Quantum Spectral Clustering
PHYSICAL REVIEW A(2020)
摘要
Spectral clustering is a powerful unsupervised machine learning algorithm for clustering data with non convex or nested structures. With roots in graph theory, it uses the spectral properties of the Laplacian matrix to project the data in a low-dimensional space where clustering is more efficient. Despite its success in clustering tasks, spectral clustering suffers in practice from a fast-growing running time of O(n^3), where n is the number of points in the dataset. In this work we propose an end-to-end quantum algorithm performing spectral clustering, extending a number of works in quantum machine learning. The quantum algorithm is composed of two parts: the first is the efficient creation of the quantum state corresponding to the projected Laplacian matrix, and the second consists of applying the existing quantum analogue of the k-means algorithm. Both steps depend polynomially on the number of clusters, as well as precision and data parameters arising from quantum procedures, and polylogarithmically on the dimension of the input vectors. Our numerical simulations show an asymptotic linear growth with n when all terms are taken into account, significantly better than the classical cubic growth. This work opens the path to other graph-based quantum machine learning algorithms, as it provides routines for efficient computation and quantum access to the Incidence, Adjacency, and projected Laplacian matrices of a graph.
更多查看译文
关键词
clustering,quantum
AI 理解论文
溯源树
样例
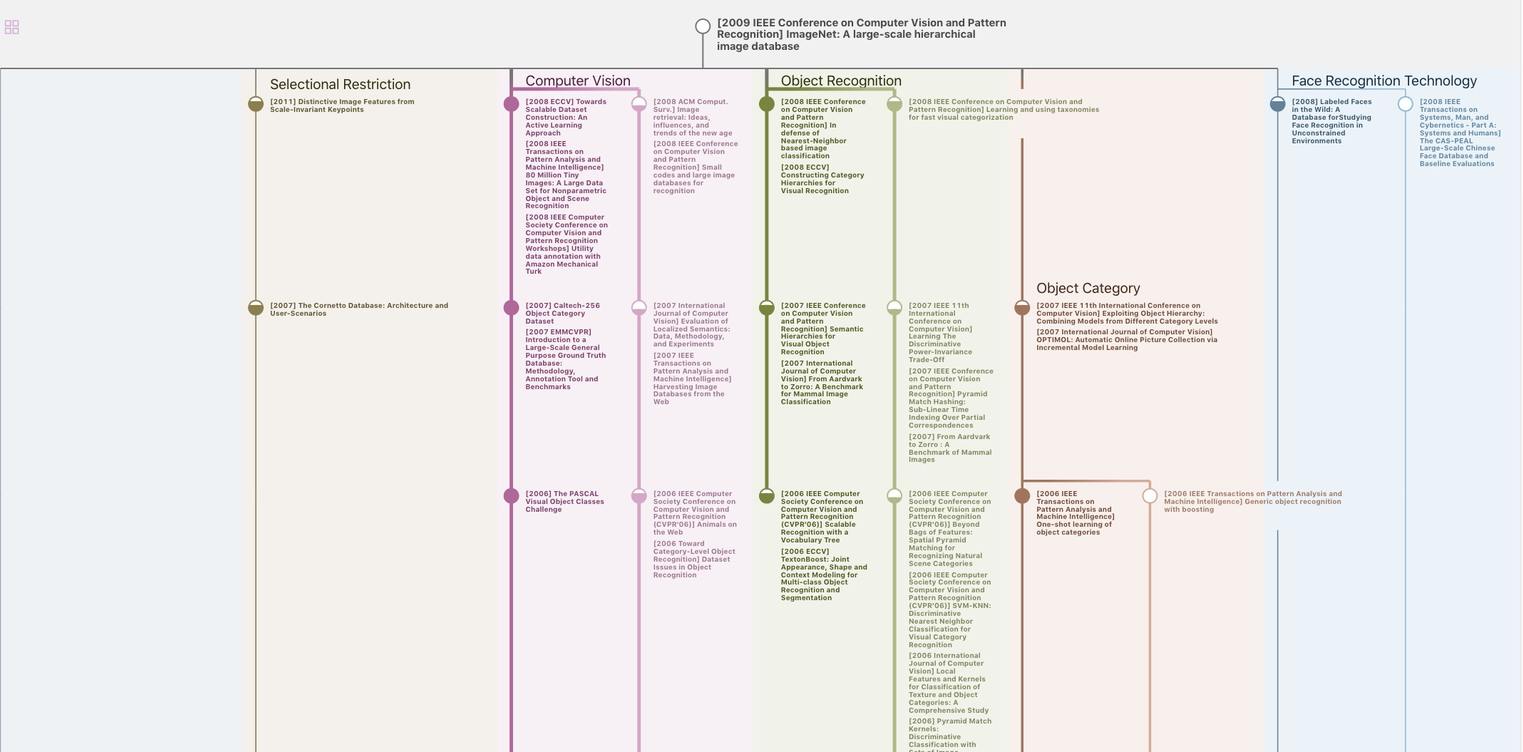
生成溯源树,研究论文发展脉络
Chat Paper
正在生成论文摘要