Learning hidden Markov models for linear Gaussian systems with applications to event-based state estimation
Automatica(2021)
摘要
This work attempts to approximate a linear Gaussian system with a finite-state hidden Markov model (HMM), which is found useful in dealing with challenges in designing networked control systems An indirect approach is developed, where a state-space model (SSM) is firstly identified for a Gaussian system and the SSM is then used as an emulator for learning an HMM. In the proposed method, the training data for the HMM are obtained from the data generated by the SSM through building a quantization mapping. Parameter learning algorithms are designed to learn the parameters of the HMM, through exploiting the periodical structural characteristics of the HMM. The convergence and asymptotic properties of the proposed algorithms are analyzed. The HMM learned using the proposed algorithms is applied to event-triggered state estimation, and numerical results on model learning and state estimation demonstrate the validity of the proposed algorithms.
更多查看译文
关键词
Event-based state estimation,Linear Gaussian system,Hidden Markov models,Parameter learning
AI 理解论文
溯源树
样例
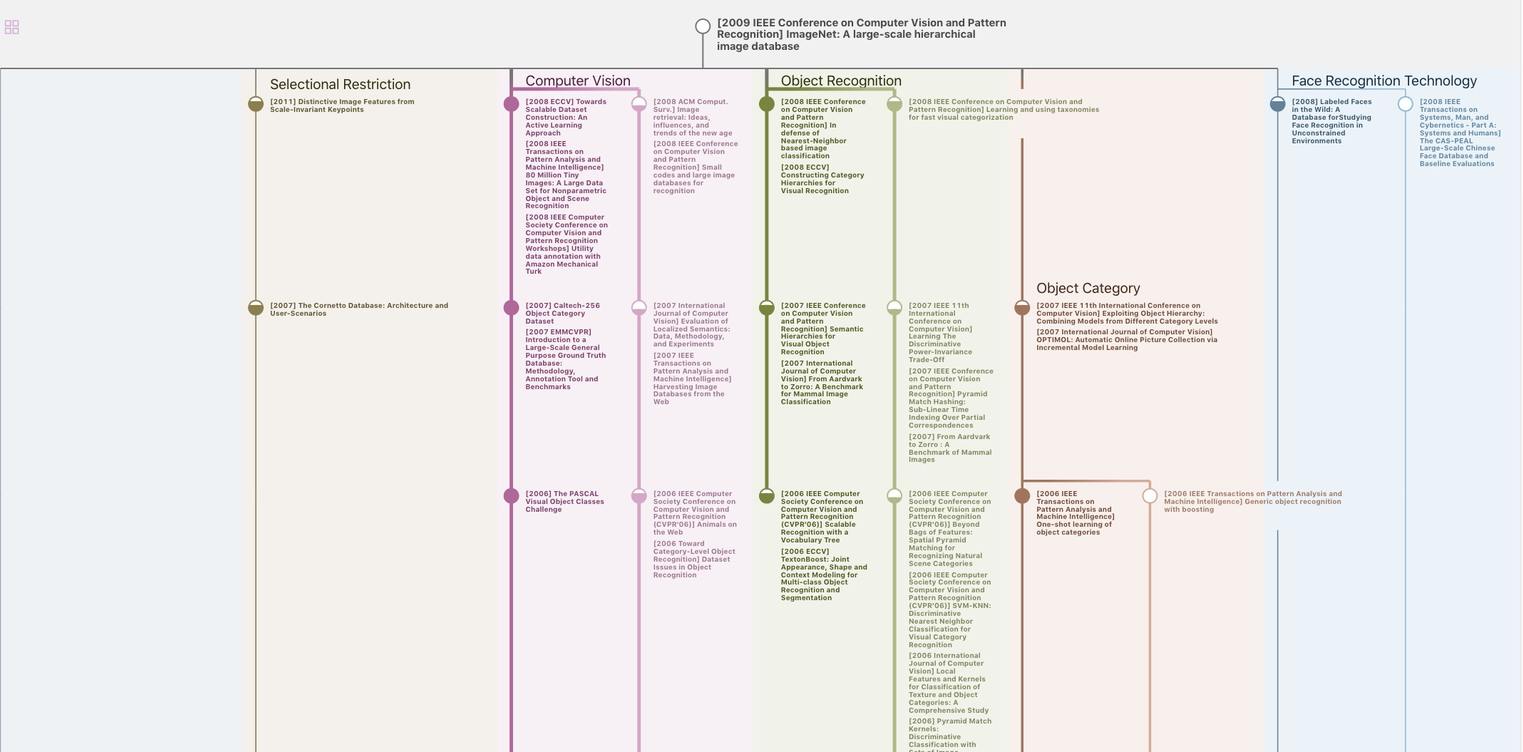
生成溯源树,研究论文发展脉络
Chat Paper
正在生成论文摘要