Challenge ICLR 2019 REPRODUCIBILITY-CHALLENGE DISCRIMINATOR-ACTOR-CRITIC : ADDRESSING SAMPLE INEFFICIENCY AND REWARD BIAS IN ADVERSARIAL IMITATION LEARNING
semanticscholar(2018)
摘要
As part of the ICLR 2019 Reproducibility Challenge we attempted to replicate the results of Discriminator-Actor-Critic: Addressing Sample Inefficiency and Reward Bias in Inverse Reinforcement Learning (Anonymous, 2019). Discriminator-Actor-Critic (DAC) is an adversarial imitation learning algorithm. It uses an off-policy reinforcement learning algorithm to improve upon the sample efficiency of existing methods, and it extends the learning environment with absorbing states and uses a new reward function in order to achieve unbiased rewards. We were able to reproduce some but not all of the claimed results, achieving comparable rewards and sample efficiency on two of four environments. All of our code is available at: https://github.com/vluzko/dac-iclr-reproducibility
更多查看译文
AI 理解论文
溯源树
样例
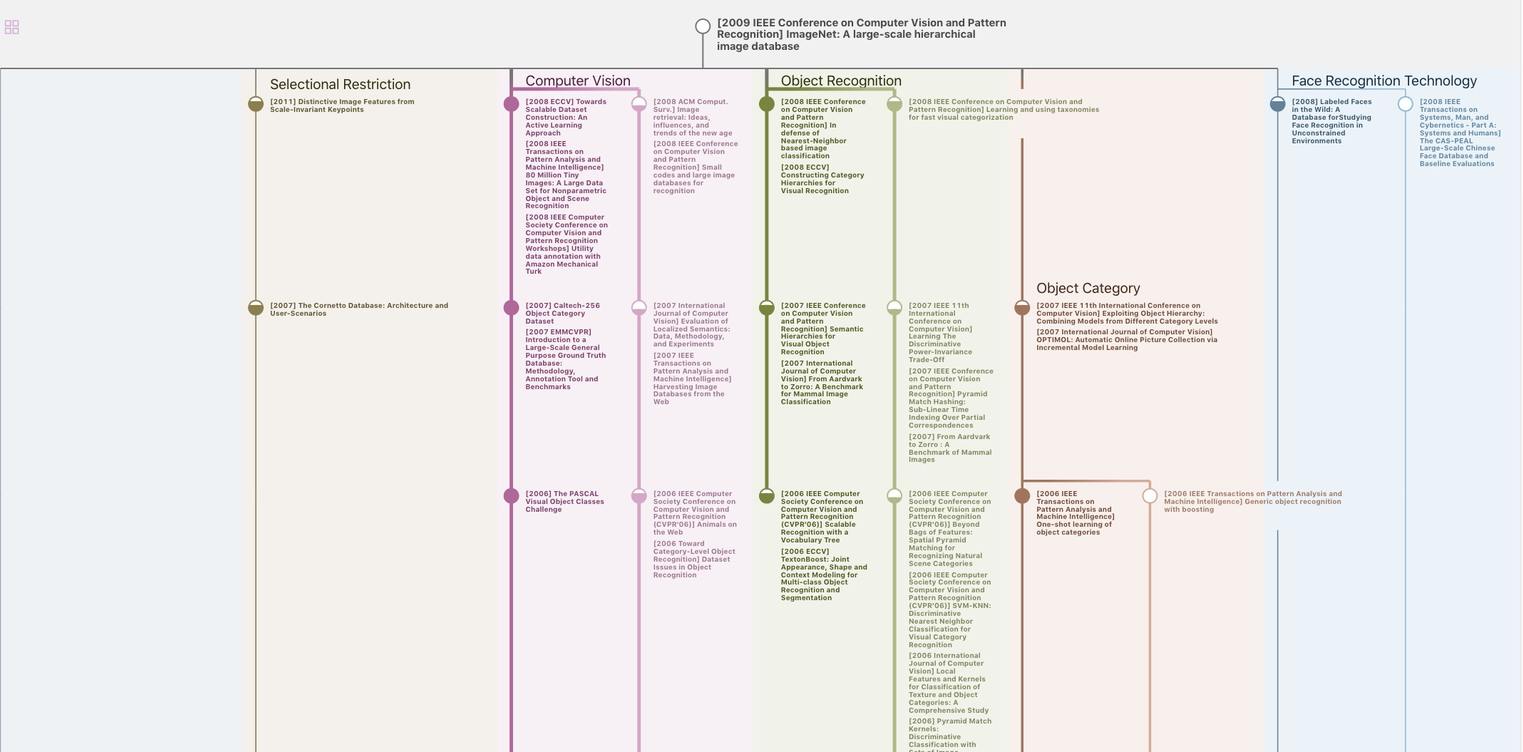
生成溯源树,研究论文发展脉络
Chat Paper
正在生成论文摘要