Bayesian Neural Networks for K-Shot Learning
semanticscholar(2017)
摘要
This thesis introduces multiple extensions and improvements to the probabilistic framework for k-shot image classification proposed by Bauer et al. [2017]. The aim of their framework is to transfer knowledge from a classifier trained on a large dataset to new classes with few training examples. We address three key considerations in this setting: the effectiveness of the weights-based concept transfer, the ability of the classifier to transform the feature space, and the influence of the featurebased representational transfer. More precisely, we propose a multitask training procedure for robust concept estimation, develop a probabilistic framework for nonlinear neural network classifiers, and analyse the impact of a bottleneck layer on the model performance respectively. The proposed extensions show improvement over the results from the original framework in terms of both effectiveness of the concept transfer and the final k-shot learning performance.
更多查看译文
AI 理解论文
溯源树
样例
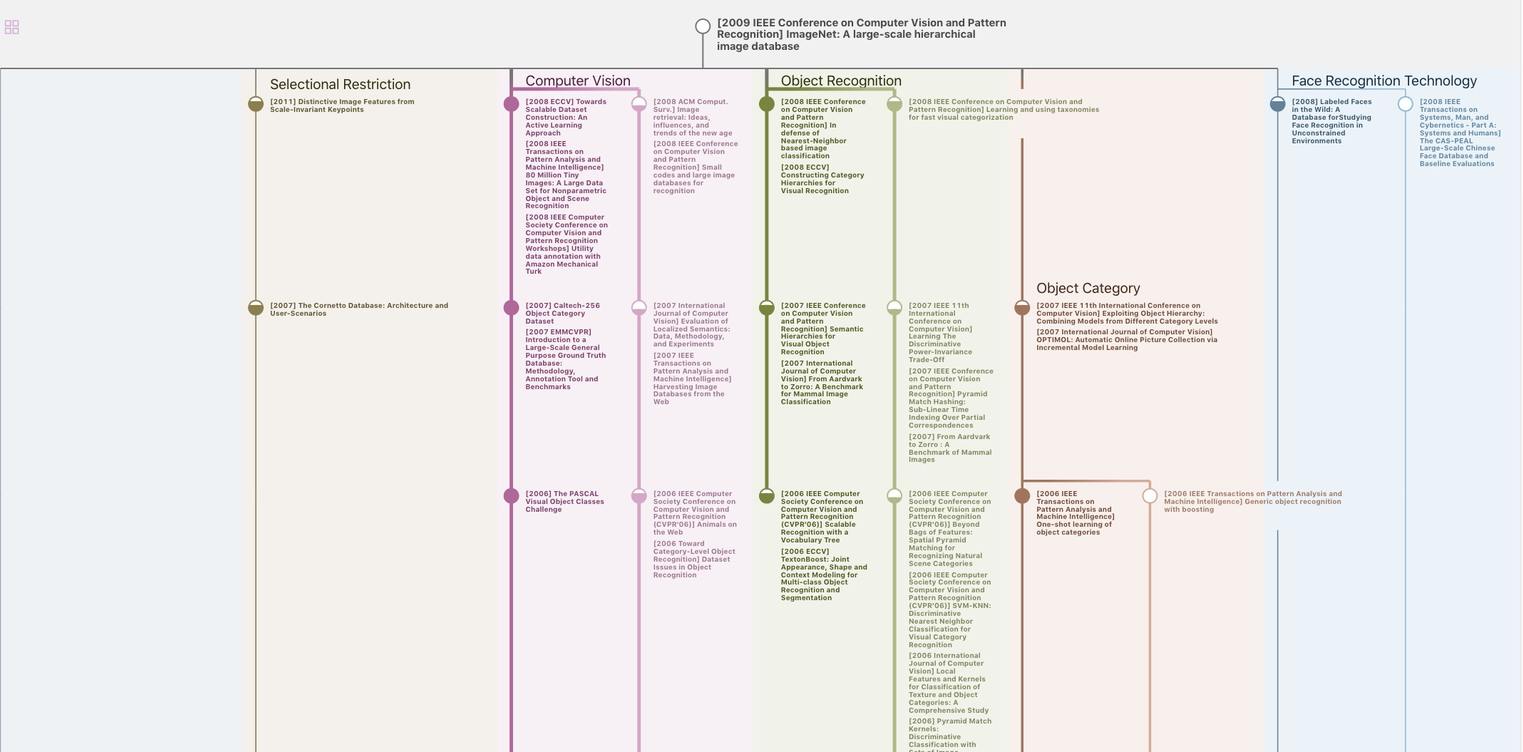
生成溯源树,研究论文发展脉络
Chat Paper
正在生成论文摘要