VPN: Learning Video-Pose Embedding for Activities of Daily Living
European Conference on Computer Vision(2020)
摘要
In this paper, we focus on the spatio-temporal aspect of recognizing Activities of Daily Living (ADL). ADL have two specific properties (i) subtle spatio-temporal patterns and (ii) similar visual patterns varying with time. Therefore, ADL may look very similar and often necessitate to look at their fine-grained details to distinguish them. Because the recent spatio-temporal 3D ConvNets are too rigid to capture the subtle visual patterns across an action, we propose a novel Video-Pose Network: VPN. The 2 key components of this VPN are a spatial embedding and an attention network. The spatial embedding projects the 3D poses and RGB cues in a common semantic space. This enables the action recognition framework to learn better spatio-temporal features exploiting both modalities. In order to discriminate similar actions, the attention network provides two functionalities - (i) an end-to-end learnable pose backbone exploiting the topology of human body, and (ii) a coupler to provide joint spatio-temporal attention weights across a video. Experiments (Code/models: https://github.com/srijandas07/VPN) show that VPN outperforms the state-of-the-art results for action classification on a large scale human activity dataset: NTU-RGB+D 120, its subset NTU-RGB+D 60, a real-world challenging human activity dataset: Toyota Smarthome and a small scale human-object interaction dataset Northwestern UCLA.
更多查看译文
关键词
Action recognition,Video,Pose,Embedding,Attention
AI 理解论文
溯源树
样例
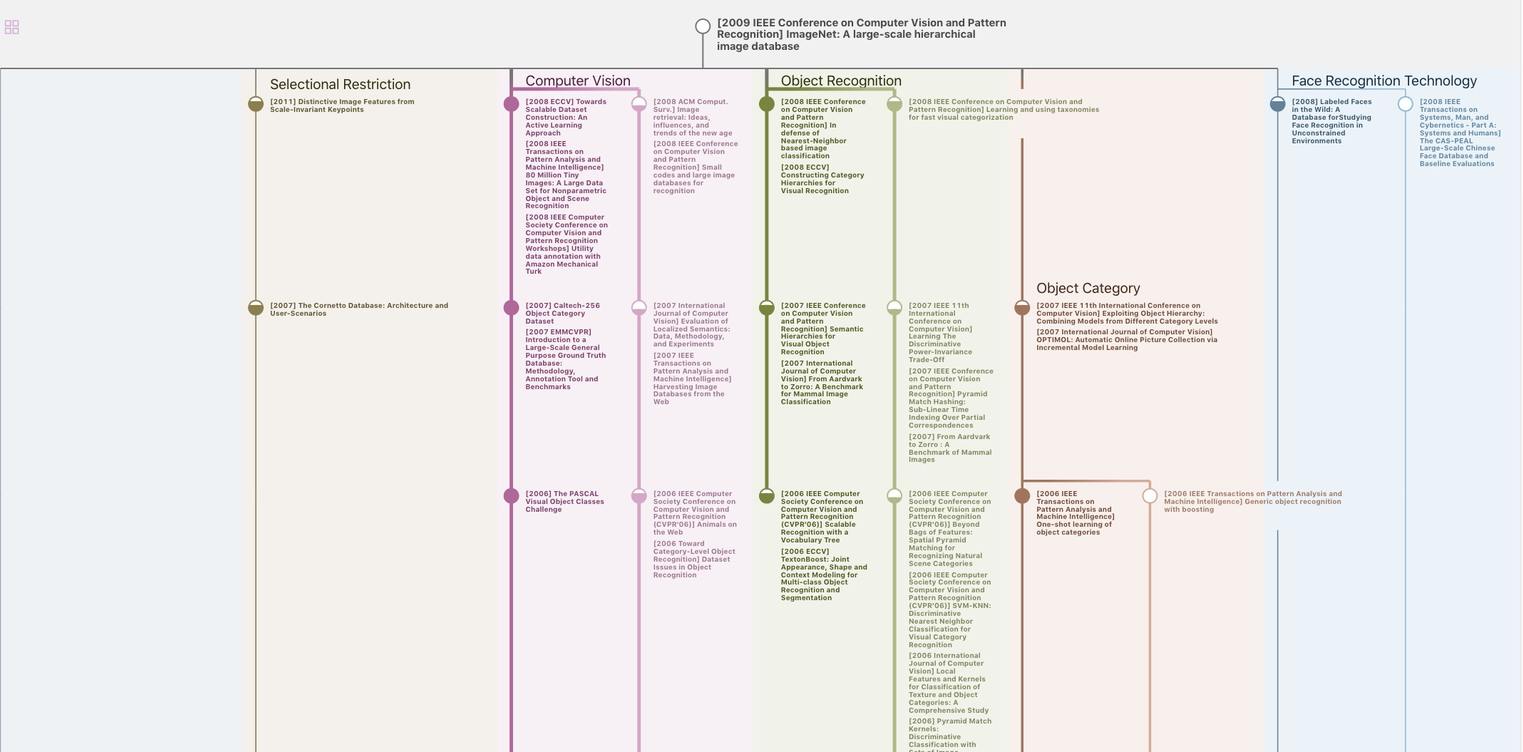
生成溯源树,研究论文发展脉络
Chat Paper
正在生成论文摘要