similarity graph to indicate similar local data characteristics. The similarity graph thus allows to explore whether high variances in a certain region are caused by the same dataset members or not. Finally, it is also possible to compare a single member against the rest of the ensemble. We evaluate our technique through two demonstration cases using volumetric multi-label segmentation mask datasets, two from the industrial domain and two from the medical domain.
更多查看译文
AI 理解论文
溯源树
样例
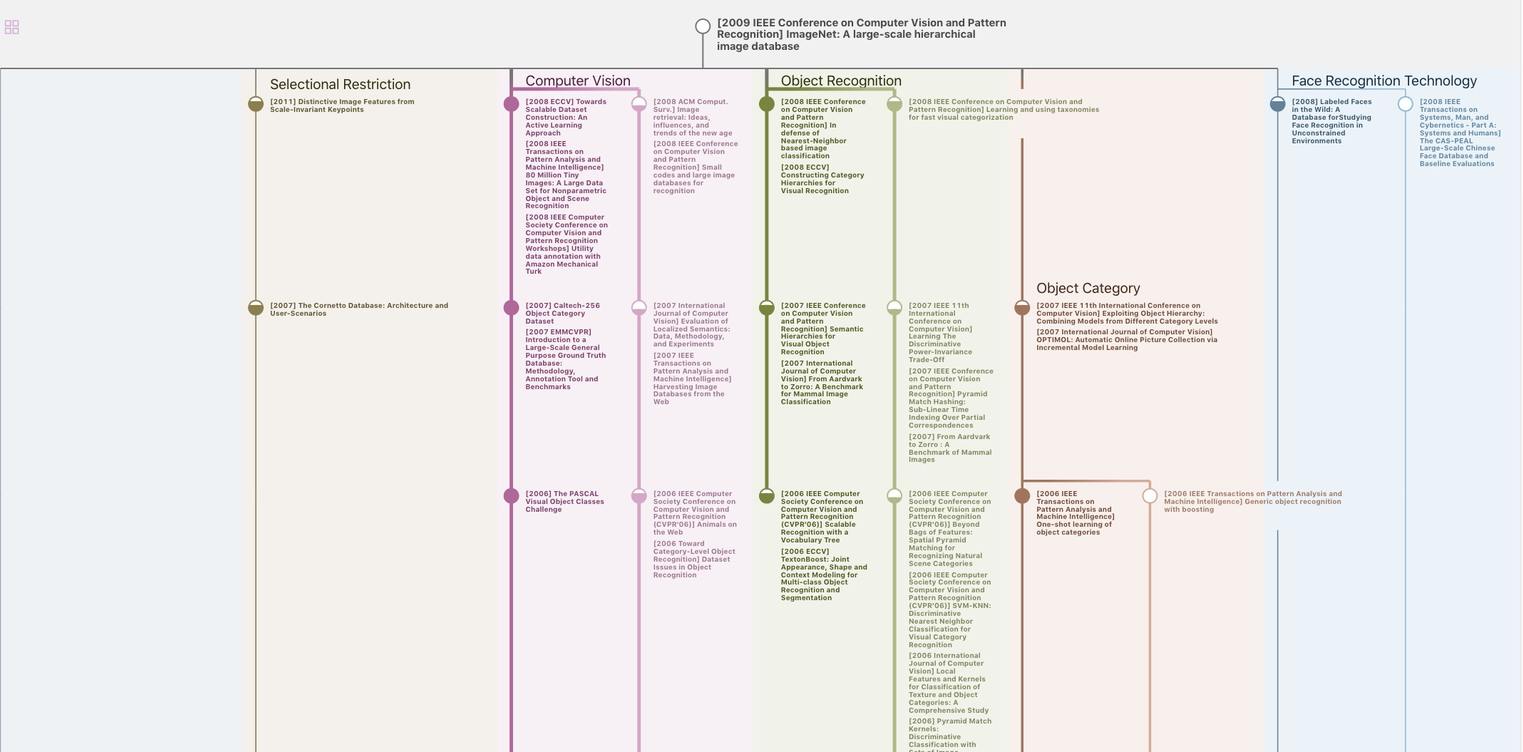
生成溯源树,研究论文发展脉络
Chat Paper
正在生成论文摘要