Novel Min-Max Reformulations of Linear Inverse Problems
JOURNAL OF MACHINE LEARNING RESEARCH(2022)
摘要
In this article, we dwell into the class of so-called ill-posed Linear Inverse Problems (LIP) which simply refer to the task of recovering the entire signal from its relatively few random linear measurements. Such problems arise in a variety of settings with applications ranging from medical image processing, recommender systems, etc. We propose a slightly generalized version of the error constrained linear inverse problem and obtain a novel and equivalent convex-concave min-max reformulation by providing an exposition to its convex geometry. Saddle points of the min-max problem are completely characterized in terms of a solution to the LIP, and vice versa. Applying simple saddle point seeking ascend-descent type algorithms to solve the min-max problems provides novel and simple algorithms to find a solution to the LIP. Moreover, the reformulation of an LIP as the min-max problem provided in this article is crucial in developing methods to solve the dictionary learning problem with almost sure recovery constraints.
更多查看译文
关键词
linear inverse problems, min-max problems, dictionary learning
AI 理解论文
溯源树
样例
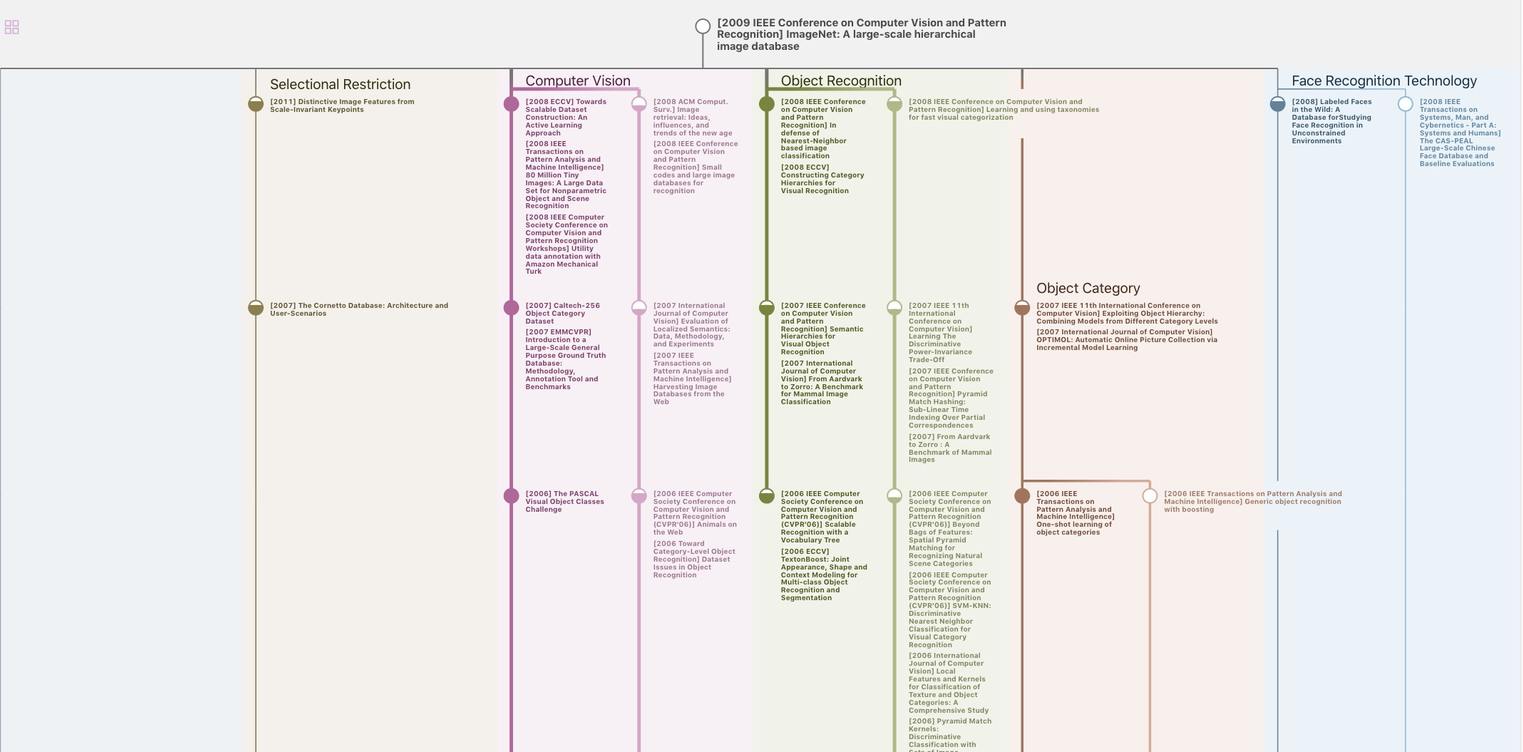
生成溯源树,研究论文发展脉络
Chat Paper
正在生成论文摘要