Near Input Sparsity Time Kernel Embeddings via Adaptive Sampling
international conference on machine learning(2020)
摘要
To accelerate kernel methods, we propose a near input sparsity time algorithm for sampling the high-dimensional feature space implicitly defined by a kernel transformation. Our main contribution is an importance sampling method for subsampling the feature space of a degree $q$ tensoring of data points in almost input sparsity time, improving the recent oblivious sketching method of (Ahle et al., 2020) by a factor of $q^{5/2}/\epsilon^2$. This leads to a subspace embedding for the polynomial kernel, as well as the Gaussian kernel, with a target dimension that is only linearly dependent on the statistical dimension of the kernel and in time which is only linearly dependent on the sparsity of the input dataset. We show how our subspace embedding bounds imply new statistical guarantees for kernel ridge regression. Furthermore, we empirically show that in large-scale regression tasks, our algorithm outperforms state-of-the-art kernel approximation methods.
更多查看译文
关键词
adaptive sampling,time
AI 理解论文
溯源树
样例
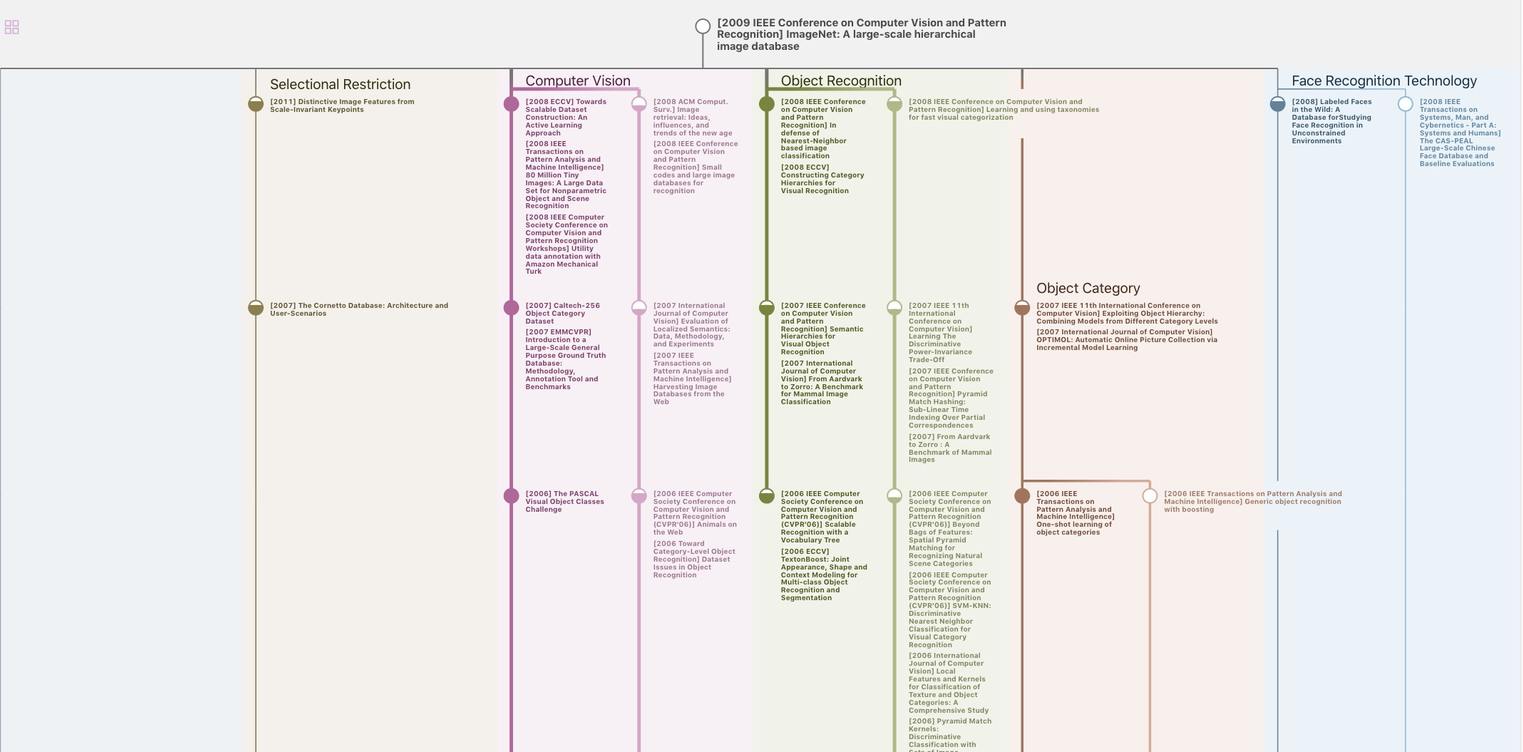
生成溯源树,研究论文发展脉络
Chat Paper
正在生成论文摘要