Evaluating urban sensing applications using actively and passively-generated mobile phone location data
semanticscholar(2015)
摘要
Mobile phone location data from telecom operators in the form of Call Detail Record (CDR) has been widely studied, especially to extract insights into urban dynamics [5]. Such massive data can be useful to extract patterns of human mobility at an incredible scale. This data allows sampling the location of a mobile device every time the device is actively interacting with the network, e.g. at call time, while sending and SMS, or while connecting to the Internet with Smartphones. The disadvantage of such data collection method is that the spatio-temporal sampling of each individual user trajectory over time might be very uneven, and perhaps biased to specific locations (e.g. home locations) or times (e.g. during the evenings). Moreover, some users might interact more or less with the network, resulting in more or less mobility information extracted from them. This could result in under-sampling the population, or more problematically, biasing the extracted insights. In the past decade, there has been a rising interest in using mobile phone location data to infer user trajectories [1], [8], and so study human mobility and their patterns [7]. Different types of data have been used in these studies. CDR data were used in [7],[2],[6]. CDR information, enriched with records from Internet access was exploited in [4]. Data from idle phones were also used in [9] to estimate the road traffic. However, to the best of our knowledge, no work so far has specifically compared the different types of datasets that a telecom operator can collect. Moreover, no work so far has analysed the limitations of using a specific dataset for a given urban sensing application. We then ask the question whether insights extracted from actively collected-mobile phone location data are a good proxy for human mobility. To answer this question, we compare such results, with results extracted by both actively and passively sampling user location, which constitute a richer set of location information. We used a real dataset collected from a telecom operator in Belgium, which had a system which allowed to collect both CDR, records of Internet connections (which we call IPDR), and passively generated data (which we call Signaling), generated because of location updates, radio access network or data sessions. We were able to decompose the dataset in three different ones: only CDR, CDR + IPDR, and all data, since each location event was tagged with the specific type of event generating it. The dataset contains anonymised mobile phone location data from Mobistar, for users in the area around the city of Mons, Belgium. The data covers users connected to 150 distinct cell towers in the city area. For each cell tower, we were given the coordinate and the azimuth of each cell sector. Thus we were able to derive a voronoi tessellation of the space, following the approach presented in [3]. Some cells cover the same area (i.e. 2G and 3G antennas installed on the same tower), resulting in being able to discriminate among 58 distinct locations in the city. The available data covers one week in October 2014. We use the available data to simulate 3 different scenarios: • availability of only CDR information, in which we only use the CALL and SMS data items, and are representing cases in which only CDR information is provided. • availability of CDR and IPDR, in which we use the above data, together with IPDR, to represent cases where all Event-driven signaling information is provided. • availability of all signaling information (CRD+IPDR+Signaling), which will be our reference for comparison. Figure 1 depicts an example of temporal sequence of events for a user in the dataset. We also depict the trajectory that we are able to detect, given the tree different scenarios. The example clearly shows that for this user, the availability of all information allows detecting 3 different visited locations, and an estimated stop time for locations 2 and 3. If no Signaling information is available, only two visited places could be detected, and the estimated stop time would also be reduced, with lowest accuracy in the case of only CDR information available. At the general level, the advantages of using Networkdriven location data (in addition to event-driven) include: • sampling more users (people who are not making calls/SMS/Internet connections); • having more samples of user locations, particularly at times