Supplementary Toyota Smarthome : Real-World Activities of Daily Living
semanticscholar(2019)
摘要
In this supplementary material, we provide more details on the Toyota Smarthome dataset (hereafter Smarthome), the evaluation protocols and the state-of-the-art methods used for comparison in the main body of the paper. In fig. 1, we present an example frame for each of the 31 activities in Smarthome. The figure shows the rich diversity of activity classes in the dataset: some activities are a composition of sub-activities (e.g., cooking is composed of cleaning dishes, cleaning up, cutting, stirring and using stove), some activities correspond to the same activity, but performed using different objects (e.g., drinking from a cup, can, or bottle), other activities are almost completely static (e.g., reading book, using phone or watching TV). To learn more about Toyota Smarthome dataset please visit the project website1. To date, there are more than 50 human activity recognition datasets. Although each one of them has unique, beneficial characteristics for the evaluation of activity recognition algorithms, they have also limitations. Table 1 lists the most popular public RGB+D ADL datasets to our knowledge with their key features. All the datasets mentioned in table 1 are captured indoors using Kinect sensors and thus provides either skeleton info or depth map in addition to RGB cue. In terms of dataset size (i.e., number of video samples and activity classes), Smarthome is the second largest dataset with 16,115 clips. For evaluation of activity recognition algorithms on Smarthome, we defined a cross-subject (CS) and two crossview (CV1 & CV2) protocols. Fig. 2, 3 and 4 depict training and testing sample distributions of activities for the different evaluation protocols. Fig. 2 shows how the activity categories are imbalanced throughout the dataset and in our evaluation protocol. For example, the number of training samples for the activities walking, drinking from glass, reading book are considerably higher than with other classes. This feature is another key difference between
更多查看译文
AI 理解论文
溯源树
样例
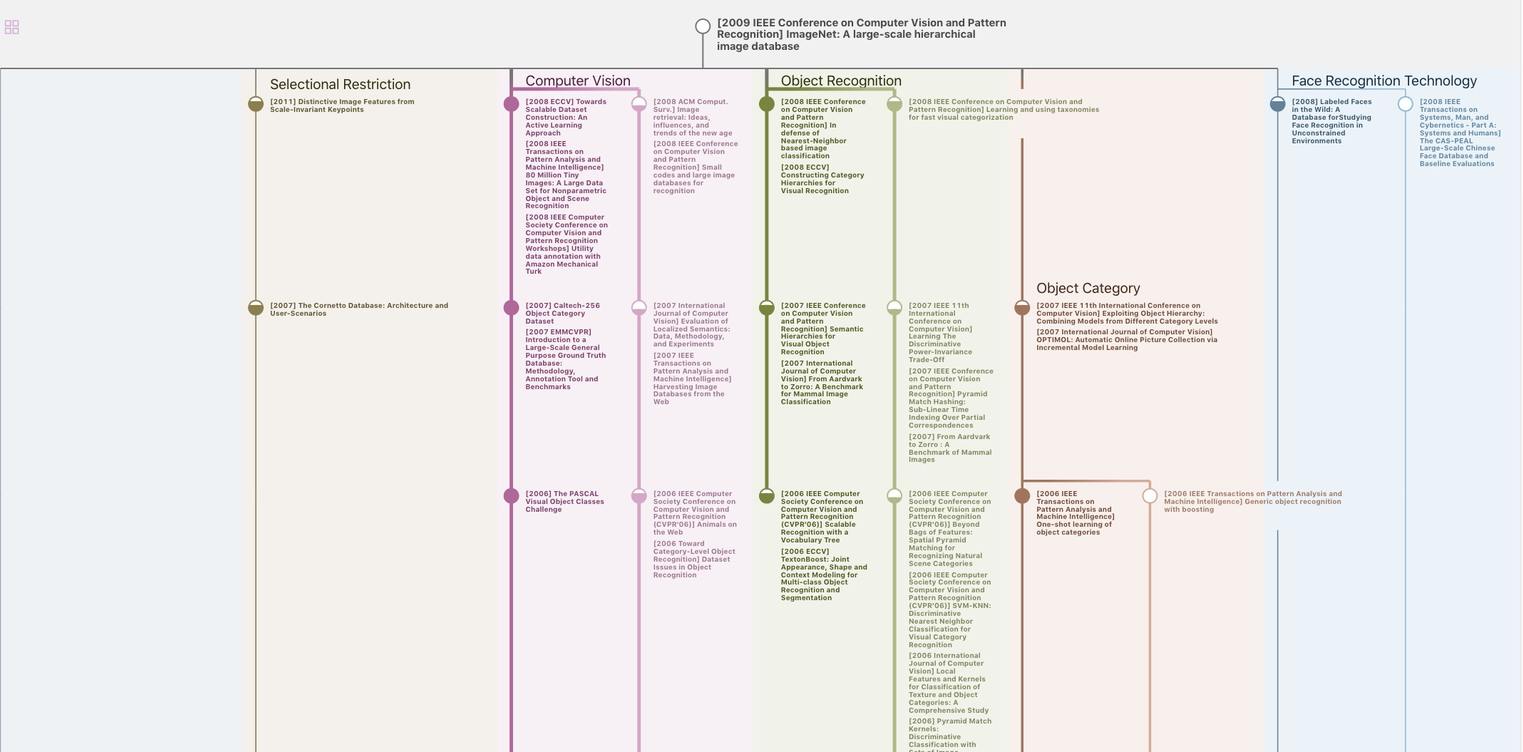
生成溯源树,研究论文发展脉络
Chat Paper
正在生成论文摘要