Generalisation Guarantees for Continual Learning with Orthogonal Gradient Descent
CoRR(2020)
摘要
In Continual Learning settings, deep neural networks are prone to Catastrophic Forgetting. Orthogonal Gradient Descent was proposed to tackle the challenge. However, no theoretical guarantees have been proven yet. We present a theoretical framework to study Continual Learning algorithms in the Neural Tangent Kernel regime. This framework comprises closed form expression of the model through tasks and proxies for Transfer Learning, generalisation and tasks similarity. In this framework, we prove that OGD is robust to Catastrophic Forgetting then derive the first generalisation bound for SGD and OGD for Continual Learning. Finally, we study the limits of this framework in practice for OGD and highlight the importance of the Neural Tangent Kernel variation for Continual Learning with OGD.
更多查看译文
关键词
continual learning,orthogonal
AI 理解论文
溯源树
样例
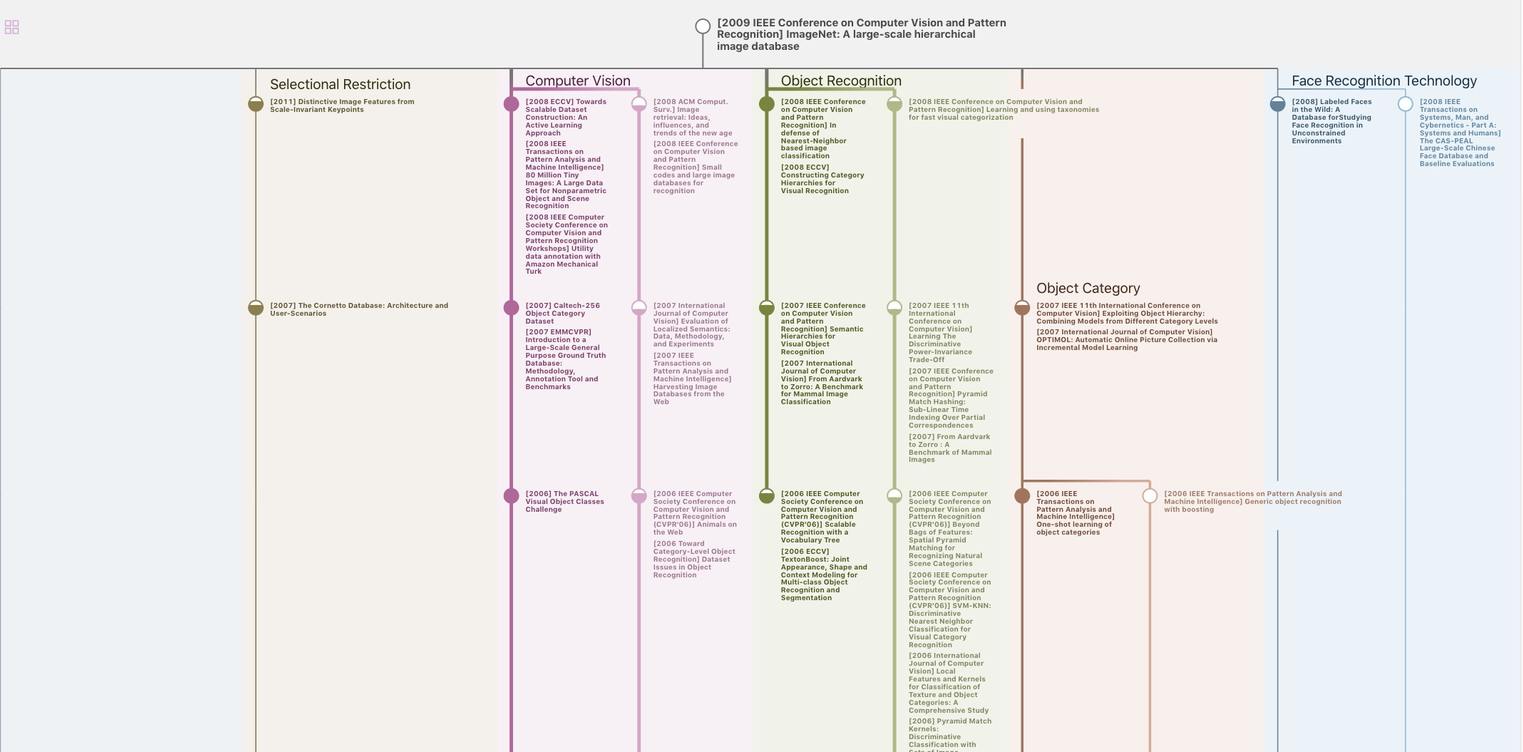
生成溯源树,研究论文发展脉络
Chat Paper
正在生成论文摘要