Learning dynamics for improving control of overactuated flying systems
IEEE Robotics and Automation Letters(2020)
摘要
Overactuated omnidirectional flying vehicles are capable of generating force and torque in any direction, which is important for applications such as contact-based industrial inspection. This comes at the price of an increase in model complexity. These vehicles usually have non-negligible, repetitive dynamics that are hard to model, such as the aerodynamic interference between the propellers. This makes it difficult for high-performance trajectory tracking using a model-based controller. This paper presents an approach that combines a data-driven and a first-principle model for the system actuation and uses it to improve the controller. In a first step, the first-principle model errors are learned offline using a Gaussian Process (GP) regressor. At runtime, the first-principle model and the GP regressor are used jointly to obtain control commands. This is formulated as an optimization problem, which avoids ambiguous solutions present in a standard inverse model in overactuated systems, by only using forward models. The approach is validated using a tilt-arm overactuated omnidirectional flying vehicle performing attitude trajectory tracking. The results show that with our proposed method, the attitude trajectory error is reduced by 32 PID controller.
更多查看译文
关键词
Aerial Systems: Mechanics and Control,Model Learning for Control
AI 理解论文
溯源树
样例
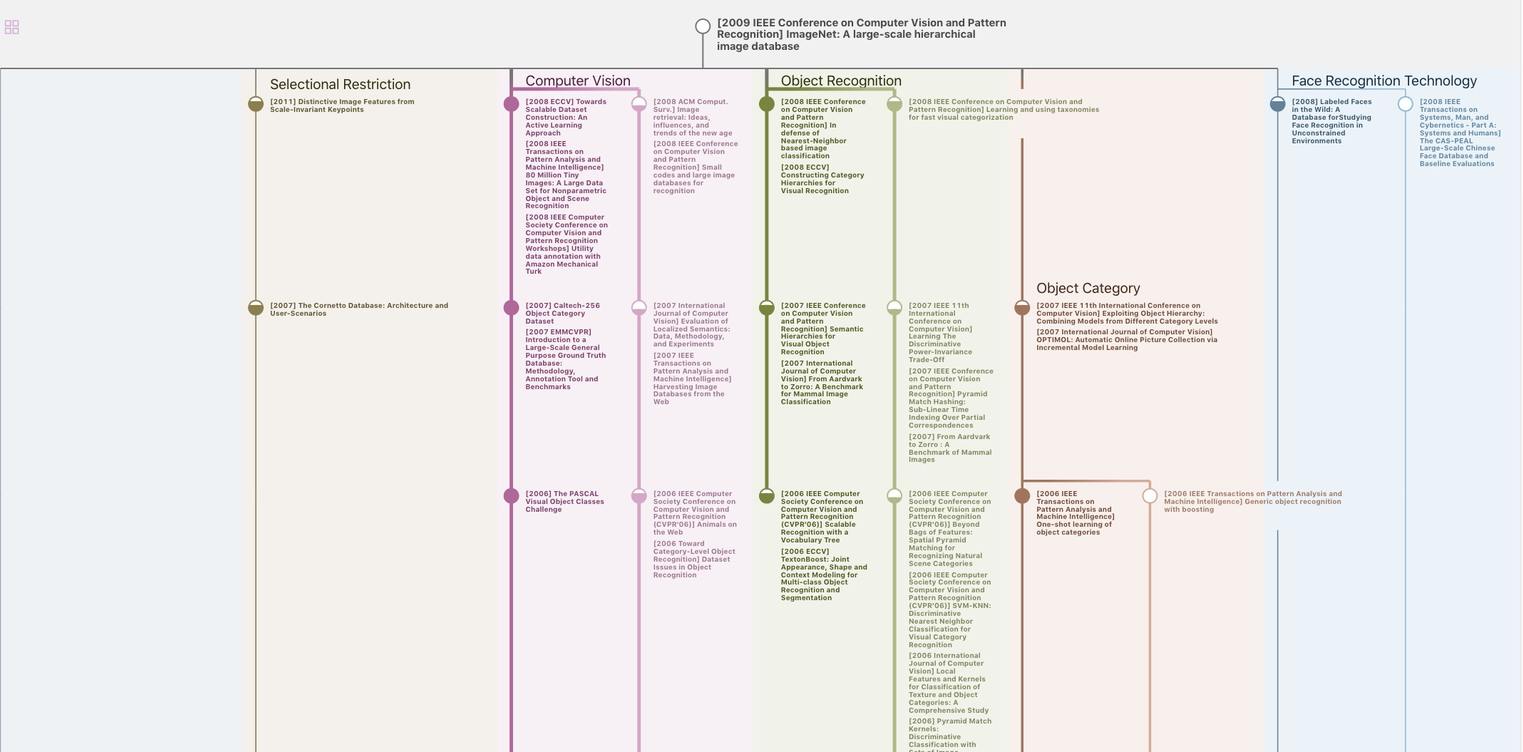
生成溯源树,研究论文发展脉络
Chat Paper
正在生成论文摘要