A semi-supervised joint learning approach to left ventricular segmentation and motion tracking in echocardiography.
2020 IEEE 17TH INTERNATIONAL SYMPOSIUM ON BIOMEDICAL IMAGING (ISBI 2020)(2020)
摘要
Accurate interpretation and analysis of echocardiography is important in assessing cardiovascular health. However, motion tracking often relies on accurate segmentation of the myocardium, which can be difficult to obtain due to inherent ultrasound properties. In order to address this limitation, we propose a semi-supervised joint learning network that exploits overlapping features in motion tracking and segmentation. The network simultaneously trains two branches: one for motion tracking and one for segmentation. Each branch learns to extract features relevant to their respective tasks and shares them with the other. Learned motion estimations propagate a manually segmented mask through time, which is used to guide future segmentation predictions. Physiological constraints are introduced to enforce realistic cardiac behavior. Experimental results on synthetic and in vivo canine 2D+t echocardiographic sequences outperform some competing methods in both tasks.
更多查看译文
关键词
Deep Learning,Echocardiography,Motion Tracking,Segmentation,Semi-Supervised
AI 理解论文
溯源树
样例
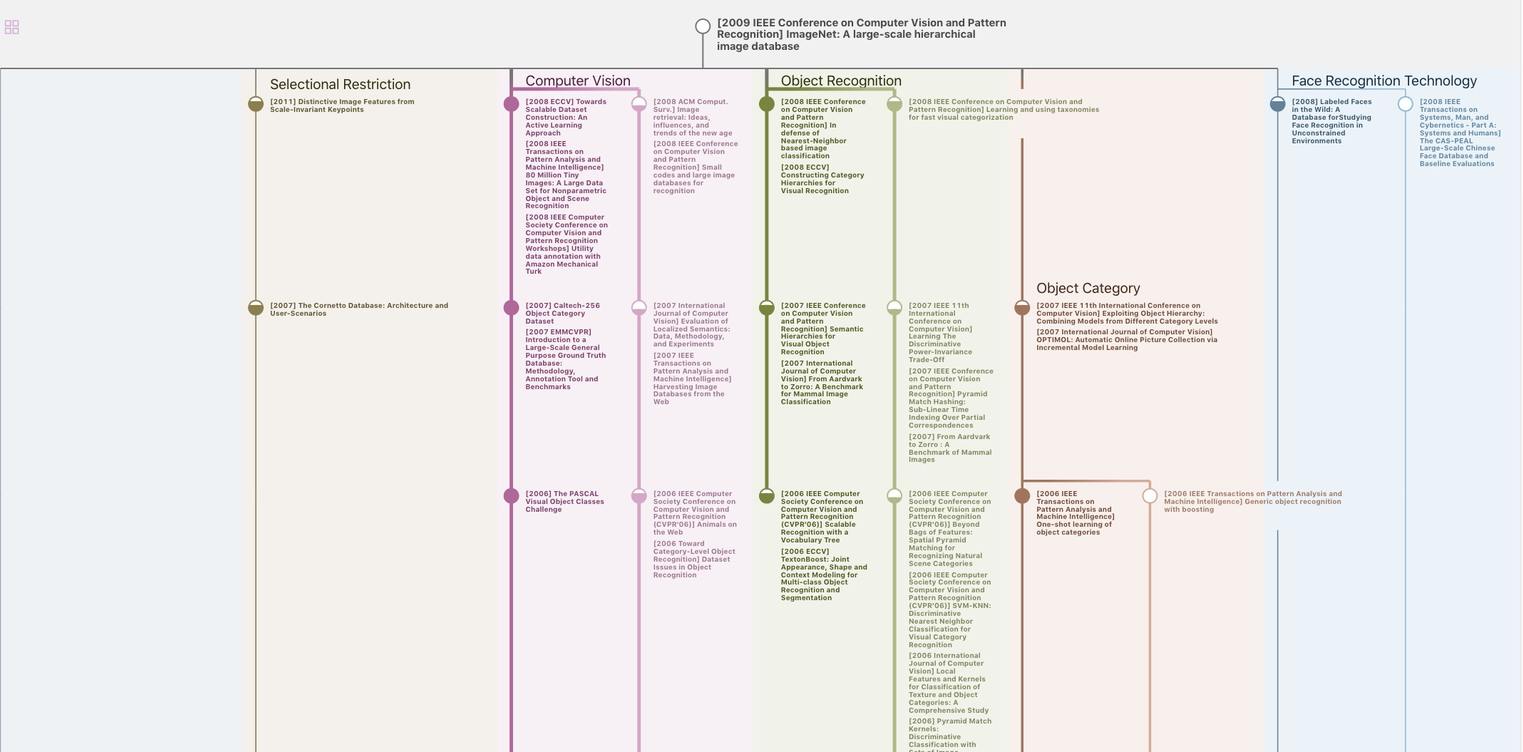
生成溯源树,研究论文发展脉络
Chat Paper
正在生成论文摘要