Synthetic A/B Testing using Synthetic Interventions
arxiv(2023)
摘要
Suppose there are $N$ units and $D$ interventions. We aim to learn the average potential outcome associated with every unit-intervention pair, i.e., $N \times D$ causal parameters. While running $N \times D$ experiments is conceivable, it can be expensive or infeasible. This work introduces an experiment design, synthetic A/B testing, and the synthetic interventions (SI) estimator to recover all $N \times D$ causal parameters while observing each unit under at most two interventions, independent of $D$. Under a novel tensor factor model for potential outcomes across units, measurements, and interventions, we establish the identification of each parameter. Further, we show the SI estimator is finite-sample consistent and asymptotically normal. Collectively, these also lead to novel results for panel data settings, particularly for synthetic controls. We empirically validate our experiment design using real e-commerce data from a large-scale A/B test.
更多查看译文
关键词
synthetic interventions,testing
AI 理解论文
溯源树
样例
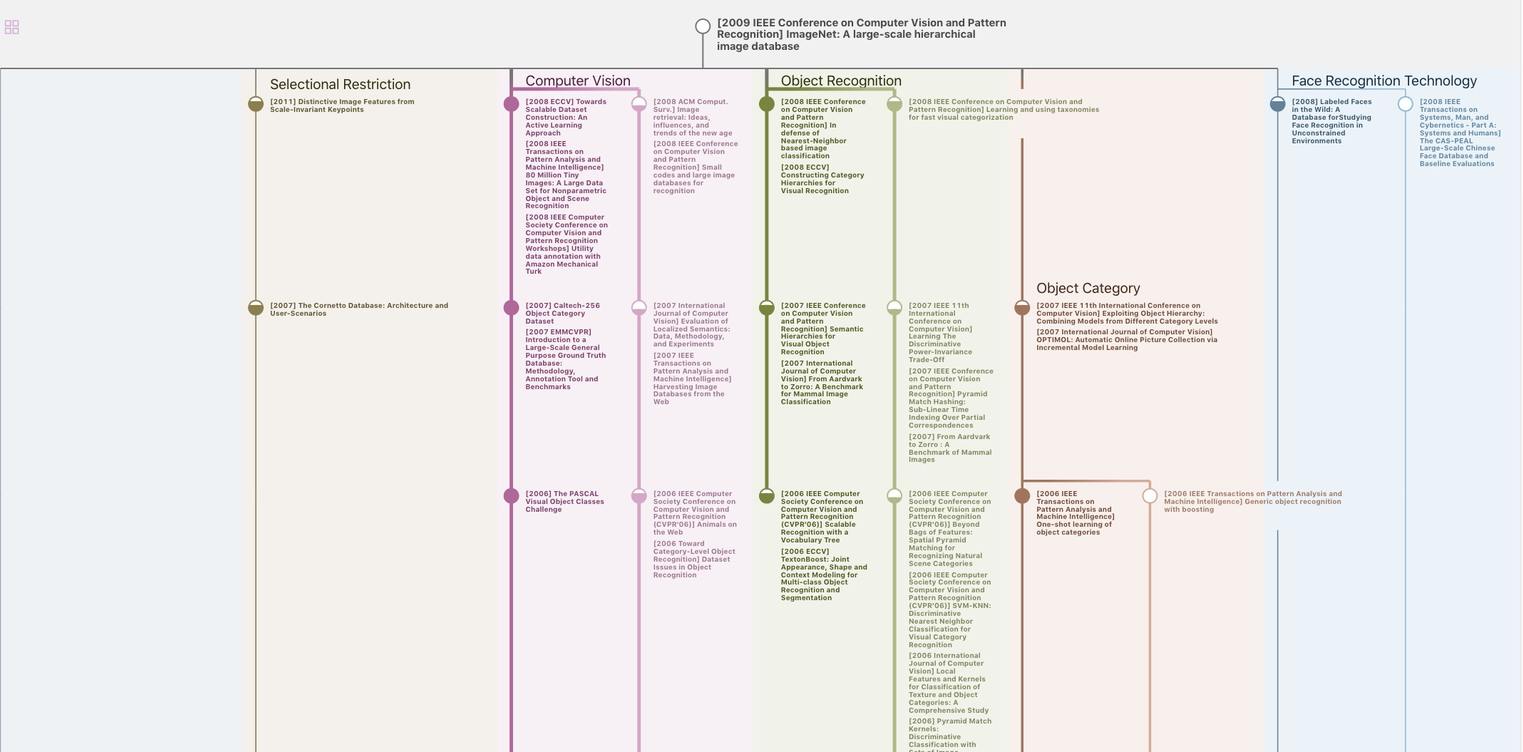
生成溯源树,研究论文发展脉络
Chat Paper
正在生成论文摘要