Deposited in DRO : 03 October 2018 Version of attached le : Accepted Version Peer-review status of attached
semanticscholar(2019)
摘要
Clinical data, such as evaluations, treatments, vital sign and lab test results, are usually observed and recorded at hospital systems. Making use of such data to help physicians evaluating the mortality risk of in-hospital patients provides an invaluable source of information which ultimately help improving the health-care services. Therefore, quick and accurate prediction of mortality can be critical for physicians to make intervention decisions. In this work, we introduce a predictive Deep Learning model aiming to evaluate the mortality risk of in-hospital patients. Stacked Desoising Autoencoder (SDA) is trained using a unique time-stamped dataset (King Abdullah International Research Center KAIMRC) which is naturally imbalanced. The work is compared to common deep learning approaches using different methods for data balancing. The proposed model demonstrated here to overcome the problem of imbalanced data and outperform common deep learning approaches with an accuracy of 77.13% for the Recall macro.
更多查看译文
AI 理解论文
溯源树
样例
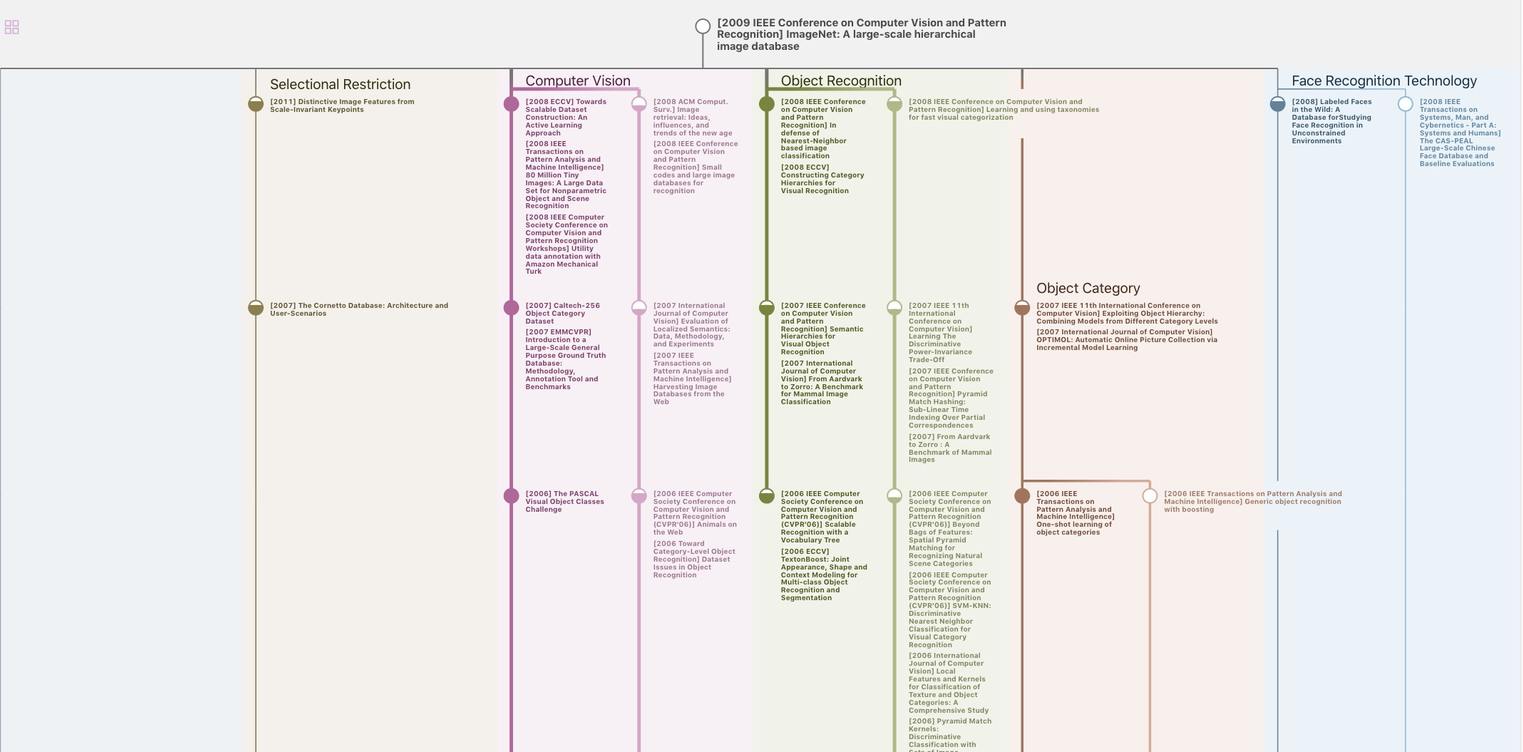
生成溯源树,研究论文发展脉络
Chat Paper
正在生成论文摘要