Joint Learning of Syntactic Features Helps Discourse Segmentation.
LREC(2020)
摘要
This paper describes an accurate framework for carrying out multi-lingual discourse segmentation with BERT (Devlin et al., 2019). The model is trained to identify segments by casting the problem as a token classification problem and jointly learning syntactic features like part-of-speech tags and dependency relations. This leads to significant improvements in performance. Experiments are performed in different languages, such as English, Dutch, German, Portuguese Brazilian and Basque to highlight the cross-lingual effectiveness of the segmenter. In particular, the model achieves a state-of-the-art F-score of 96.7 for the RST-DT corpus (Carlson et al., 2003) improving on the previous best model by 7.2%. Additionally, a qualitative explanation is provided for how proposed changes contribute to model performance by analyzing errors made on the test data.
更多查看译文
关键词
syntactic features,segmentation,learning
AI 理解论文
溯源树
样例
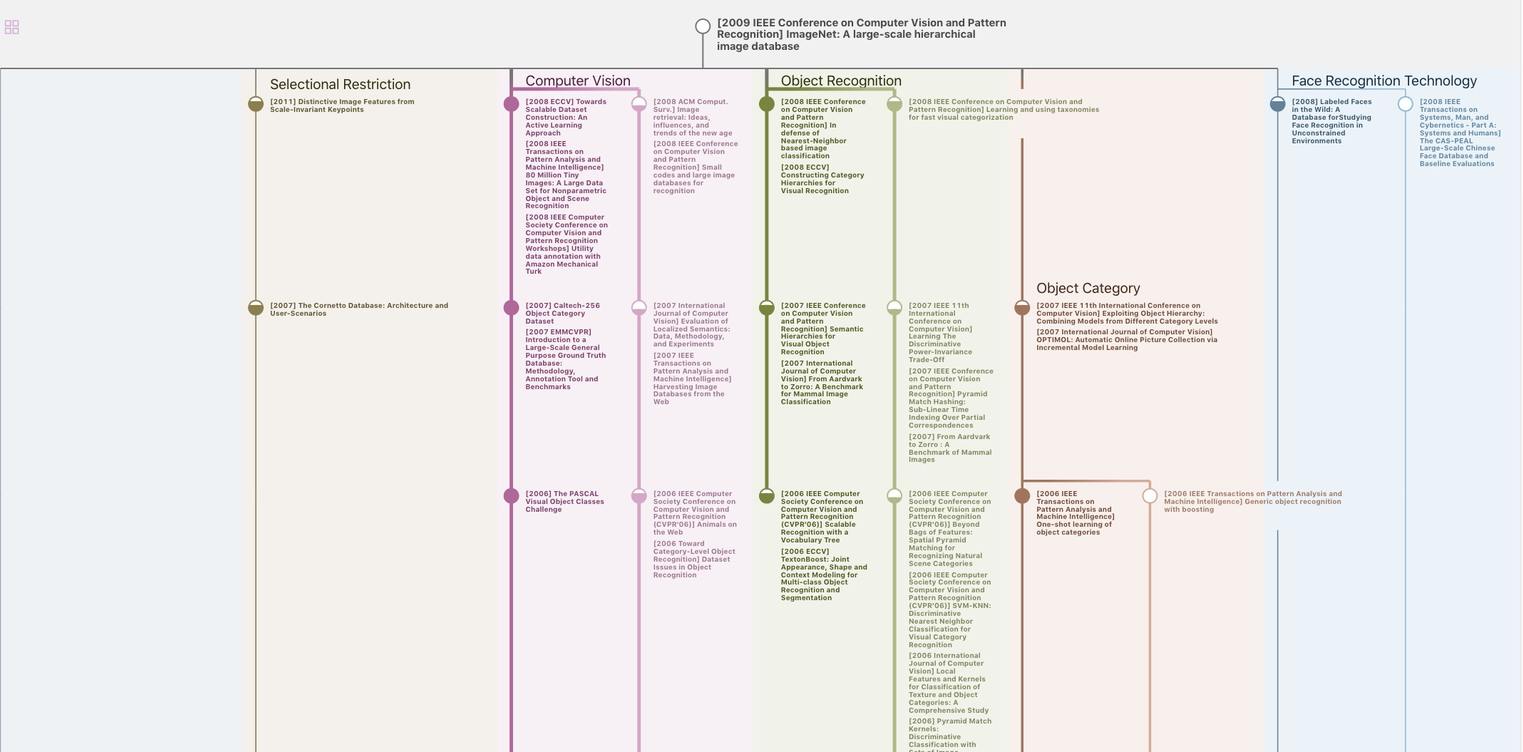
生成溯源树,研究论文发展脉络
Chat Paper
正在生成论文摘要