On generating network tra ic datasets with synthetic a acks for intrusion detection
semanticscholar(2019)
摘要
Most research in the area of intrusion detection requires datasets to develop, evaluate or compare systems in one way or another. In this eld, however, nding suitable datasets is a challenge on to itself. Most publicly available datasets have negative qualities that limit their usefulness. In this article, we propose ID2T (Intrusion Detection Dataset Toolkit) to tackle this problem. ID2T facilitates the creation of labeled datasets by injecting synthetic aacks into background trac. e injected synthetic aacks blend themselves with the background trac by mimicking the background trac’s properties to eliminate any trace of ID2T’s usage. is work has three core contribution areas. First, we present a comprehensive survey on intrusion detection datasets. In the survey, we propose a classication to group the negative qualities we found in the datasets. Second, the architecture of ID2T is revised, improved and expanded. e architectural changes enable ID2T to inject recent and advanced aacks such as the widespread EternalBlue exploit or botnet communication paerns. e toolkit’s new functionality provides a set of tests, known as TIDED (Testing Intrusion Detection Datasets), that help identify potential defects in the background trac into which aacks are injected. ird, we illustrate how ID2T is used in dierent use-case scenarios to evaluate the performance of anomaly and signature-based intrusion detection systems in a reproducible manner. ID2T is open source soware and is made available to the community to expand its arsenal of aacks and capabilities.
更多查看译文
AI 理解论文
溯源树
样例
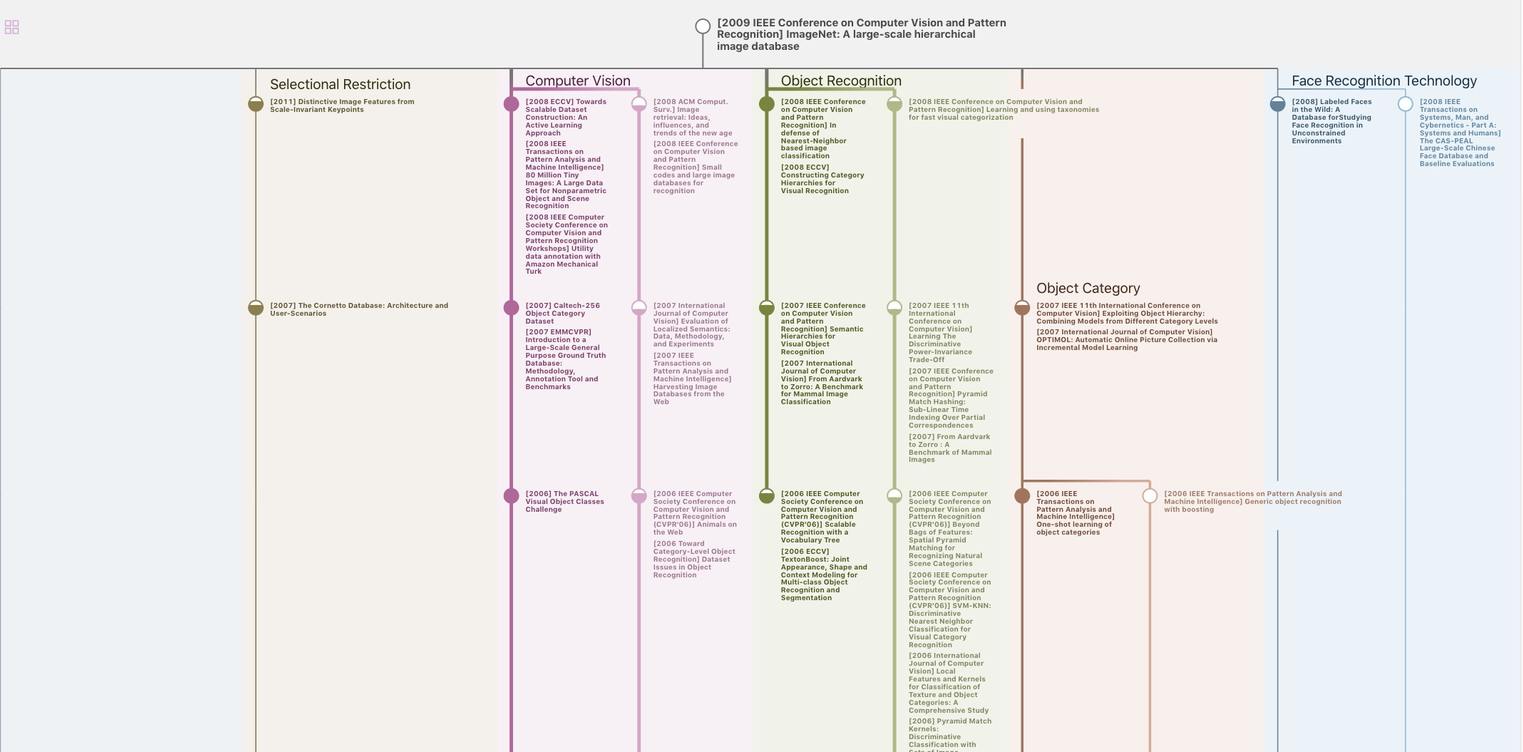
生成溯源树,研究论文发展脉络
Chat Paper
正在生成论文摘要