Dynamic Clustering with Discrete Time Event Prediction
SIGIR '20: The 43rd International ACM SIGIR conference on research and development in Information Retrieval Virtual Event China July, 2020(2020)
摘要
Discrete time event sequences from web users are commonly encountered in a variety of real-world domains, from digital marketing to recommender systems. Most of the literature in the area focuses on supervised prediction tasks of the user's next action or return time. However, little attention has been paid to the challenging task of modeling user evolution and behavior in a quantitative fashion. For example, progress of the users' knowledge in an online tutorial, user exploration, or engagement. We propose methods to perform a time-varying clustering along with event predictions in a unified domain agnostic framework. Our framework can help track the evolution of the users as they interact with the platform. We evaluate our methods on three real-world datasets and show that our method performs at par with the supervised baseline on the prediction tasks while providing meaningful clusters.
更多查看译文
关键词
Dynamic clustering, Neural networks, Discrete time events
AI 理解论文
溯源树
样例
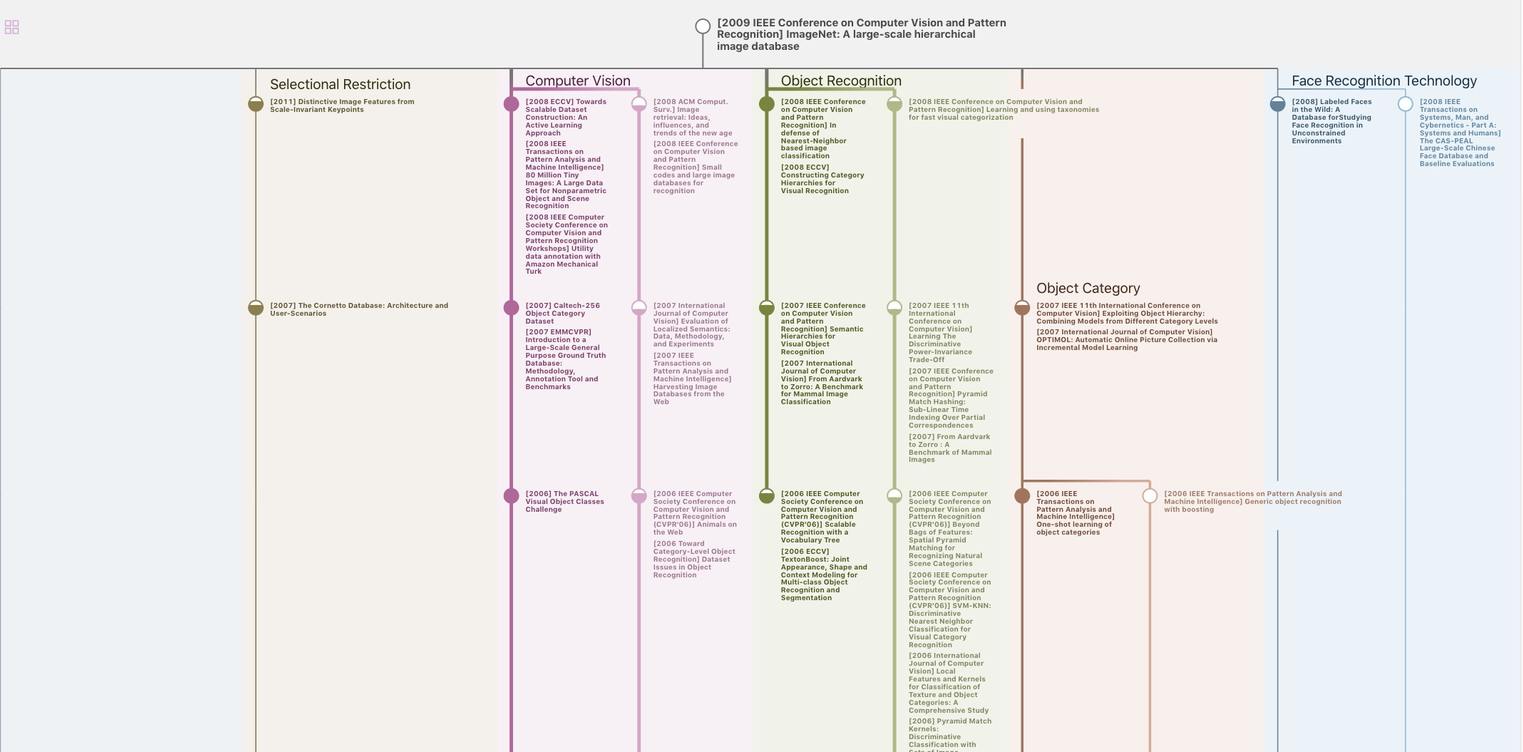
生成溯源树,研究论文发展脉络
Chat Paper
正在生成论文摘要