Two Sides of the Same Coin: White-box and Black-box Attacks for Transfer Learning
KDD '20: The 26th ACM SIGKDD Conference on Knowledge Discovery and Data Mining Virtual Event CA USA July, 2020(2020)
摘要
Transfer learning has become a common practice for training deep learning models with limited labeled data in a target domain. On the other hand, deep models are vulnerable to adversarial attacks. Though transfer learning has been widely applied, its effect on model robustness is unclear. To figure out this problem, we conduct extensive empirical evaluations to show that fine-tuning effectively enhances model robustness under white-box FGSM attacks. We also propose a black-box attack method for transfer learning models which attacks the target model with the adversarial examples produced by its source model. To systematically measure the effect of both white-box and black-box attacks, we propose a new metric to evaluate how transferable are the adversarial examples produced by a source model to a target model. Empirical results show that the adversarial examples are more transferable when fine-tuning is used than they are when the two networks are trained independently.
更多查看译文
关键词
Transfer Learning, Neural Networks, Adversarial Attacks
AI 理解论文
溯源树
样例
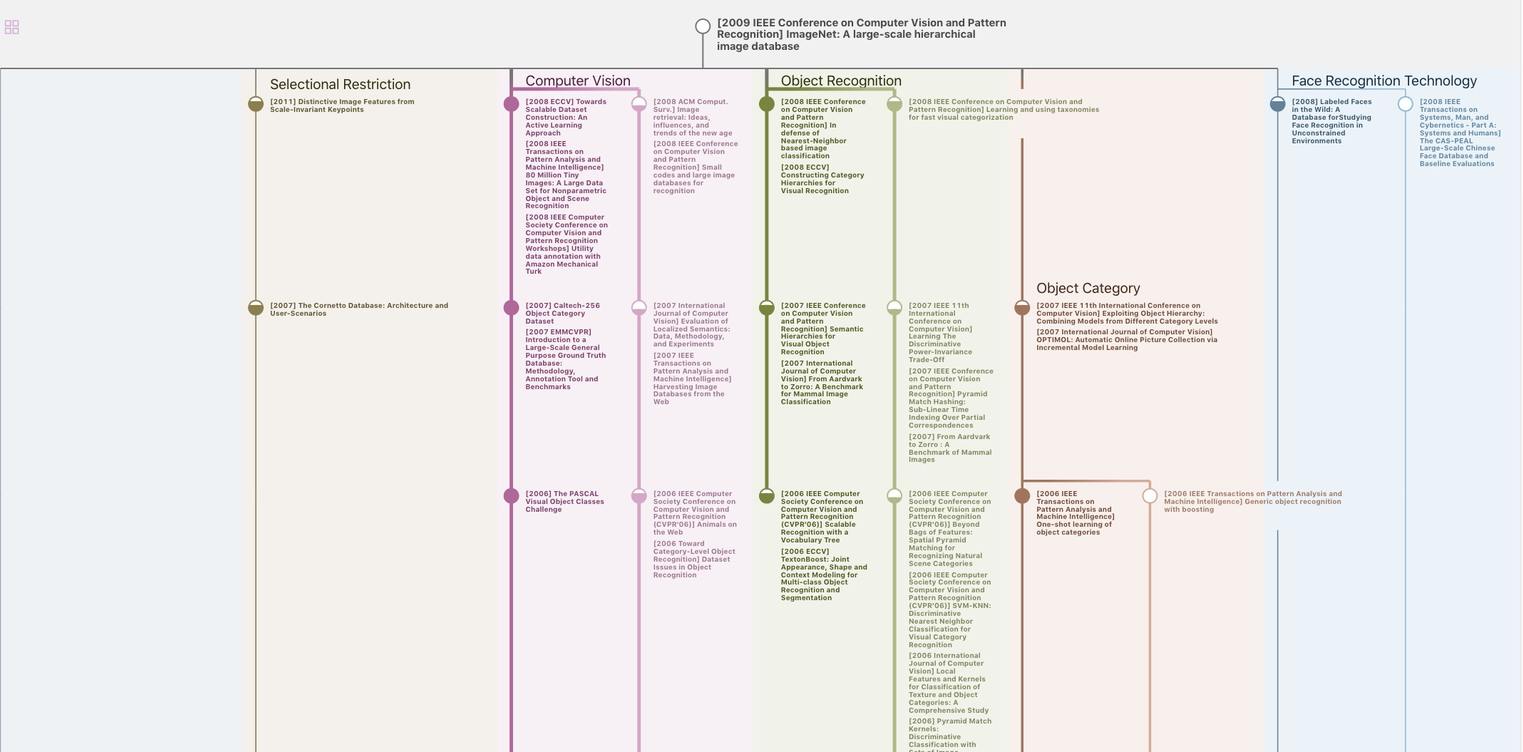
生成溯源树,研究论文发展脉络
Chat Paper
正在生成论文摘要