Explainable Deep One-Class Classification
ICLR(2021)
摘要
Deep one-class classification variants for anomaly detection learn a mapping that concentrates nominal samples in feature space causing anomalies to be mapped away. Because this transformation is highly non-linear, finding interpretations poses a significant challenge. In this paper we present an explainable deep one-class classification method, Fully Convolutional Data Description (FCDD), where the mapped samples are themselves also an explanation heatmap. FCDD yields competitive detection performance and provides reasonable explanations on common anomaly detection benchmarks with CIFAR-10 and ImageNet. On MVTec-AD, a recent manufacturing dataset offering ground-truth anomaly maps, FCDD meets the state of the art in an unsupervised setting, and outperforms its competitors in a semi-supervised setting. Finally, using FCDD's explanations we demonstrate the vulnerability of deep one-class classification models to spurious image features such as image watermarks.
更多查看译文
关键词
classification,deep,one-class
AI 理解论文
溯源树
样例
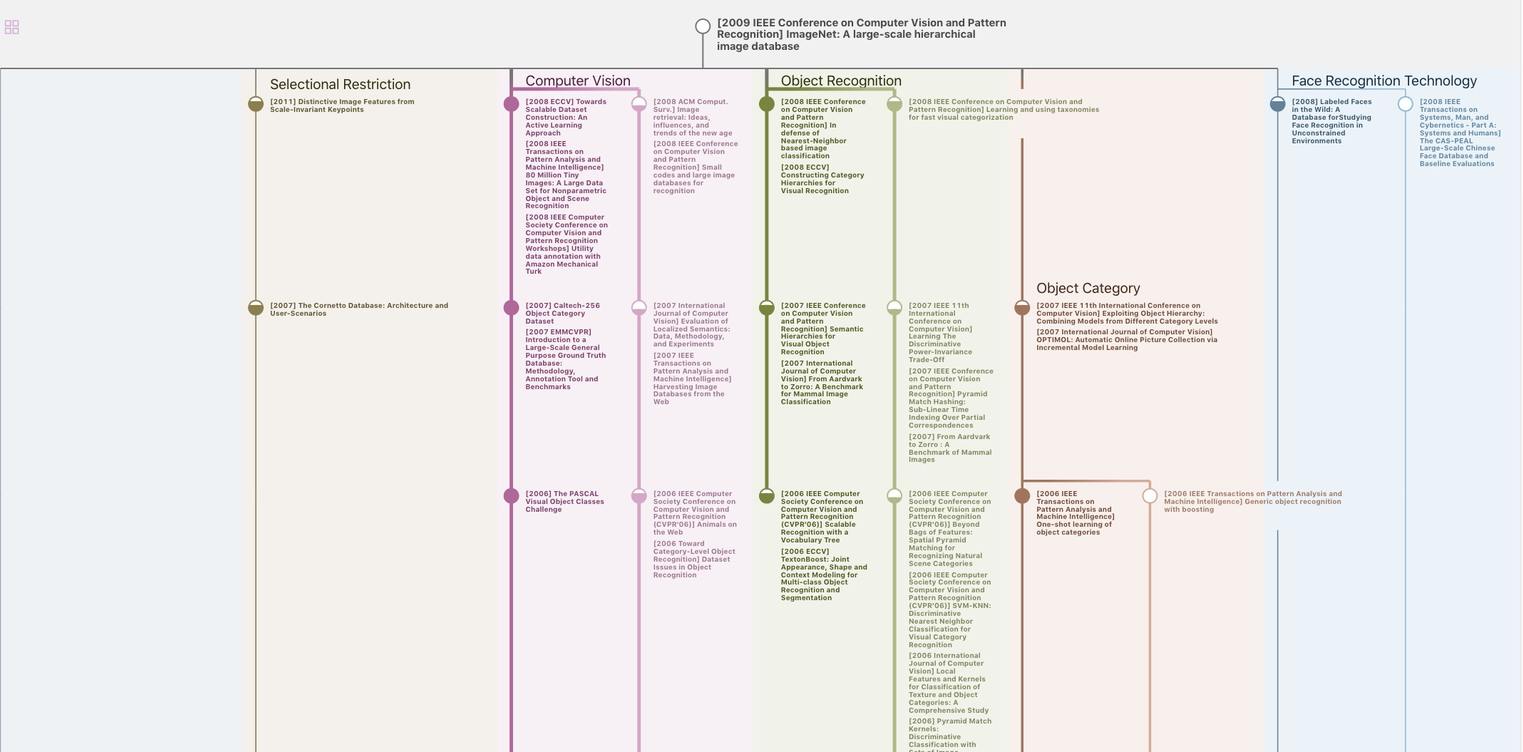
生成溯源树,研究论文发展脉络
Chat Paper
正在生成论文摘要