Lightbulb: A Photonic-Nonvolatile-Memory-Based Accelerator For Binarized Convolutional Neural Networks
PROCEEDINGS OF THE 2020 DESIGN, AUTOMATION & TEST IN EUROPE CONFERENCE & EXHIBITION (DATE 2020)(2020)
摘要
Although Convolutional Neural Networks (CNNs) have demonstrated the state-of-the-art inference accuracy in various intelligent applications, each CNN inference involves millions of expensive floating point multiply-accumulate (MAC) operations. To energy-efficiently process CNN inferences, prior work proposes an electro-optical accelerator to process powerof-2 quantized CNNs by electro-optical ripple-carry adders and optical binary shifters. The electro-optical accelerator also uses SRAM registers to store intermediate data. However, electrooptical ripple-carry adders and SRAMs seriously limit the operating frequency and inference throughput of the electrooptical accelerator, due to the long critical path of the adder and the long access latency of SRAMs. In this paper, we propose a photonic nonvolatile memory (NVM)-based accelerator, LightBulb, to process binarized CNNs by high frequency photonic XNOR gates and popcount units. LightBulb also adopts photonic racetrack memory to serve as input/output registers to achieve high operating frequency. Compared to prior electro-optical accelerators, on average, LightBulb improves the CNN inference throughput by 17x similar to 173x and the inference throughput per Watt by 17.5x similar to 660x.
更多查看译文
关键词
Optical Accelerator, Photonic Racetrack Memory, Photonic Phase Change Memory, Binarized Neural Network
AI 理解论文
溯源树
样例
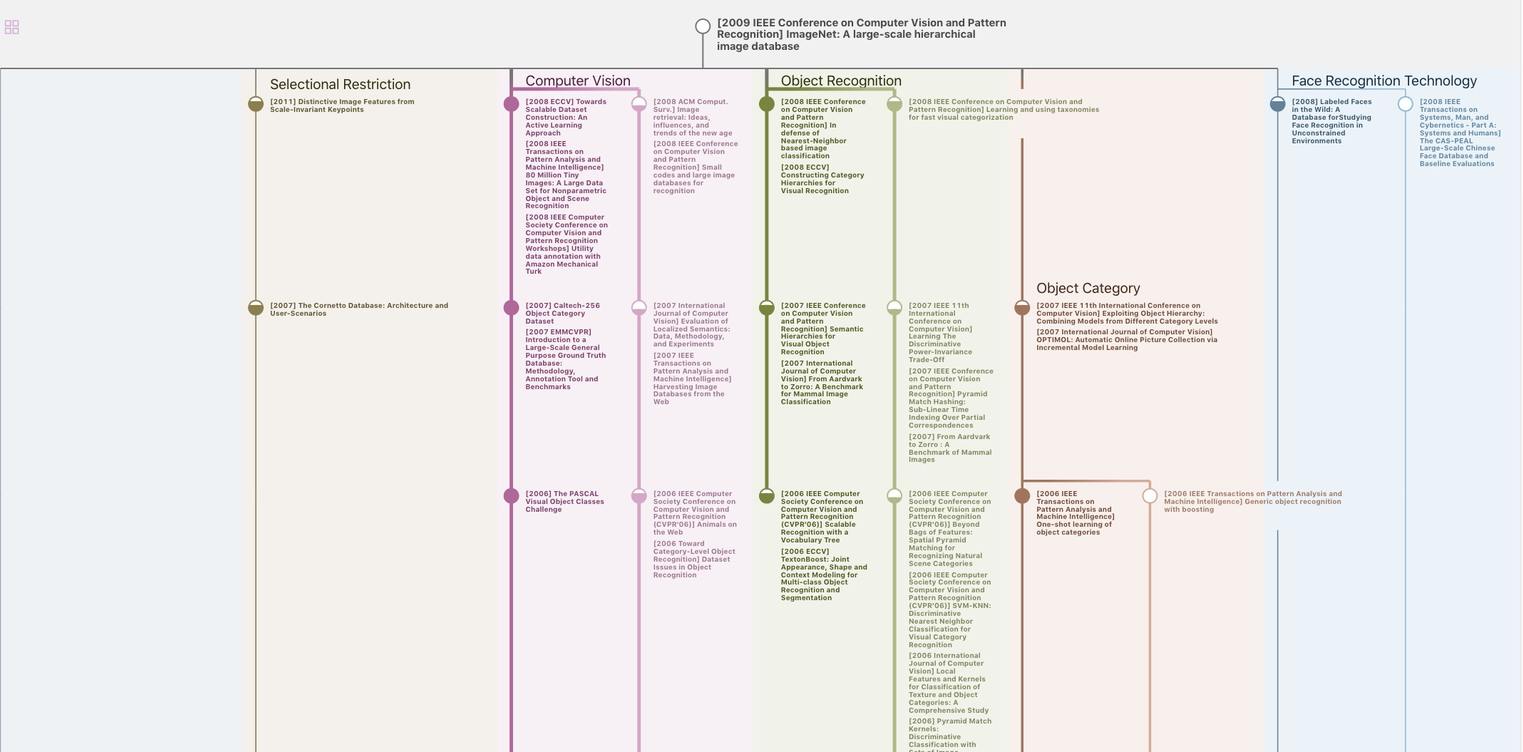
生成溯源树,研究论文发展脉络
Chat Paper
正在生成论文摘要