Towards Few-Shot Event Mention Retrieval - An Evaluation Framework and A Siamese Network Approach.
LREC(2020)
摘要
Automatically analyzing events in a large amount of text is crucial for situation awareness and decision making. Previous approaches treat event extraction as "one size fits all" with an ontology defined a priori. The resulted extraction models are built just for extracting those types in the ontology. These approaches cannot be easily adapted to new event types nor new domains of interest. To accommodate personalized event-centric information needs, this paper introduces the few-shot Event Mention Retrieval (EMR) task: given a user-supplied query consisting of a handful of event mentions, return relevant event mentions found in a corpus. This formulation enables "query by example", which drastically lowers the bar of specifying event-centric information needs. The retrieval setting also enables fuzzy search. We present an evaluation framework leveraging existing event datasets such as ACE. We also develop a Siamese Network approach, and show that it performs better than ad-hoc retrieval models in the few-shot EMR setting.
更多查看译文
关键词
Information Extraction, Information Retrieval, Evaluation Methodologies
AI 理解论文
溯源树
样例
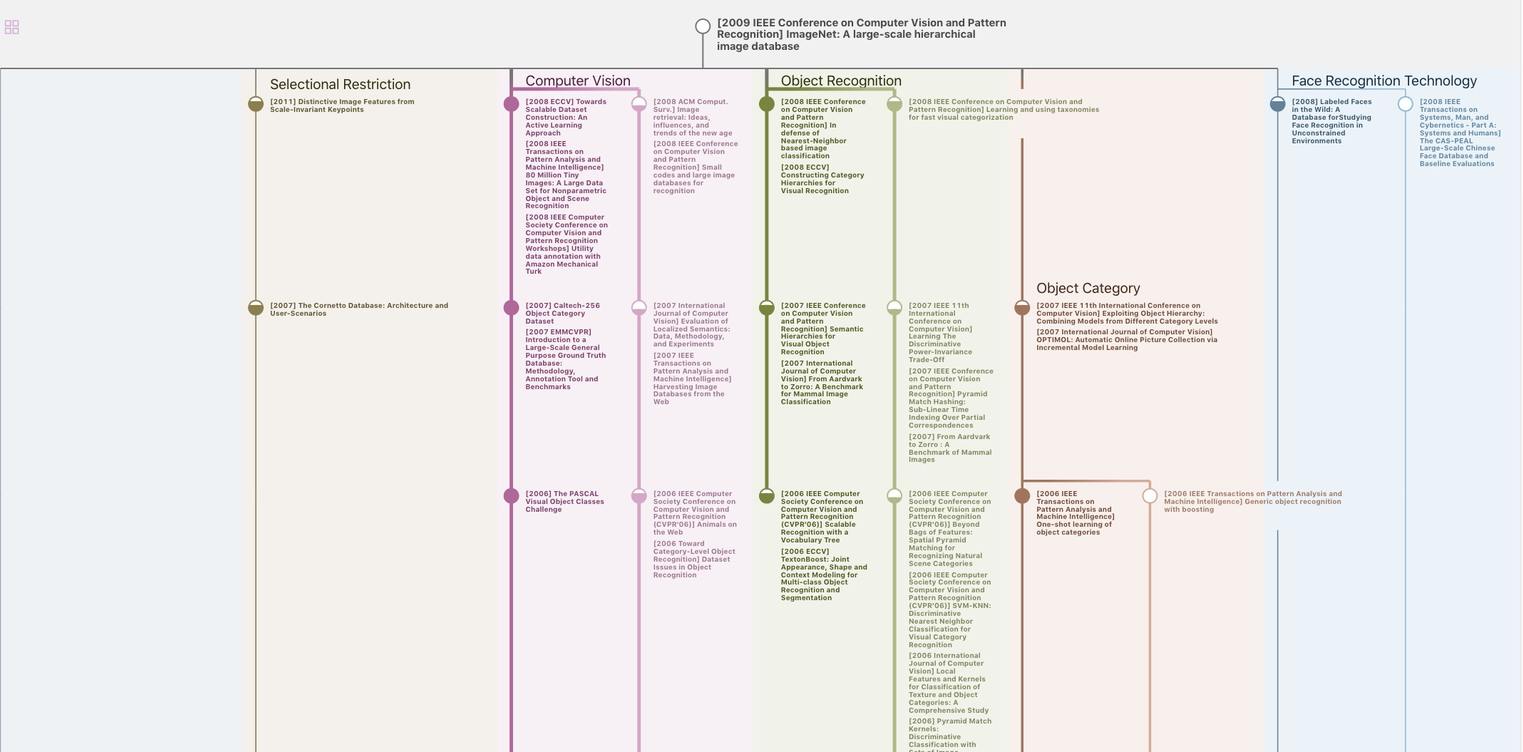
生成溯源树,研究论文发展脉络
Chat Paper
正在生成论文摘要