Time-Aware User Embeddings as a Service
KDD '20: The 26th ACM SIGKDD Conference on Knowledge Discovery and Data Mining Virtual Event CA USA July, 2020(2020)
摘要
Digital media companies typically collect rich data in the form of sequences of online user activities. Such data is used in various applications, involving tasks ranging from click or conversion prediction to recommendation or user segmentation. Nonetheless, each application depends upon specialized feature engineering that requires a lot of effort and typically disregards the time-varying nature of the online user behavior. Learning time-preserving vector representations of users (user embeddings), irrespective of a specific task, would save redundant effort and potentially lead to higher embedding quality. To that end, we address the limitations of the current state-of-the-art self-supervised methods for task-independent (unsupervised) sequence embedding, and propose a novel Time-Aware Sequential Autoencoder (TASA) that accounts for the temporal aspects of sequences of activities. The generated embeddings are intended to be readily accessible for many problem formulations and seamlessly applicable to desired tasks, thus sidestepping the burden of task-driven feature engineering. The proposed TASA shows improvements over alternative self-supervised models in terms of sequence reconstruction. Moreover, the embeddings generated by TASA yield increases in predictive performance on both proprietary and public data. It also achieves comparable results to supervised approaches that are trained on individual tasks separately and require substantially more computational effort. TASA has been incorporated within a pipeline designed to provide time-aware user embeddings as a service, and the use of its embeddings exhibited lifts in conversion prediction AUC on four audiences.
更多查看译文
关键词
user representation, neural embeddings, sequential models
AI 理解论文
溯源树
样例
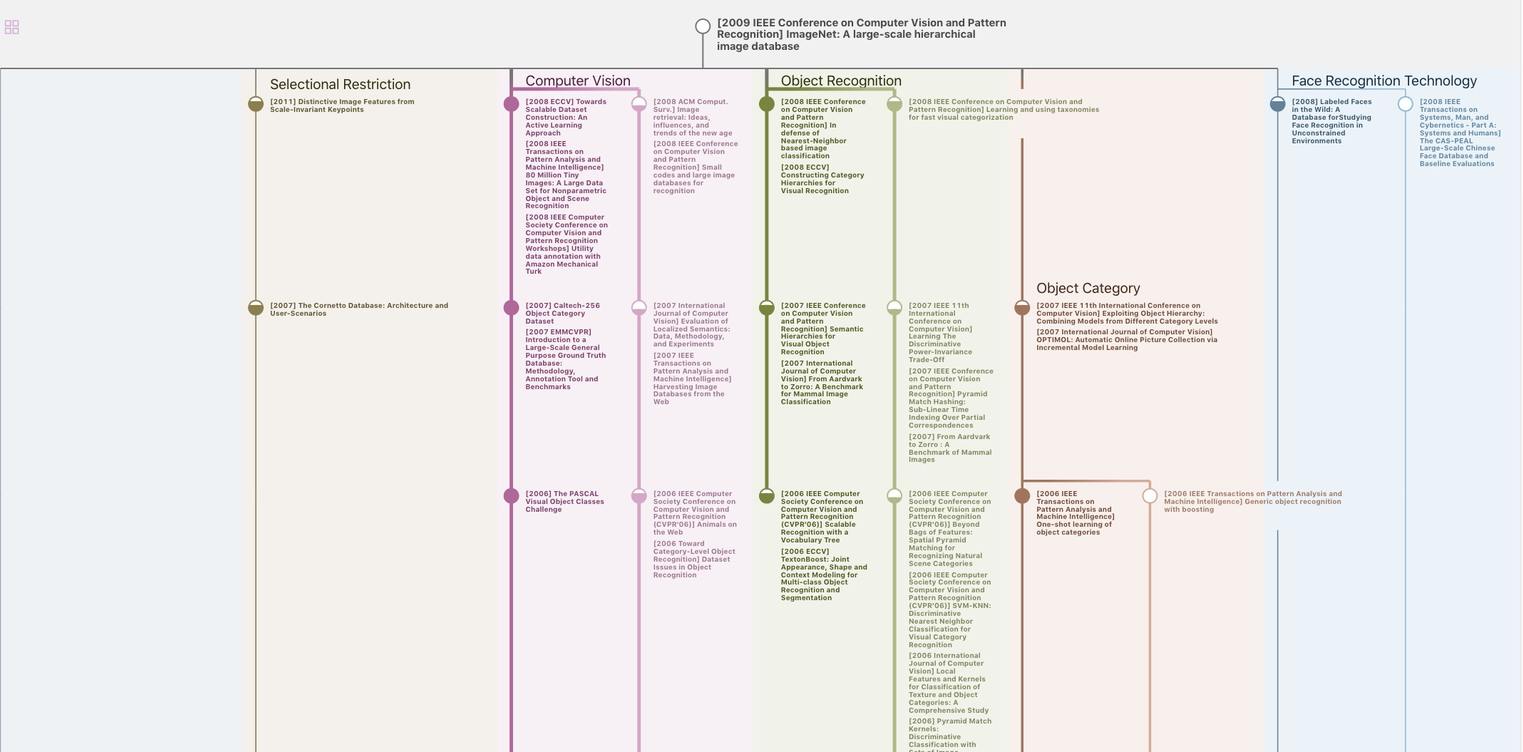
生成溯源树,研究论文发展脉络
Chat Paper
正在生成论文摘要