Hematologist-Level Classification of Mature B-Cell Neoplasm Using Deep Learning on Multiparameter Flow Cytometry Data.
CYTOMETRY PART A(2020)
摘要
The wealth of information captured by multiparameter flow cytometry (MFC) can be analyzed by recent methods of computer vision when represented as a single image file. We therefore transformed MFC raw data into a multicolor 2D image by a self-organizing map and classified this representation using a convolutional neural network. By this means, we built an artificial intelligence that is not only able to distinguish diseased from healthy samples, but it can also differentiate seven subtypes of mature B-cell neoplasm. We trained our model with 18,274 cases including chronic lymphocytic leukemia and its precursor monoclonal B-cell lymphocytosis, marginal zone lymphoma, mantle cell lymphoma, prolymphocytic leukemia, follicular lymphoma, hairy cell leukemia, lymphoplasmacytic lymphoma and achieved a weighted F1 score of 0.94 on a separate test set of 2,348 cases. Furthermore, we estimated the trustworthiness of a classification and could classify 70% of all cases with a confidence of 0.95 and higher. Our performance analyses indicate that particularly for rare subtypes further improvement can be expected when even more samples are available for training. (c) 2020 The Authors. Cytometry Part A published by Wiley Periodicals, Inc. on behalf of International Society for Advancement of Cytometry.
更多查看译文
关键词
deep learning,self-organizing maps,non-Hodgkin lymphoma
AI 理解论文
溯源树
样例
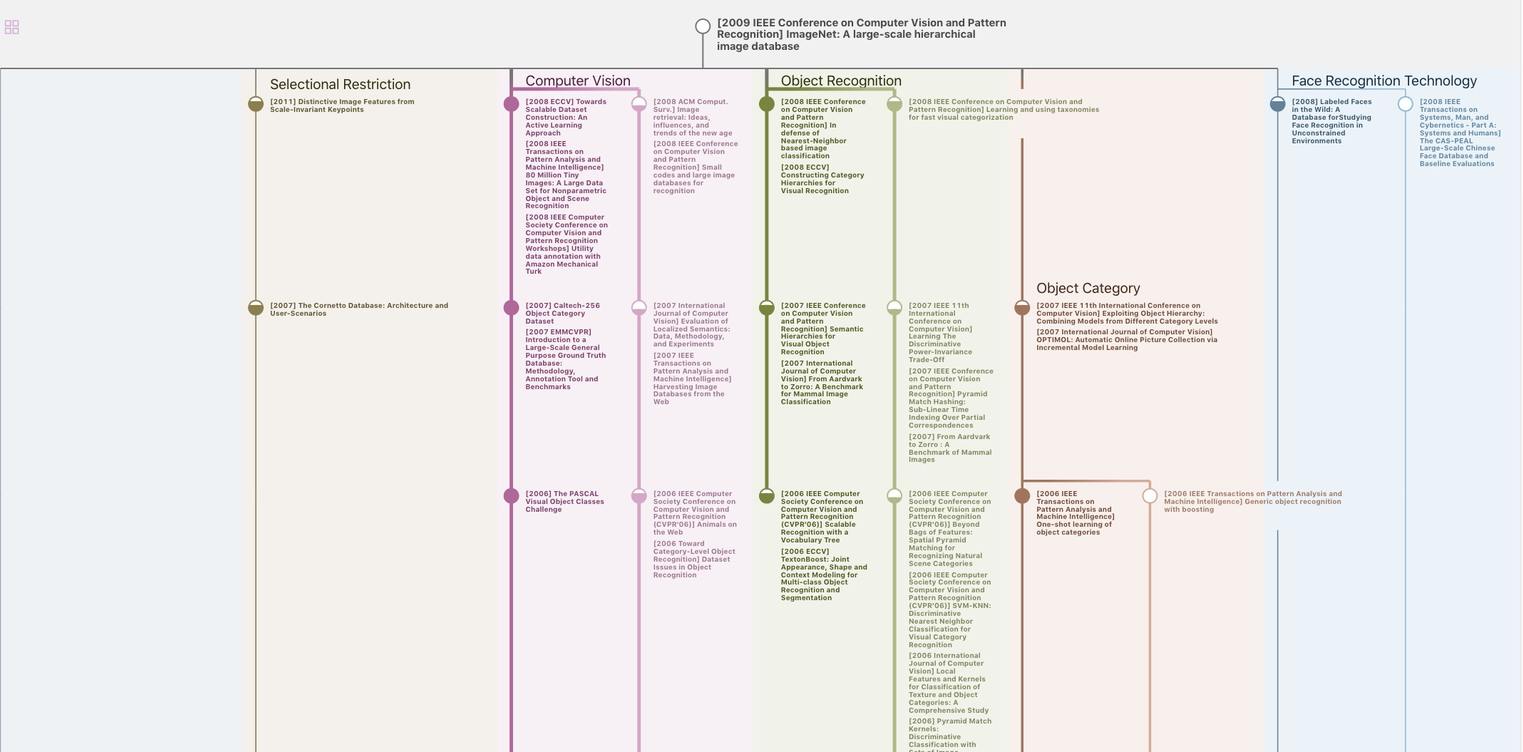
生成溯源树,研究论文发展脉络
Chat Paper
正在生成论文摘要