Improving Deep Metric Learning with Virtual Classes and Examples Mining
arxiv(2022)
摘要
In deep metric learning, the training procedure relies on sampling informative tuples. However, as the training procedure progresses, it becomes nearly impossible to sample relevant hard negative examples without proper mining strategies or generation-based methods. Recent work on hard negative generation have shown great promises to solve the mining problem. However, this generation process is difficult to tune and often leads to incorrectly labeled examples. To tackle this issue, we introduce MIRAGE, a generation-based method that relies on virtual classes entirely composed of generated examples that act as buffer areas between the training classes. We empirically show that virtual classes significantly improve the results on popular datasets (Cub-200-2011 and Cars-196) compared to other generation methods.
更多查看译文
关键词
image retrieval,metric learning,example mining,virtual classes,example generation
AI 理解论文
溯源树
样例
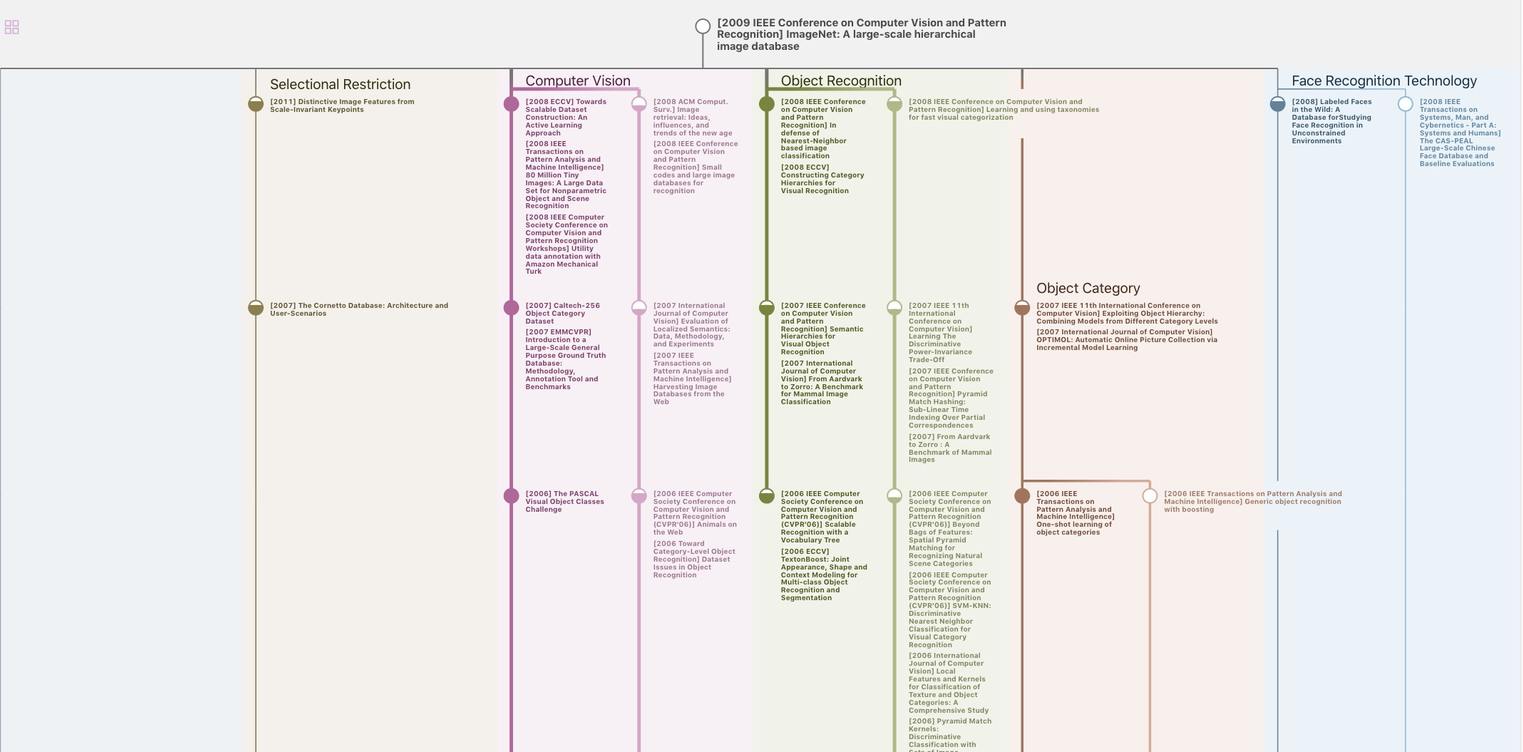
生成溯源树,研究论文发展脉络
Chat Paper
正在生成论文摘要