Learning not to Discriminate: Task Agnostic Learning for Improving Monolingual and Code-switched Speech Recognition
arxiv(2020)
摘要
Recognizing code-switched speech is challenging for Automatic Speech Recognition (ASR) for a variety of reasons, including the lack of code-switched training data. Recently, we showed that monolingual ASR systems fine-tuned on code-switched data deteriorate in performance on monolingual speech recognition, which is not desirable as ASR systems deployed in multilingual scenarios should recognize both monolingual and code-switched speech with high accuracy. Our experiments indicated that this loss in performance could be mitigated by using certain strategies for fine-tuning and regularization, leading to improvements in both monolingual and code-switched ASR. In this work, we present further improvements over our previous work by using domain adversarial learning to train task agnostic models. We evaluate the classification accuracy of an adversarial discriminator and show that it can learn shared layer parameters that are task agnostic. We train end-to-end ASR systems starting with a pooled model that uses monolingual and code-switched data along with the adversarial discriminator. Our proposed technique leads to reductions in Word Error Rates (WER) in monolingual and code-switched test sets across three language pairs.
更多查看译文
关键词
task agnostic learning,monolingual,speech,recognition,code-switched
AI 理解论文
溯源树
样例
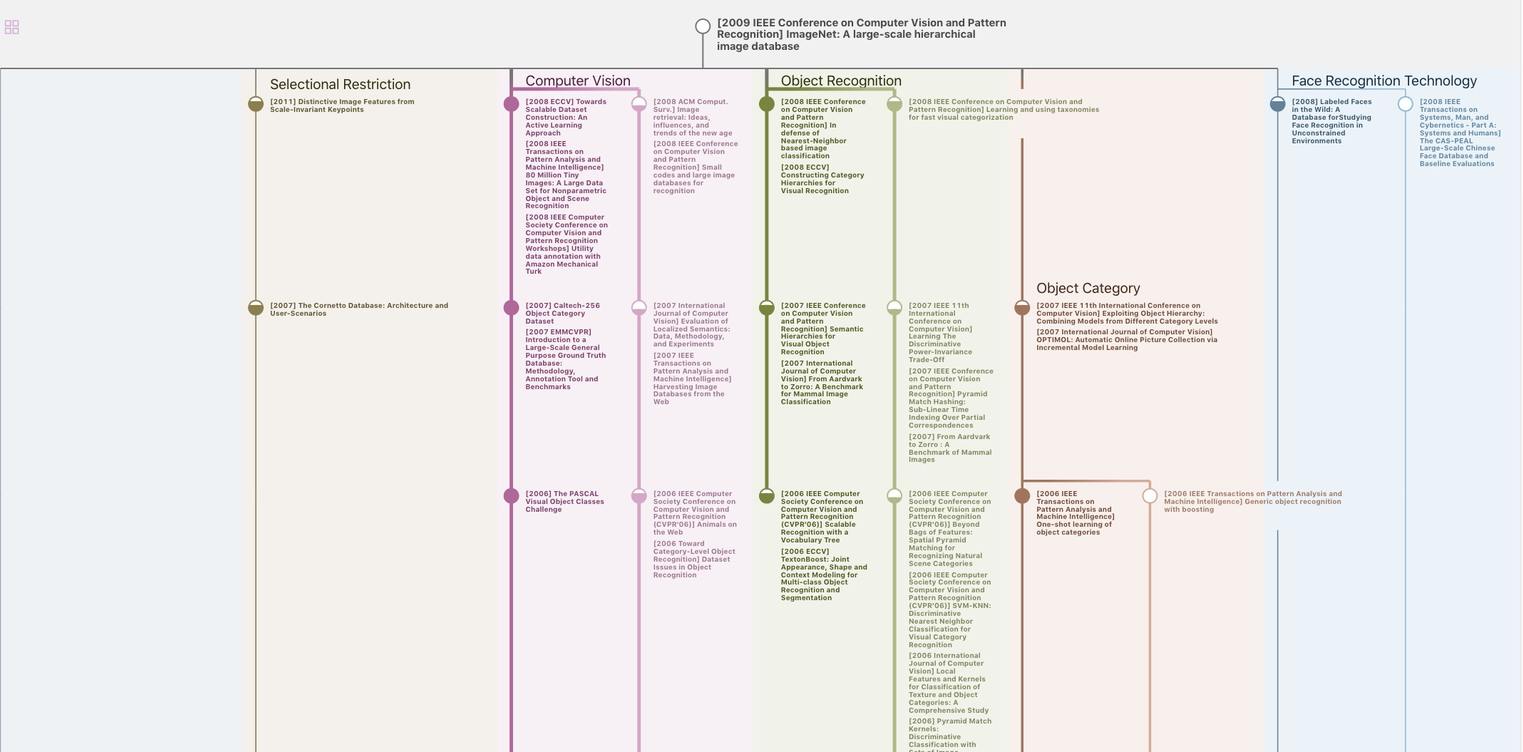
生成溯源树,研究论文发展脉络
Chat Paper
正在生成论文摘要