Iterative Regularization With Convex Regularizers
AISTATS(2021)
摘要
We study iterative/implicit regularization for linear models, when the bias is convex but not necessarily strongly convex. We characterize the stability properties of a primaldual gradient based approach, analyzing its convergence in the presence of worst case deterministic noise. As a main example, we specialize and illustrate the results for the problem of robust sparse recovery. Key to our analysis is a combination of ideas from regularization theory and optimization in the presence of errors. Theoretical results are complemented by experiments showing that state-of-the-art performances can be achieved with considerable computational speed-ups.
更多查看译文
AI 理解论文
溯源树
样例
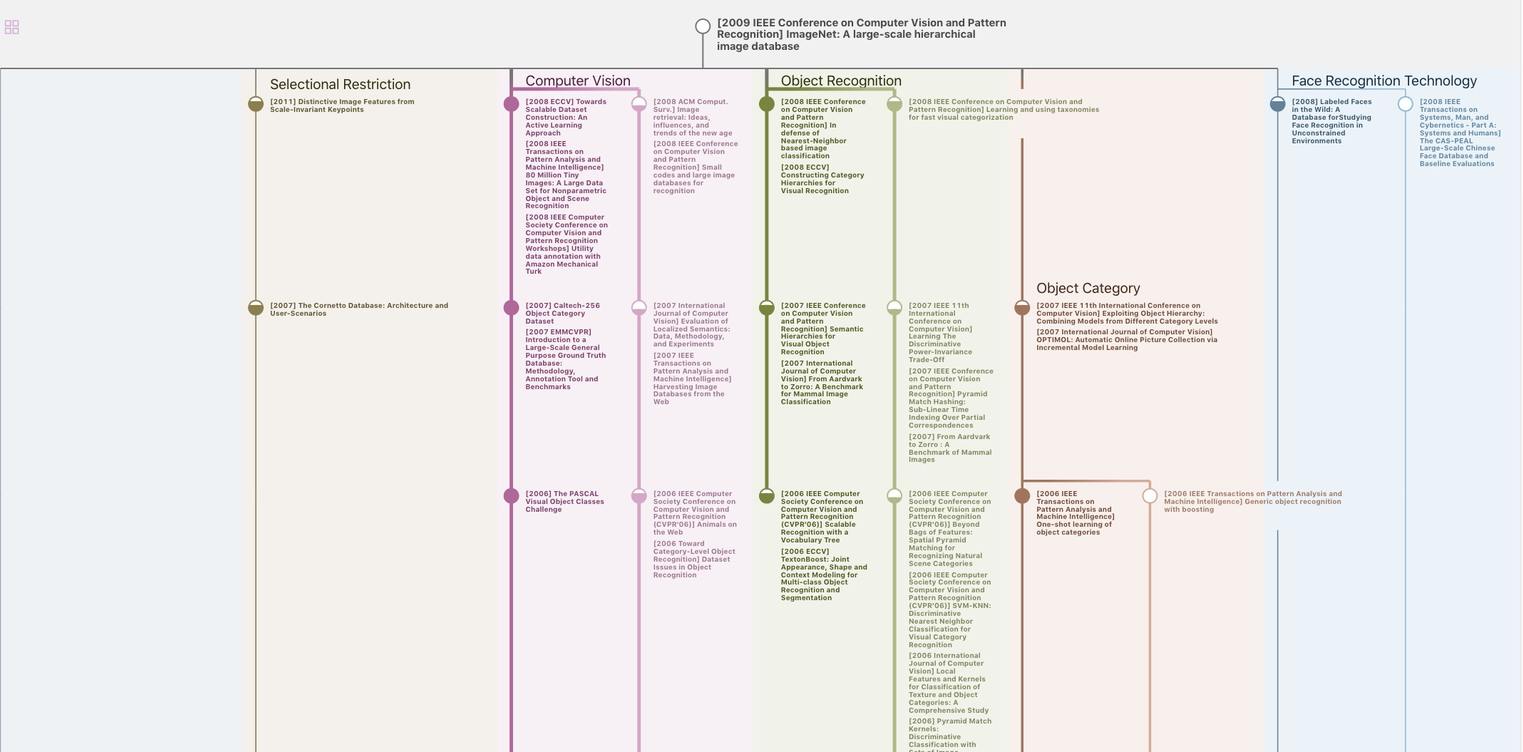
生成溯源树,研究论文发展脉络
Chat Paper
正在生成论文摘要