Three-layer Approach to Detect Anomalies in Industrial Environments based on Machine Learning
2020 IEEE Conference on Industrial Cyberphysical Systems (ICPS)(2020)
摘要
This paper introduces a general approach to design a tailored solution to detect rare events in different industrial applications based on Internet of Things (IoT) networks and machine learning algorithms. We propose a general framework based on three layers (physical, data and decision) that defines the possible designing options so that the rare events/anomalies can be detected ultra-reliably. This general framework is then applied in a well-known benchmark scenario, namely Tennessee Eastman Process. We then analyze this benchmark under three threads related to data processes: acquisition, fusion and analytics. Our numerical results indicate that: (i) event-driven data acquisition can significantly decrease the number of samples while filtering measurement noise, (ii) mutual information data fusion method can significantly decrease the variable spaces and (iii) quantitative association rule mining method for data analytics is effective for the rare event detection, identification and diagnosis. These results indicates the benefits of an integrated solution that jointly considers the different levels of data processing following the proposed general three layer framework, including details of the communication network and computing platform to be employed.
更多查看译文
关键词
cyber-physical systems,fault detection,industrial IoT,Tennessee Eastman Process
AI 理解论文
溯源树
样例
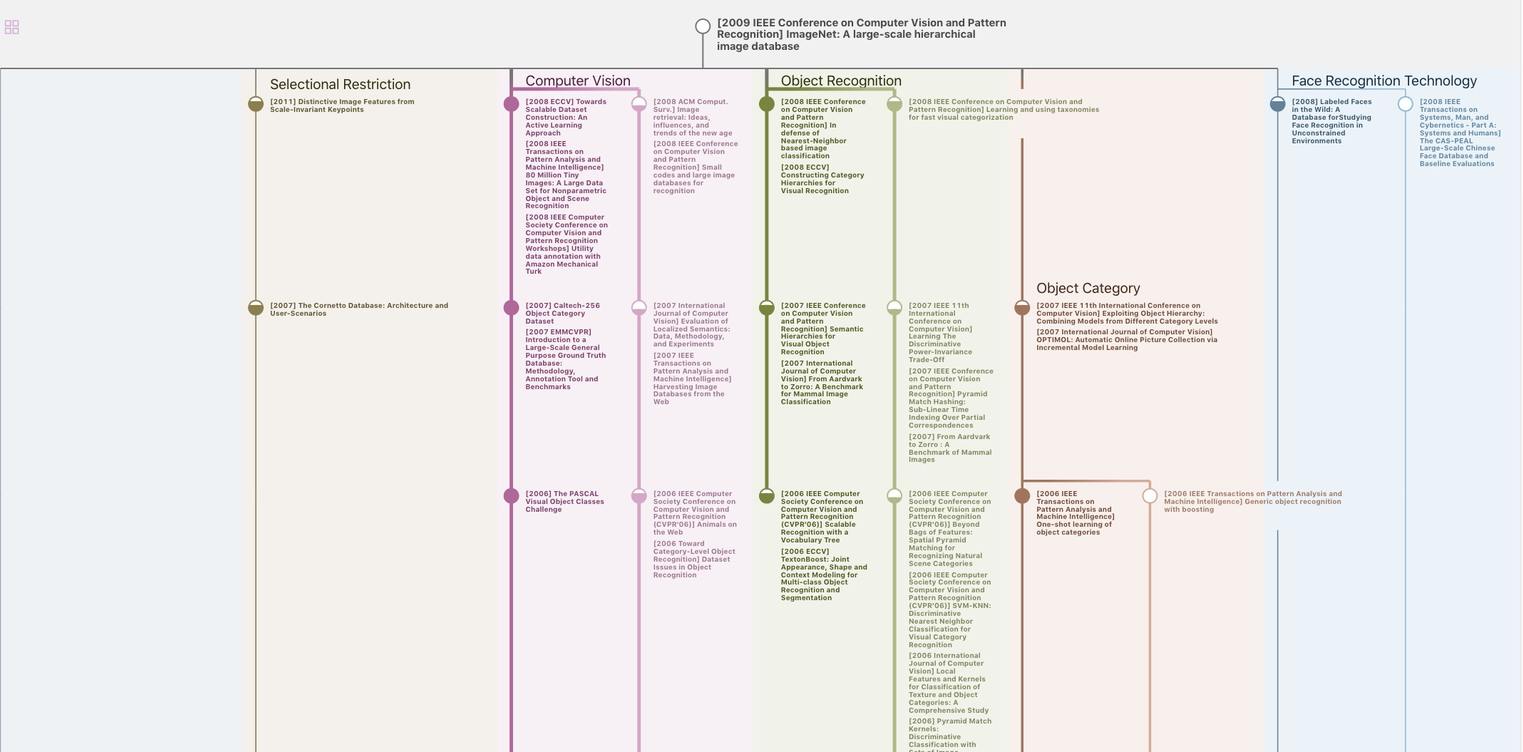
生成溯源树,研究论文发展脉络
Chat Paper
正在生成论文摘要