A Note on the Global Convergence of Multilayer Neural Networks in the Mean Field Regime
arxiv(2020)
摘要
In a recent work, we introduced a rigorous framework to describe the mean field limit of the gradient-based learning dynamics of multilayer neural networks, based on the idea of a neuronal embedding. There we also proved a global convergence guarantee for three-layer (as well as two-layer) networks using this framework. In this companion note, we point out that the insights in our previous work can be readily extended to prove a global convergence guarantee for multilayer networks of any depths. Unlike our previous three-layer global convergence guarantee that assumes i.i.d. initializations, our present result applies to a type of correlated initialization. This initialization allows to, at any finite training time, propagate a certain universal approximation property through the depth of the neural network. To achieve this effect, we introduce a bidirectional diversity condition.
更多查看译文
关键词
multilayer neural networks,mean field regime,neural networks,global convergence
AI 理解论文
溯源树
样例
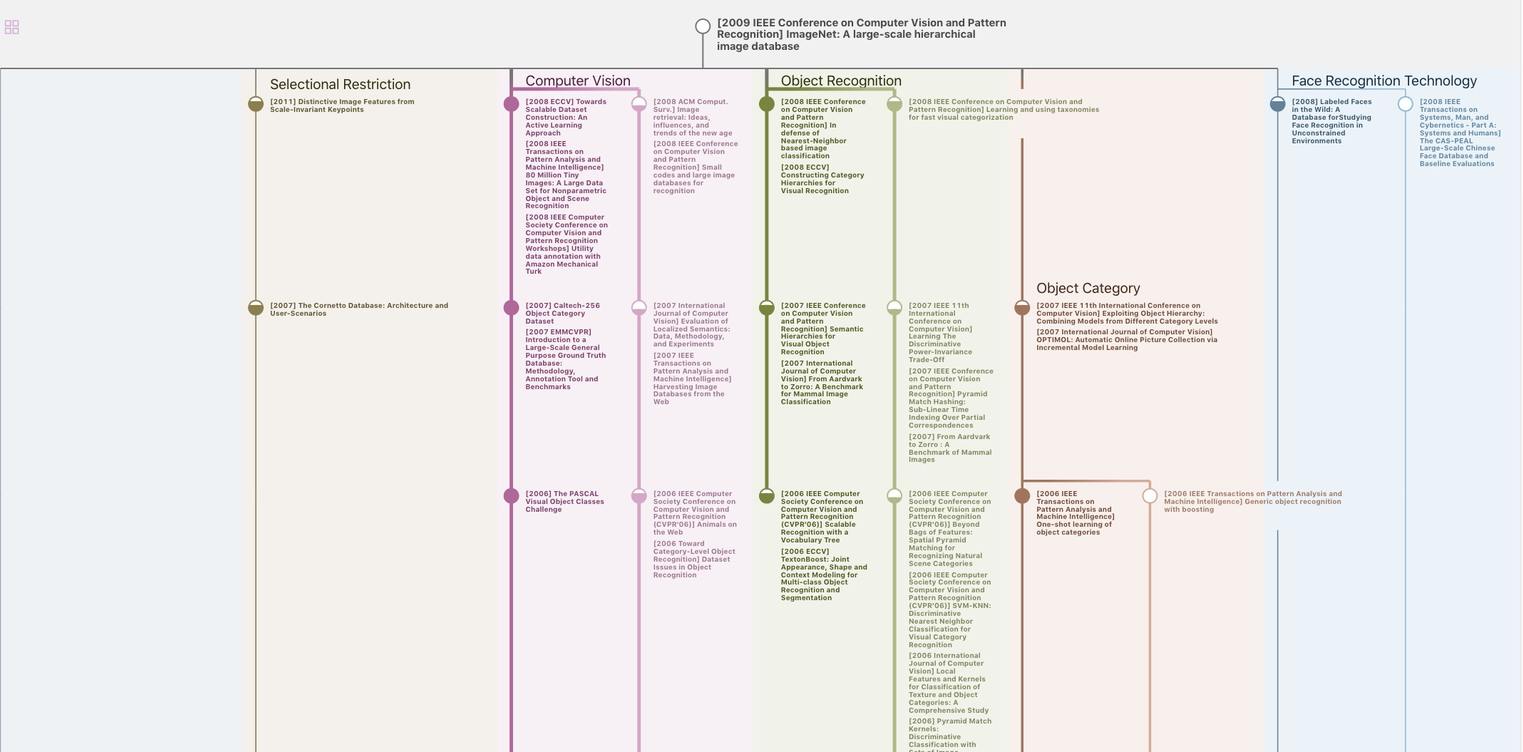
生成溯源树,研究论文发展脉络
Chat Paper
正在生成论文摘要