Copula Guided Neural Topic Modelling for Short Texts
SIGIR '20: The 43rd International ACM SIGIR conference on research and development in Information Retrieval Virtual Event China July, 2020(2020)
摘要
Extracting the topical information from documents is important for public opinion analysis, text classification, and information retrieval tasks. Compared with identifying a wide variety of topics from long documents, it is challenging to generate a concentrated topic distribution for each short message. Although this problem can be tackled by adjusting the hyper-parameters in traditional topic models such as Latent Dirichlet Allocation, it remains an open problem in neural topic modelling. In this paper, we focus on adapting the popular Auto-Encoding Variational Bayes based neural topic models to short texts, by exploring the Archimedean copulas to guide the estimated topic distributions derived from linear projected samples of re-parameterized posterior distributions. Experimental results show the superiority of our method when compared with existing neural topic models in terms of perplexity, topic coherence, and classification accuracy.
更多查看译文
关键词
Neural topic modelling, Short text modelling, Auto-encoding variational Bayes, Archimedean copulas
AI 理解论文
溯源树
样例
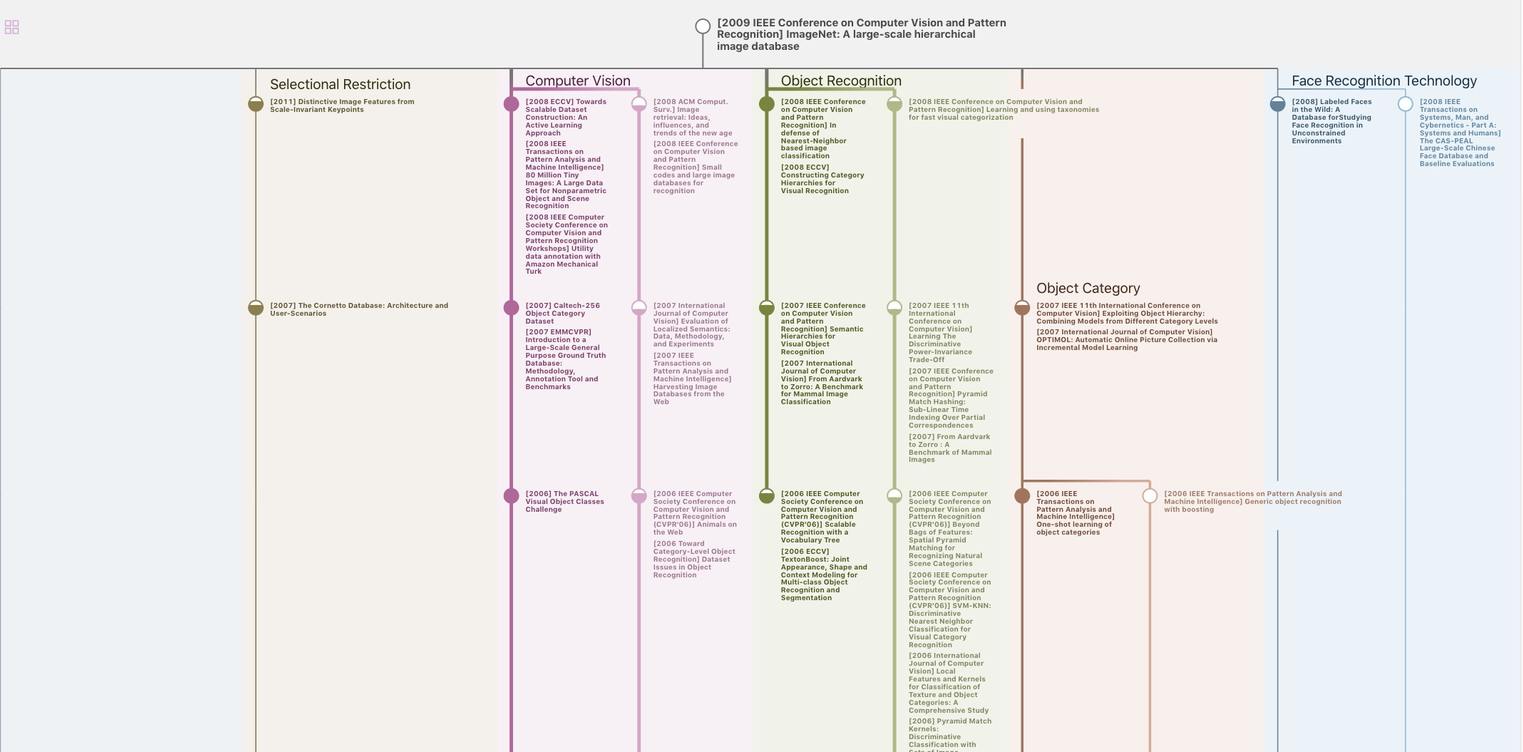
生成溯源树,研究论文发展脉络
Chat Paper
正在生成论文摘要