Multi-Task Variational Information Bottleneck
arxiv(2020)
摘要
Multi-task learning (MTL) is an important subject in machine learning and artificial intelligence. Its applications to computer vision, signal processing, and speech recognition are ubiquitous. Although this subject has attracted considerable attention recently, the performance and robustness of the existing models to different tasks have not been well balanced. This article proposes an MTL model based on the architecture of the variational information bottleneck (VIB), which can provide a more effective latent representation of the input features for the downstream tasks. Extensive observations on three public data sets under adversarial attacks show that the proposed model is competitive to the state-of-the-art algorithms concerning the prediction accuracy. Experimental results suggest that combining the VIB and the task-dependent uncertainties is a very effective way to abstract valid information from the input features for accomplishing multiple tasks.
更多查看译文
关键词
variational,information,multi-task
AI 理解论文
溯源树
样例
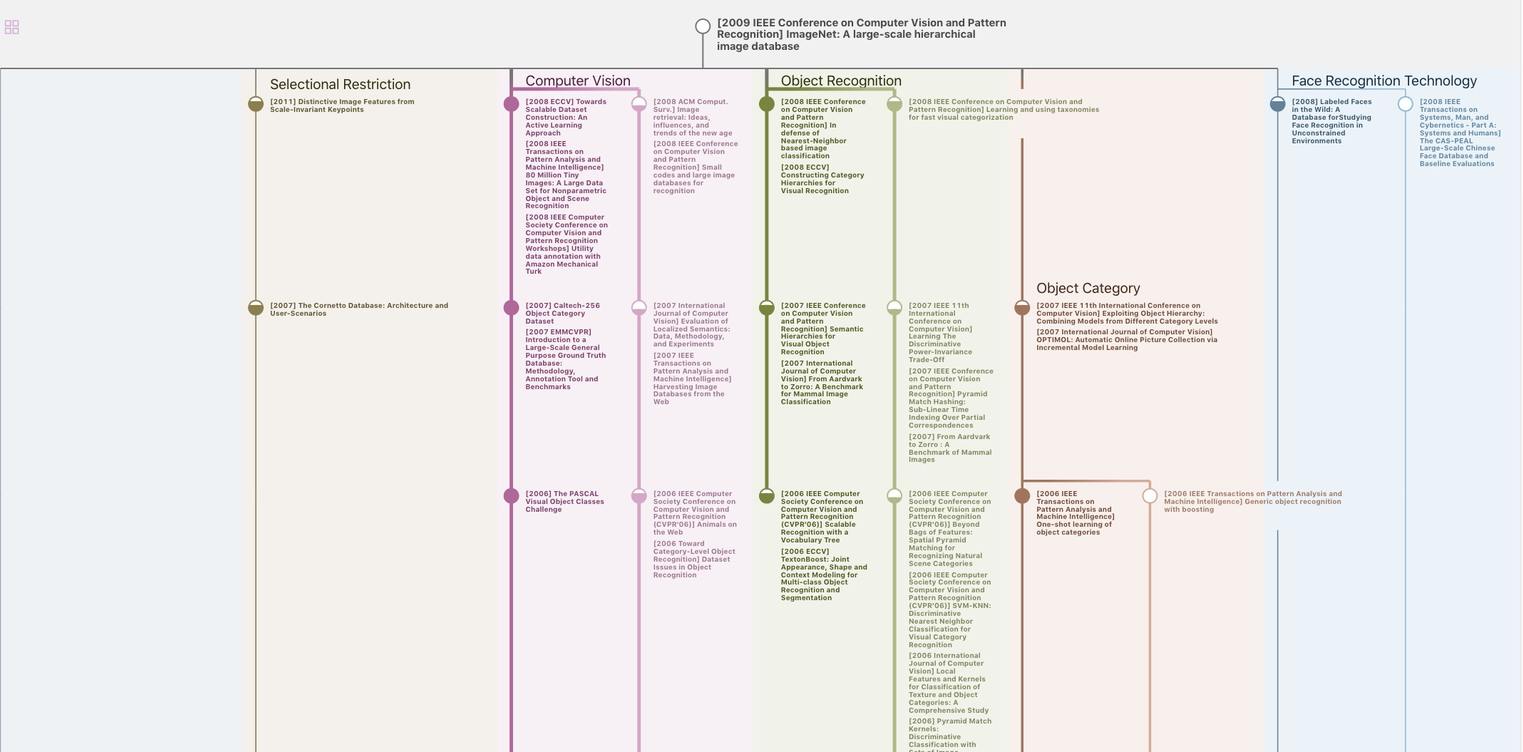
生成溯源树,研究论文发展脉络
Chat Paper
正在生成论文摘要