Automatic Detection of Angiectasia : Evaluation of Deep Learning and Handcrafted Approaches ∗
semanticscholar(2018)
摘要
Angiectasia, formerly called angiodysplasia, is one of the most frequent vascular lesions and often the cause of gastrointestinal bleedings. Medical specialists assessing videos of examinations reach a detection performance of 16% for the detection of bleeding to 69% for the detection of angiectasia. In this paper, we present several machine-learning-based approaches for angiectasia detection in wireless video capsule endoscopy images. The most promising results for pixel-wise localization and framewise detection are obtained by the proposed deep learning approach using generative adversarial networks (GANs) with a sensitivity of 88% and specificity of 99.9% for pixel-wise localization and a sensitivity of 98% and a specificity of 100% for frame-wise detection, which fits the requirements for automatic angiectasia detection in real clinical settings. Superficial vascular lesion called angiectasia represent one of the most common source of bleeding in the small bowel and are therefore important to be detected. The most common procedure to detect angiectasia is video capsule endoscopy (VCE). State-of-the-art software from the industry is able to reach an automatic detection sensitivity of 41% and a specificity of 67%. For a clinical scenario, both, sensitivity and specificity should be as close as possible to 100%, but at least larger than 85% [1]. Automatic detection of angiectasia is not very well researched, and there are only a few publications on the topic using saliency detection [2]. Therefore, in this work, we test different machine learning approaches to tackle automatic angiectasia detection in VCE videos. Pixel-wise segmentation/localization approaches. The presented localization approach is able to pixel accurate mark the angiectasia in the given frame based on a GAN architecture initially developed for the retinal vessel segmentation in fundoscopic images called V-GAN. We added an additional output layer to the generator network that implements an activation layer with step function in order to generate the binary segmentation output. Frame-wise detection approaches. These approaches
更多查看译文
AI 理解论文
溯源树
样例
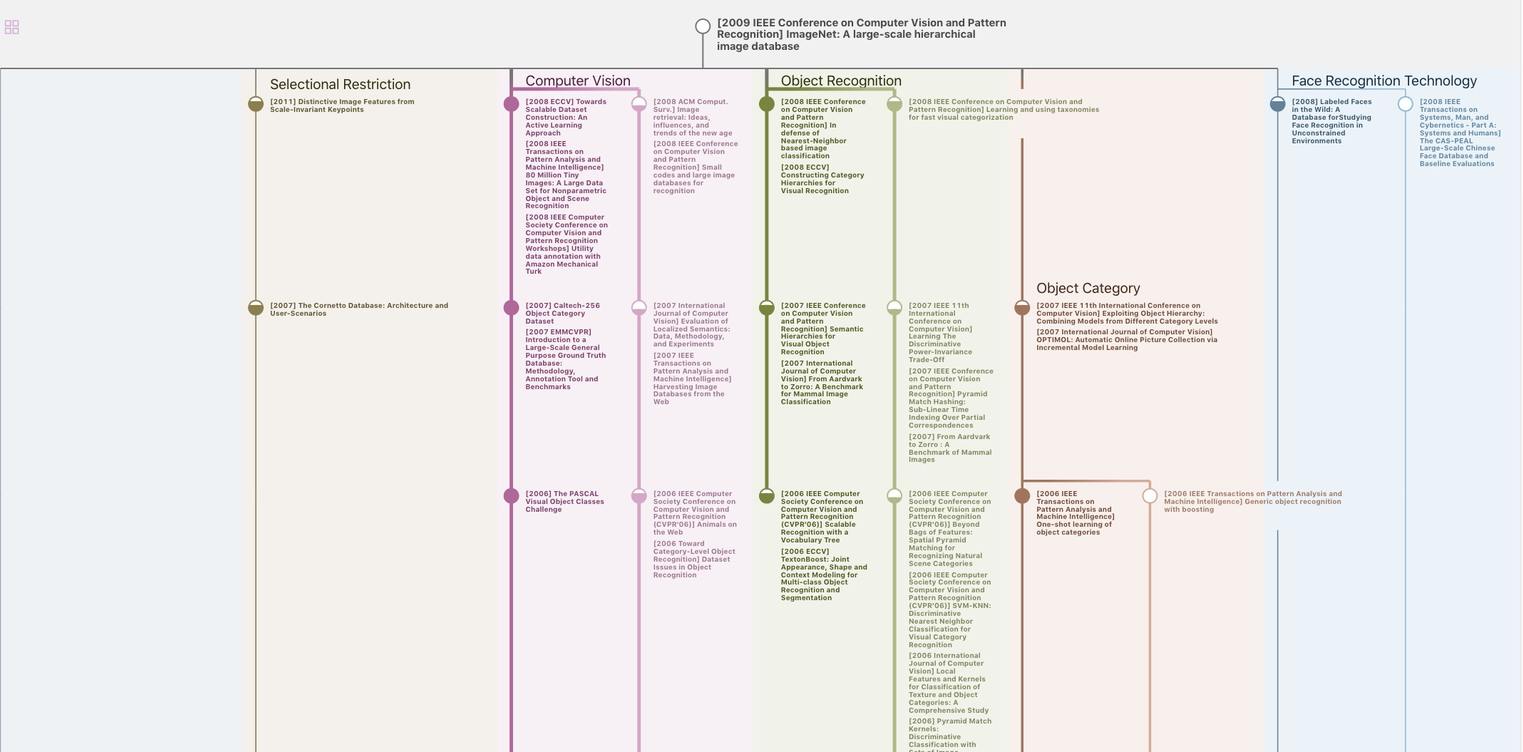
生成溯源树,研究论文发展脉络
Chat Paper
正在生成论文摘要