Hybrid Control for Learning Motor Skills.
WAFR(2021)
摘要
We develop a hybrid control approach for robot learning based on combining learned predictive models with experience-based state-action policy mappings to improve the learning capabilities of robotic systems. Predictive models provide an understanding of the task and the physics (which improves sample-efficiency), while experience-based policy mappings are treated as "muscle memory" that encode favorable actions as experiences that override planned actions. Hybrid control tools are used to create an algorithmic approach for combining learned predictive models with experience-based learning. Hybrid learning is presented as a method for efficiently learning motor skills by systematically combining and improving the performance of predictive models and experience-based policies. A deterministic variation of hybrid learning is derived and extended into a stochastic implementation that relaxes some of the key assumptions in the original derivation. Each variation is tested on experience-based learning methods (where the robot interacts with the environment to gain experience) as well as imitation learning methods (where experience is provided through demonstrations and tested in the environment). The results show that our method is capable of improving the performance and sample-efficiency of learning motor skills in a variety of experimental domains.
更多查看译文
关键词
Hybrid control theory, Optimal control, Learning theory
AI 理解论文
溯源树
样例
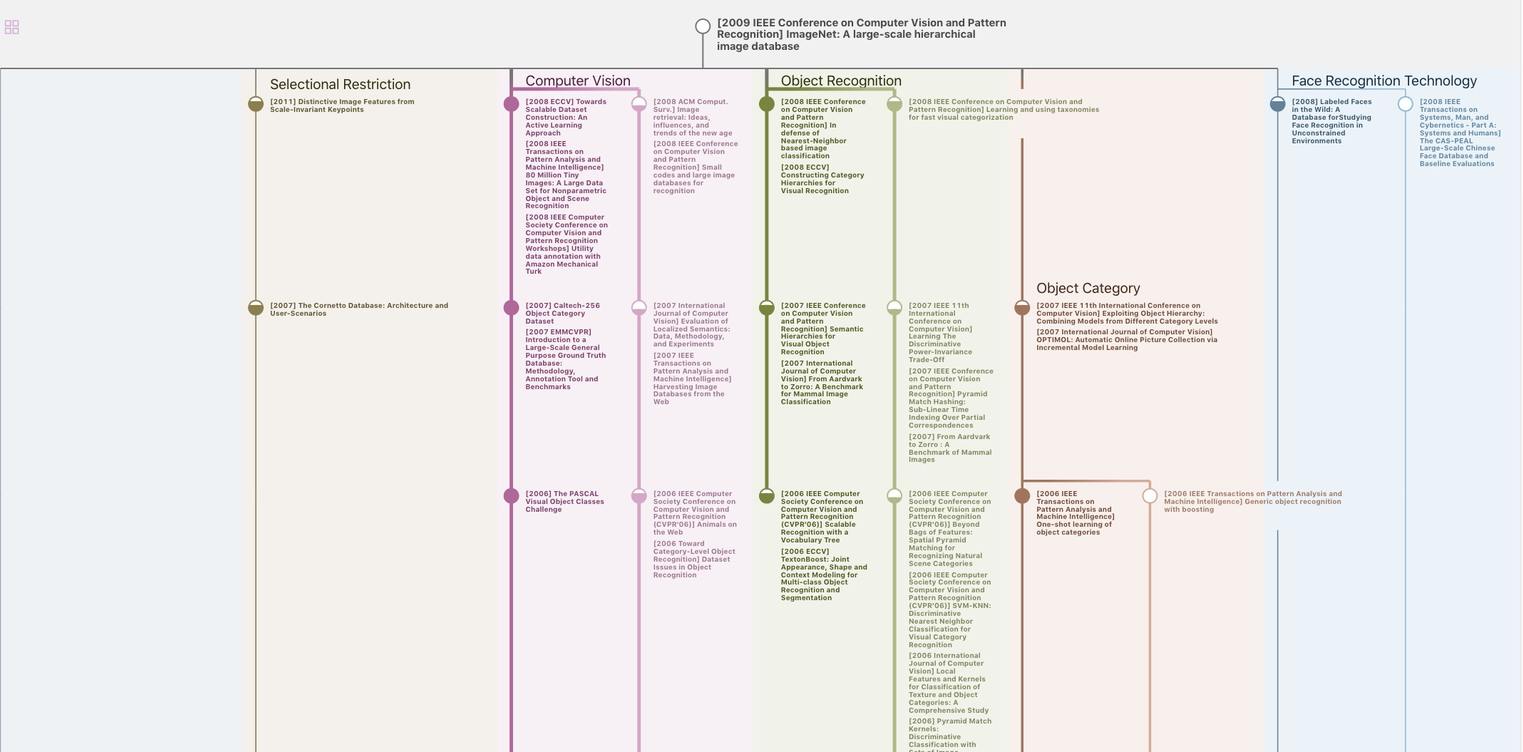
生成溯源树,研究论文发展脉络
Chat Paper
正在生成论文摘要