where users are not too active, e.g. at night); Motivated by this example, in the following sections we quantitatively and qualitative analyse the difference of the three datasets from the point of view of extracting accurate trajectories. This is firstly done by extracting applicationindependent characteristics. Then, we selected frequently used urban sensing applications designed for Telco data, and compared the accuracy of the extracted insights among the different datasets, highlighting in which cases one dataset is preferable compared to the others. I. APPLICATION-INDEPENDENT COMPARISON We can compare the three datasets along different dimensions. We started by looking at the classes of users for which we have the different events, and try to characterise them in terms of amount of events we are collecting. A first comparison Fig. 1. Example of temporal sequence of events (top), and estimated trajectories using three different datasets (bottom). To simplify the reading, the locations have been drawn in one dimension (as opposed to the two dimensions (latitude and longitude). between the three datasets is in terms of the set of users for which we have data. We analysed one week in October counting, for each user, the number of the three different types of events (CDR, IPDR and Signaling). Figure 2 shows a Venn diagram of the unique users by data type. Only for about 11% of the users we can see all three different types of events. This is due to that fact that not all users have smartphones for which IPDR can be generated. Moreover, some users are only seen very temporary in the dataset (users only traversing the city), and so only Signaling information is available. Not all users generate the same number of events. The number of events by user follows a long tail distribution. Clearly considering only CDR or CDR+IPDR events, the average number of events per user is smaller. We then ask the question whether this decrease in the number of events is concentrated in particular hours of the day, or is equally spread over time. Figure 3(a) show the distribution of users by number of distinct hours for which there is at least one event. Curves CDR and CDR+IPDR look very close, to show that the large amount of IPDR events are on average concentrated in the same hours as the CDR events. Signaling events are able to sample the user location over many more hours. However, if we only consider daily hours (from 6 to 22), as reported in Figure 3(b) we notice that difference decreases. This let is hypothesise that actively generated location data are a good sample of user location during daylight hours. We will verify this intuition in the next section. Fig. 2. Percentage of users per data type and relative intersections. (a) all hours (b) daylight hours Fig. 3. Distribution of user by number of hours for which at least one event II. APPLICATION-DEPENDENT COMPARISON We have taken a frequently used example of urban sensing application using mobile phone location data: the count estimation over time, such as the number of people being in a certain cell in a given time interval. This information is highly relevant for many sectors, such as Retail, Property, Leisure and Media, since it allows to compare locations in terms of expected crowd. Clearly, an accurate estimation of the time series of number of users by location is crucial to provide trustable insights. We computed user count time series for each location in the city, starting from the three different datasets. Cumulative count estimation by location is show in Figure 4(a), ranked by increasing value of count (based on the reference dataset). We computed two measures of error. The first measure is the root mean square error computed on the hourly estimates compared to the counts estimated using all data (CDR+IPDR+Signaling). The error ranges from 0 to 1, and low values correspond to low error. Moreover, we computed a measure of Normalised discounted cumulative gain (nDCG) [10] used in recommender systems to measure rank quality. We have chosen this measure to evaluate whether the estimated ranking of crowded locations is kept the same by using CDR or CDR+IPDR only information. The error ranges from 0 to 1, and high values correspond to low error. This measure is different from the RMSE, since it does not take into account the absolute estimate count for each location or (a) by antenna (b) error by day of week Fig. 4. Density estimation
更多查看译文
AI 理解论文
溯源树
样例
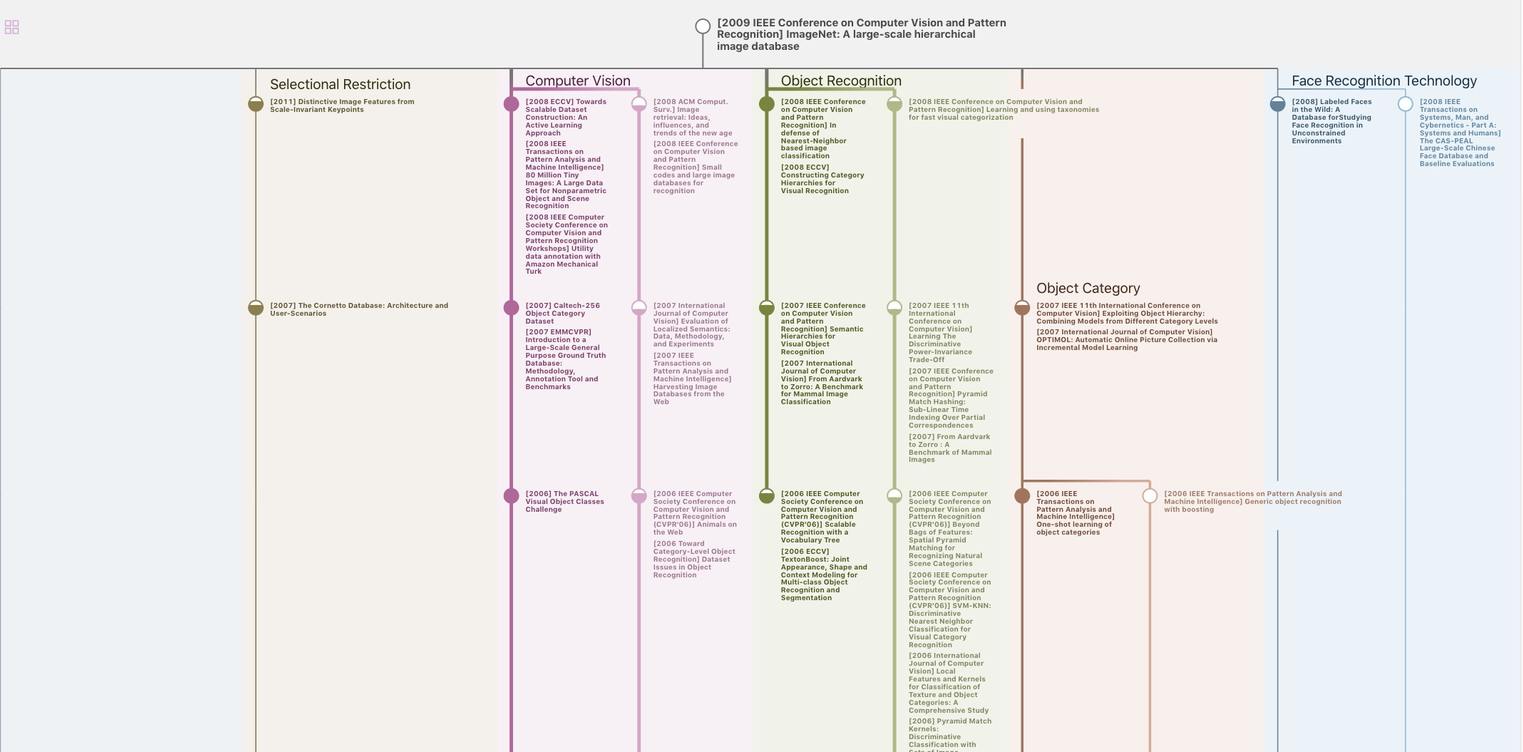
生成溯源树,研究论文发展脉络
Chat Paper
正在生成论文摘要