High Dimensional Channel Estimation Using Deep Generative Networks
IEEE Journal on Selected Areas in Communications(2020)
摘要
This paper presents a novel compressed sensing (CS) approach to high dimensional wireless channel estimation by optimizing the input to a deep generative network. Channel estimation using generative networks relies on the assumption that the reconstructed channel lies in the range of a generative model. Channel reconstruction using generative priors outperforms conventional CS techniques and requires fewer pilots. It also eliminates the need of a priori knowledge of the sparsifying basis, instead using the structure captured by the deep generative model as a prior. Using this prior, we also perform channel estimation from one-bit quantized pilot measurements, and propose a novel optimization objective function that attempts to maximize the correlation between the received signal and the generator's channel estimate while minimizing the rank of the channel estimate. Our approach significantly outperforms sparse signal recovery methods such as Orthogonal Matching Pursuit (OMP) and Approximate Message Passing (AMP) algorithms such as EM-GM-AMP for narrowband mmWave channel reconstruction, and its execution time is not noticeably affected by the increase in the number of received pilot symbols.
更多查看译文
关键词
MIMO channel estimation,generative adversarial networks (GAN),compressed sensing,one-bit receivers
AI 理解论文
溯源树
样例
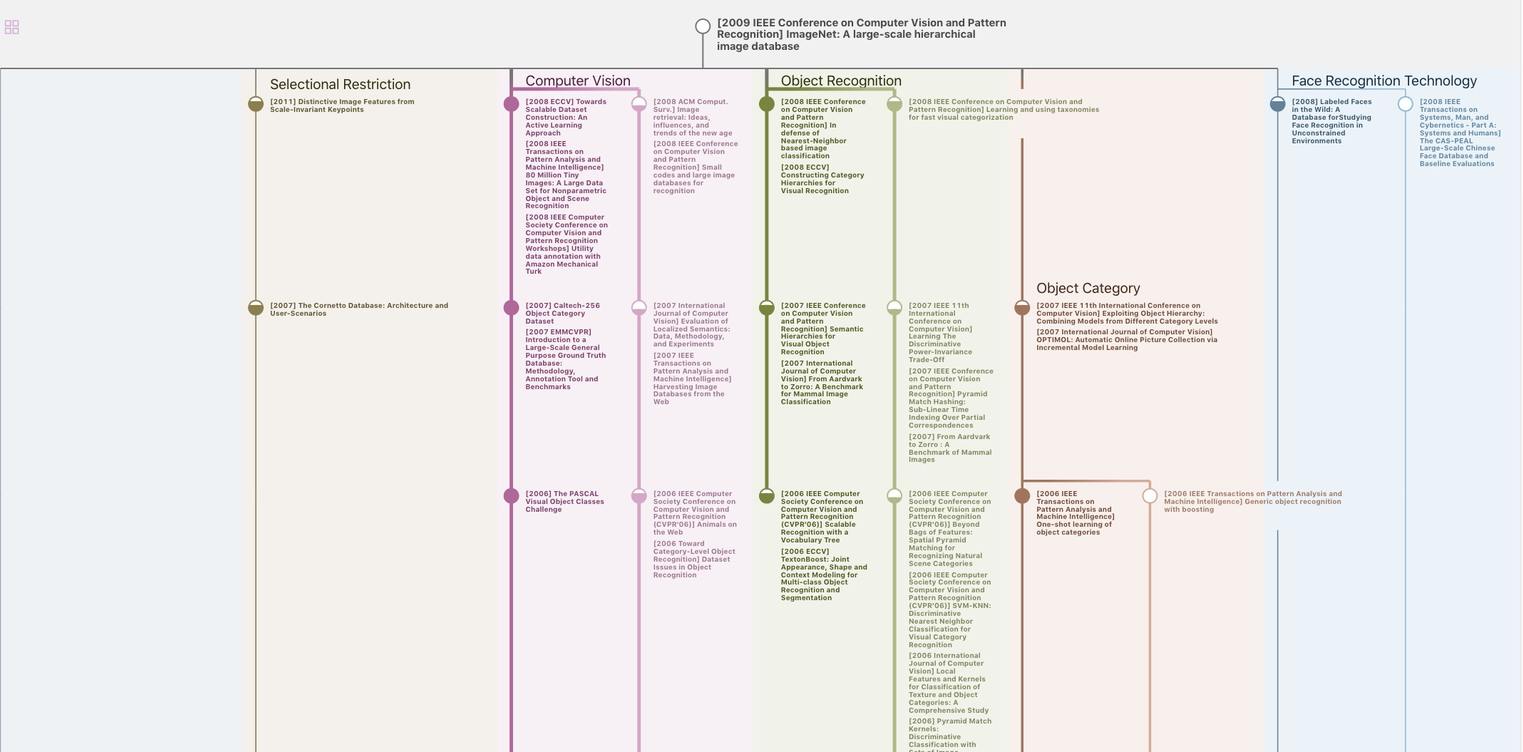
生成溯源树,研究论文发展脉络
Chat Paper
正在生成论文摘要