Rich Information is Affordable: A Systematic Performance Analysis of Second-order Optimization Using K-FAC
KDD '20: The 26th ACM SIGKDD Conference on Knowledge Discovery and Data Mining Virtual Event CA USA July, 2020(2020)
摘要
Rich information matrices from first and second-order derivatives have many potential applications in both theoretical and practical problems in deep learning. However, computing these information matrices is extremely expensive and this enormous cost is currently limiting its application to important problems regarding generalization, hyperparameter tuning, and optimization of deep neural networks. One of the most challenging use cases of information matrices is their use as a preconditioner for the optimizers, since the information matrices need to be updated every step. In this work, we conduct a step-by-step performance analysis when computing the Fisher information matrix during training of ResNet-50 on ImageNet, and show that the overhead can be reduced to the same amount as the cost of performing a single SGD step. We also show that the resulting Fisher preconditioned optimizer can converge in 1/3 the number of epochs compared to SGD, while achieving the same Top-1 validation accuracy. This is the first work to achieve such accuracy with K-FAC while reducing the training time to match that of SGD.
更多查看译文
关键词
information matrix, distributed training, performance optimization
AI 理解论文
溯源树
样例
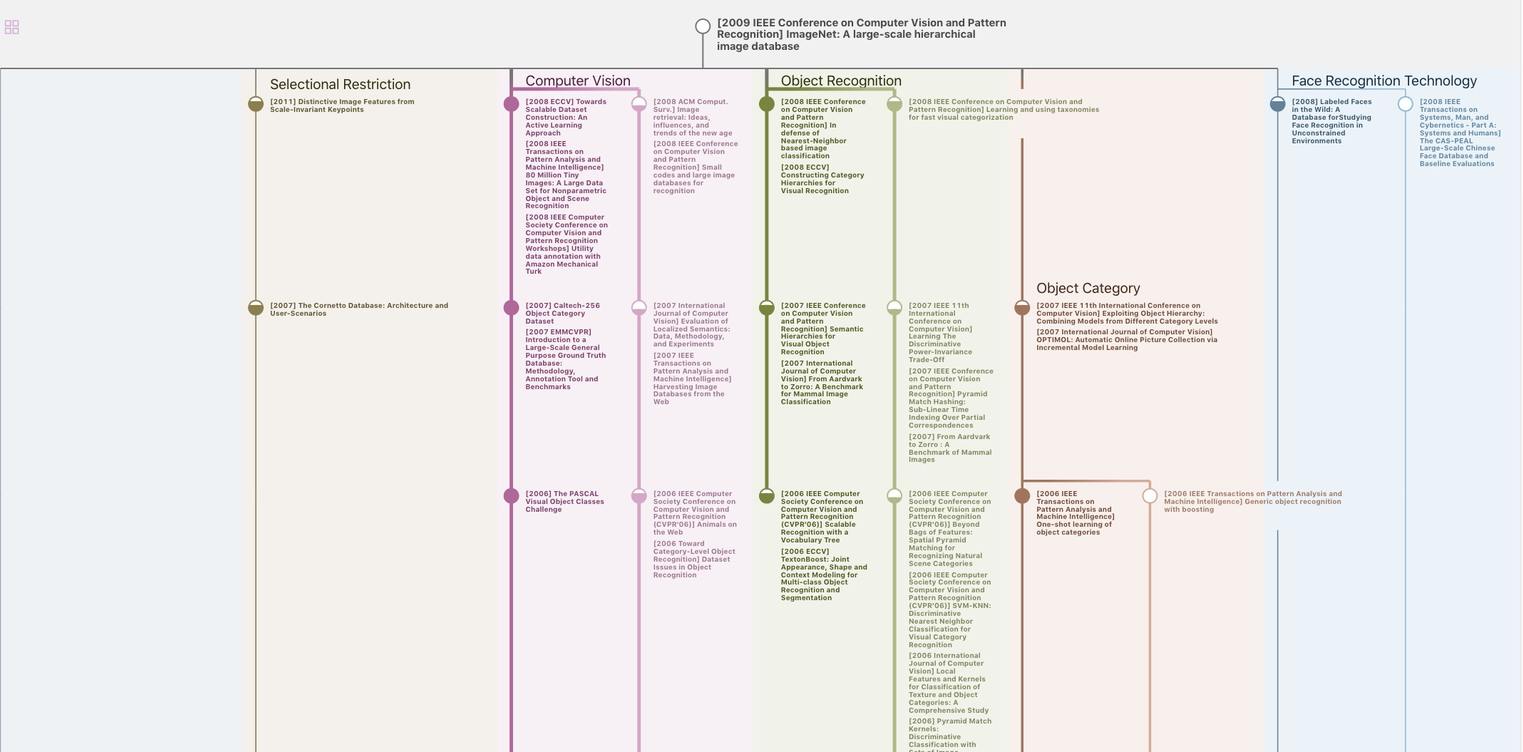
生成溯源树,研究论文发展脉络
Chat Paper
正在生成论文摘要