Automatic Generation of FPGA Kernels From Open Format CNN Models
2020 IEEE 28th Annual International Symposium on Field-Programmable Custom Computing Machines (FCCM)(2020)
摘要
The continuing exponential increase of deep learning applications like image classification or object detection requires faster and faster processing speeds while keeping the development time small. Specifically, there is a broad interest for unifying machine learning models into a universal ecosystem so that developers can benefit from framework interoperability and seamless device-specific acceleration. This is a more challenging task for FPGAs which are promising platforms but need extra effort in order to be part of this ecosystem. This work is based on an early development stage open-source project which is called HLS4ML originally created for particle physics applications via the automatic translation of neural networks on embedded Xilinx FPGAs. Our proposed solution involves a generalized optimization scheme on top of HLS4ML that automatically converts open format AI models called ONNX for cloud FPGAs. Our design also achieved in a demonstrated inference $102 \times $ over single-core CPU and $6.6 \times $ over GPU with a good tradeoff between accuracy.
更多查看译文
关键词
deep learning,machine learning,universal ecosystem,framework interoperability,open-source project,HLS4ML,particle physics applications,automatic translation,neural networks,embedded Xilinx FPGAs,generalized optimization scheme,AI models,cloud FPGAs,automatic generation,FPGA kernels,open format CNN models,device-specific acceleration,ONNX
AI 理解论文
溯源树
样例
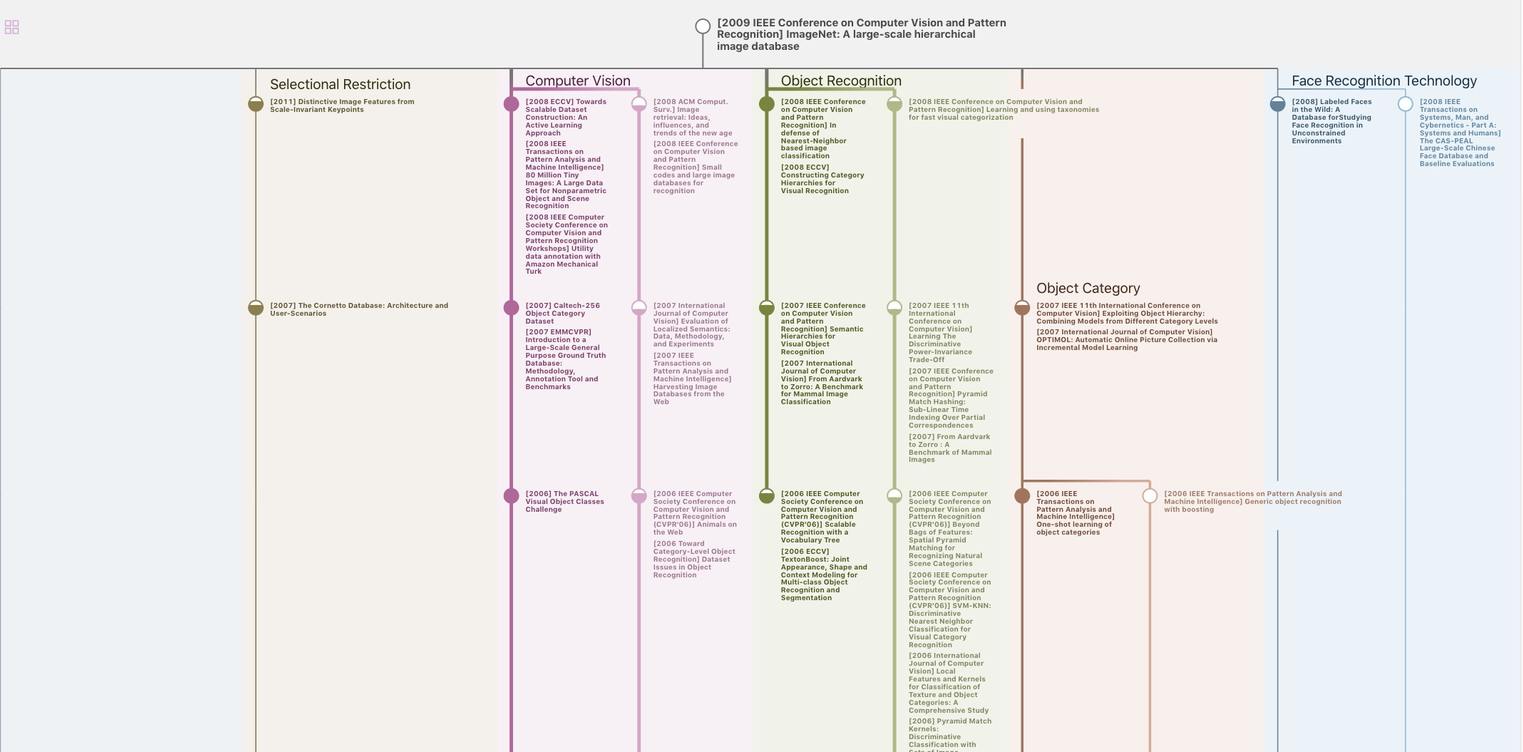
生成溯源树,研究论文发展脉络
Chat Paper
正在生成论文摘要