Baselines for Chest X-Ray Report Generation.
ML4H@NeurIPS(2020)
摘要
With advances in deep learning and image captioning over the past few years, researchers have recently begun applying computer vision methods to radiology report generation. Typically, these generated reports have been evaluated using general domain natural language generation (NLG) metrics like CIDEr and BLEU. However, there is little work assessing how appropriate these metrics are for healthcare, where correctness is critically important. In this work, we profile a number of models for automatic report generation on this dataset, including: random report retrieval, nearest neighbor report retrieval, n-gram language models, and neural network approaches. These models serve to calibrate our understanding for what the opaque general domain NLG metrics mean. In particular, we find that the standard NLG metrics (e.g. BLEU, CIDEr) actually assign higher scores to random (but grammatical) clinical sentences over n-gram-derived sentences, despite the n-gram sentences achieving higher clinical accuracy. This casts doubt on the usefulness of these domain-agnostic metrics, though unsurprisingly we find that the best performance-on both CIDEr/BLEU and clinical correctness-was achieved by more sophisticated models.
更多查看译文
AI 理解论文
溯源树
样例
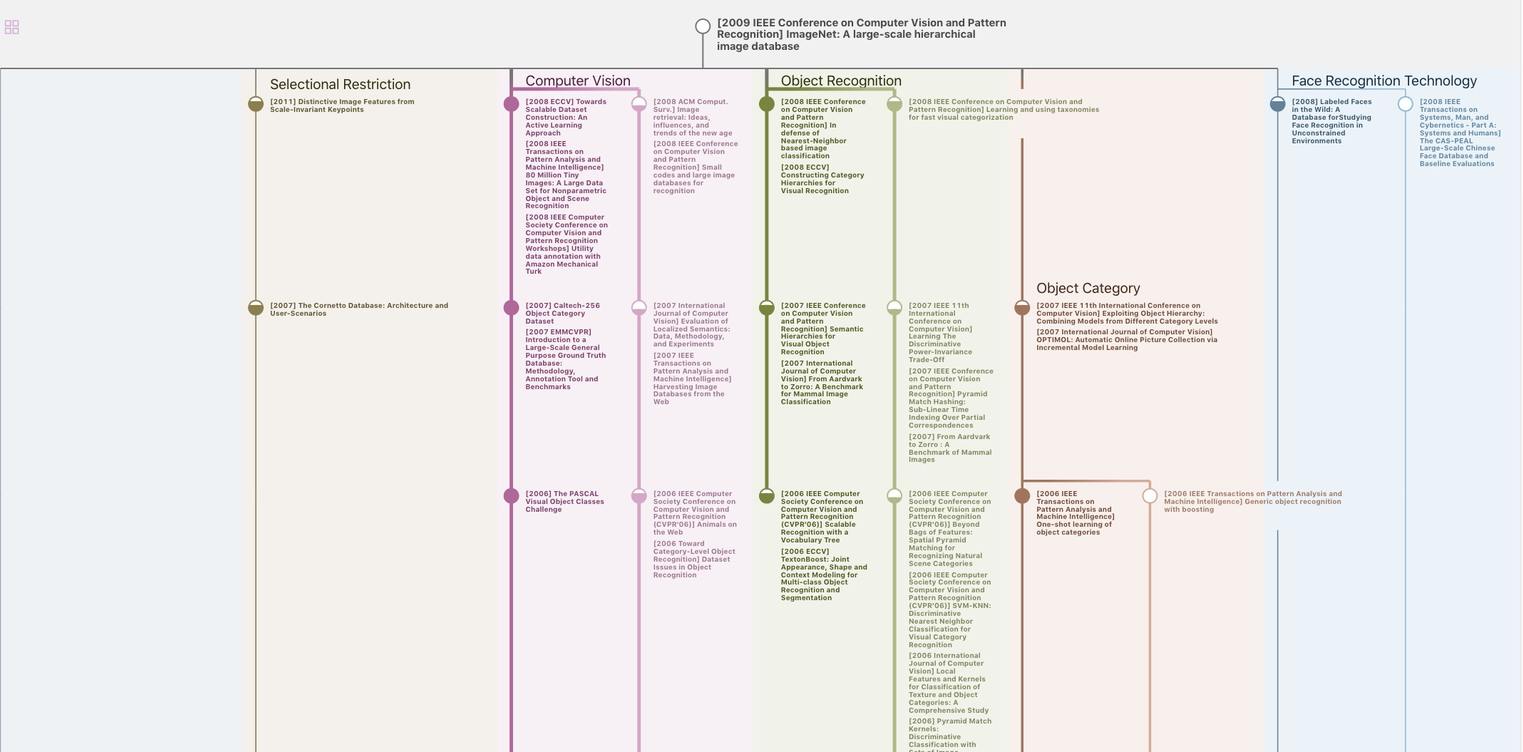
生成溯源树,研究论文发展脉络
Chat Paper
正在生成论文摘要