Reinforcement Learning for Robotic Assembly with Force Control
user-5d54d98b530c705f51c2fe5a(2020)
摘要
Today, industrial robots deployed across various industries are mostly doing repetitive tasks. The overall task performance hinges on the accuracy of their controllers to track pre-defined trajectories. To this end, endowing these machines with a greater level of intelligence to autonomously acquire skills is desirable. The main challenge is to design adaptable, yet robust, control algorithms in the face of inherent difficulties in modeling all possible system behaviors and the necessity of behavior generalization. Reinforcement learning (RL) methods hold promises for solving such challenges, because they promise agents to learn behaviors through interaction with their surrounding environments and ideally generalize to new unseen scenarios [1, 2, 3, 4].In this research, we aim to learn policies for robotic assembly in high-precision settings. Specifically, we tackle two such problems detailed in Chapter 2 and Chapter 3 respectively: 1) assembly of a rigid peg into a deformable hole, where the diameter of the hole is smaller than that of the peg; and the robot is non-compliant which only provides position and velocity control interface; 2) assembly of high-precision work-pieces, in this case robot is compliant, we can access torque control interface. In both tasks, the required precision exceeds the robot position controller’s accuracy. In real manufacturing, human labor can accomplish such high-accuracy complex tasks in a fairly easy manner. For example, a peg in hole insertion is achieved by “feeling” the contacts. This can be achieve with heuristics based on force feedback, for instance by probing the hole before inserting or moving the peg around the …
更多查看译文
AI 理解论文
溯源树
样例
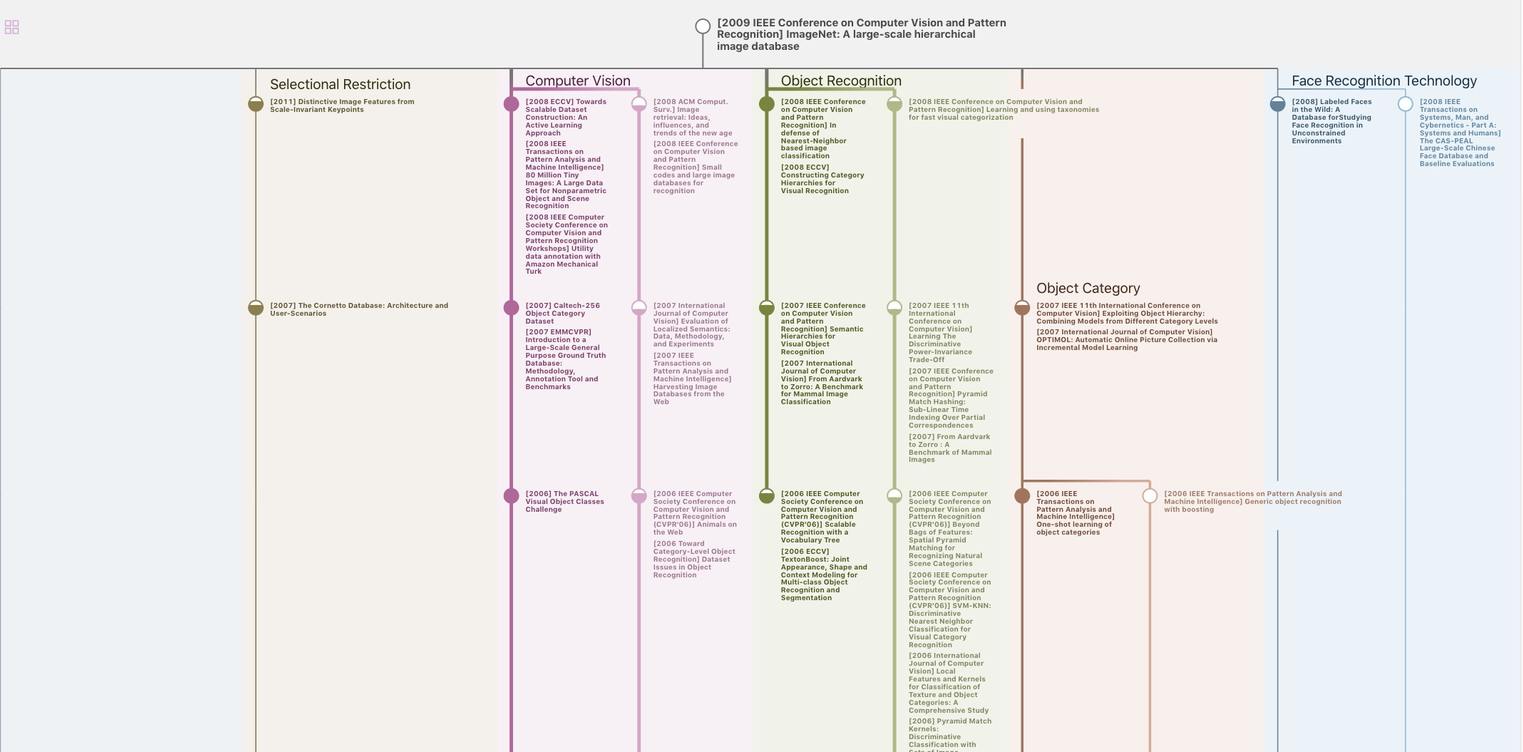
生成溯源树,研究论文发展脉络
Chat Paper
正在生成论文摘要