Structure motif centric learning framework for inorganic crystalline systems
arxiv(2020)
摘要
Incorporation of physical principles in a network-based machine learning (ML) architecture is a fundamental step toward the continued development of artificial intelligence for materials science and condensed matter physics. In this work, as inspired by the Pauling rule, we propose that structure motifs (polyhedral formed by cations and surrounding anions) in inorganic crystals can serve as a central input to a machine learning framework for crystalline inorganic materials. Taking metal oxides as examples, we demonstrated that, an unsupervised learning algorithm Motif2Vec is able to convert the presence of structure motifs and their connections in a large set of crystalline compounds into unique vector representations. The connections among complex materials can be largely determined by the presence of different structure motifs and their clustering information are identified by our Motif2Vec algorithm. To demonstrate the novel use of structure motif information, we show that a motif-centric learning framework can be effectively created by combining motif information with the recently developed atom-based graph neural networks to form an atom-motif dual graph network (AMDNet). Taking advantage of node and edge information on both atomic and motif level, the AMDNet is more accurate than an atom graph network in predicting electronic structure related material properties of metal oxides such as band gaps. The work illustrates the route toward fundamental design of graph neural network learning architecture for complex material properties by incorporating beyond-atom physical principles.
更多查看译文
AI 理解论文
溯源树
样例
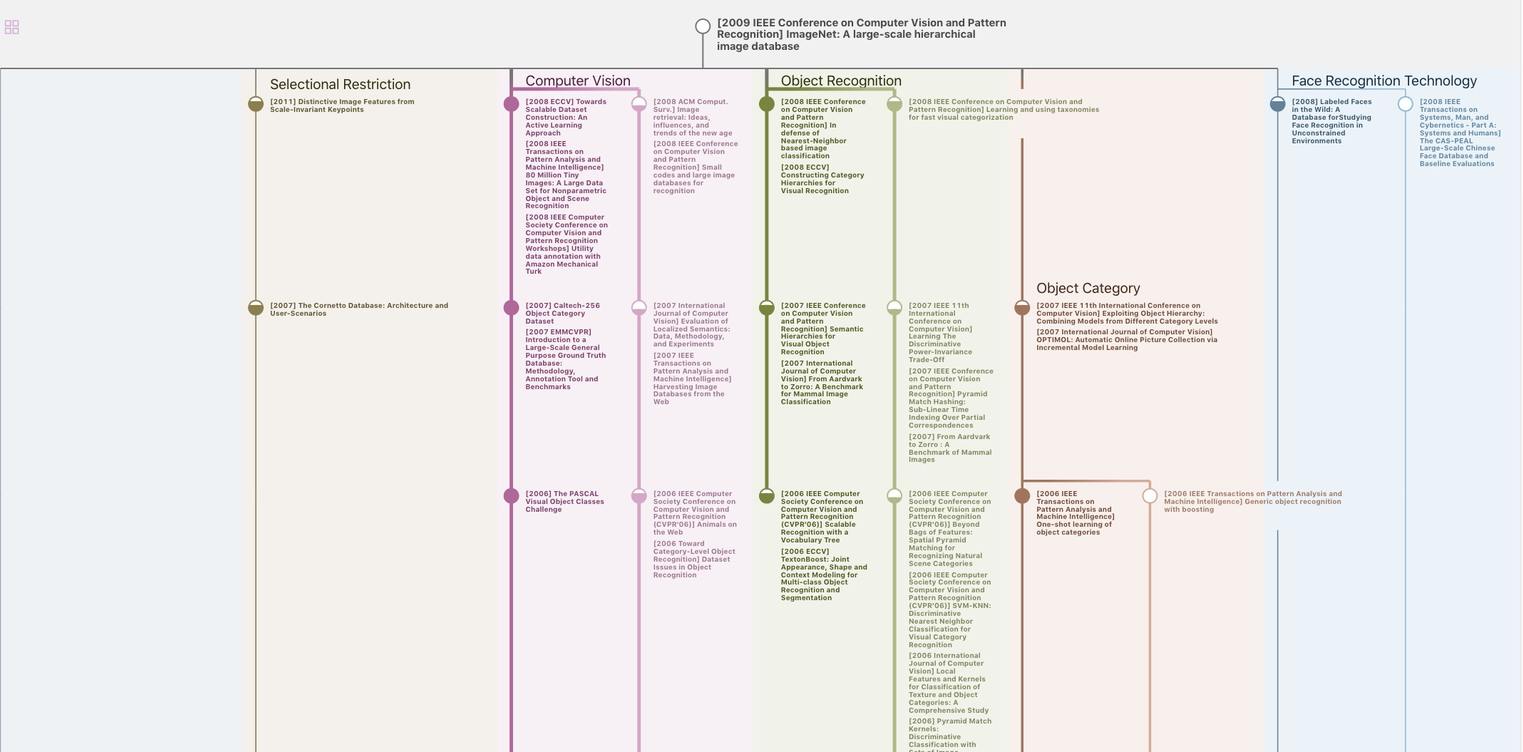
生成溯源树,研究论文发展脉络
Chat Paper
正在生成论文摘要