D S ] 1 2 Ju l 2 01 9 Online-Learning for min-max discrete problems
Theoretical Computer Science(2019)
摘要
We study various discrete nonlinear combinatorial optimization problems in an online learning framework. In the first part, we address the question of whether there are negative results showing that getting a vanishing (or even vanishing approximate) regret is computational hard. We provide a general reduction showing that many (min-max) polynomial time solvable problems not only do not have a vanishing regret, but also no vanishing approximation α-regret, for some α (unless NP = BPP ). Then, we focus on a particular min-max problem, the min-max version of the vertex cover problem which is solvable in polynomial time in the offline case. The previous reduction proves that there is no (2 − ǫ)-regret online algorithm, unless Unique Game is in BPP ; we prove a matching upper bound providing an online algorithm based on the online gradient descent method. Then, we turn our attention to online learning algorithms that are based on an offline optimization oracle that, given a set of instances of the problem, is able to compute the optimum static solution. We show that for different nonlinear discrete optimization problems, it is strongly NP -hard to solve the offline optimization oracle, even for problems that can be solved in polynomial time in the static case (e.g. min-max vertex cover, min-max perfect matching, etc.). On the positive side, we present an online algorithm with vanishing regret that is based on the follow the perturbed leader algorithm for a generalized knapsack problem. Keywords— Online learning, min-max problems, hardness, follow the leader, online gradient descent
更多查看译文
AI 理解论文
溯源树
样例
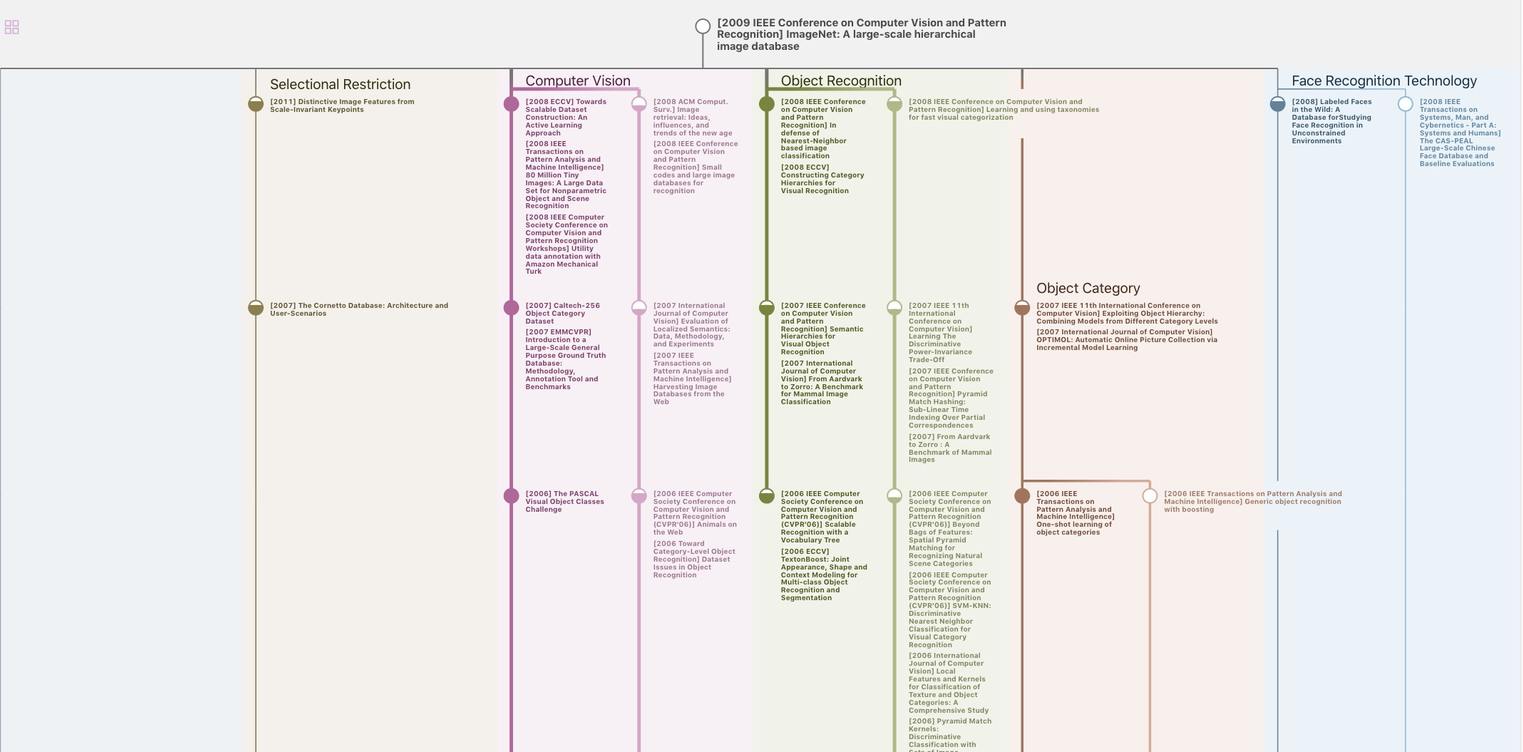
生成溯源树,研究论文发展脉络
Chat Paper
正在生成论文摘要