Cascade Model-based Propensity Estimation for Counterfactual Learning to Rank
SIGIR '20: The 43rd International ACM SIGIR conference on research and development in Information Retrieval Virtual Event China July, 2020(2020)
摘要
Unbiased counterfactual learning to rank (CLTR) requires click propensities to compensate for the difference between user clicks and true relevance of search results via inverse propensity scoring (IPS). Current propensity estimation methods assume that user click behavior follows the position-based click model (PBM) and estimate click propensities based on this assumption. However, in reality, user clicks often follow the cascade model (CM), where users scan search results from top to bottom and where each next click depends on the previous one. In this cascade scenario, PBM-based estimates of propensities are not accurate, which, in turn, hurts CLTR performance. In this paper, we propose a propensity estimation method for the cascade scenario, called cascade model-based inverse propensity scoring (CM-IPS). We show that CM-IPS keeps CLTR performance close to the full-information performance in case the user clicks follow the CM, while PBM-based CLTR has a significant gap towards the full-information. The opposite is true if the user clicks follow PBM instead of the CM. Finally, we suggest a way to select between CM- and PBM-based propensity estimation methods based on historical user clicks.
更多查看译文
AI 理解论文
溯源树
样例
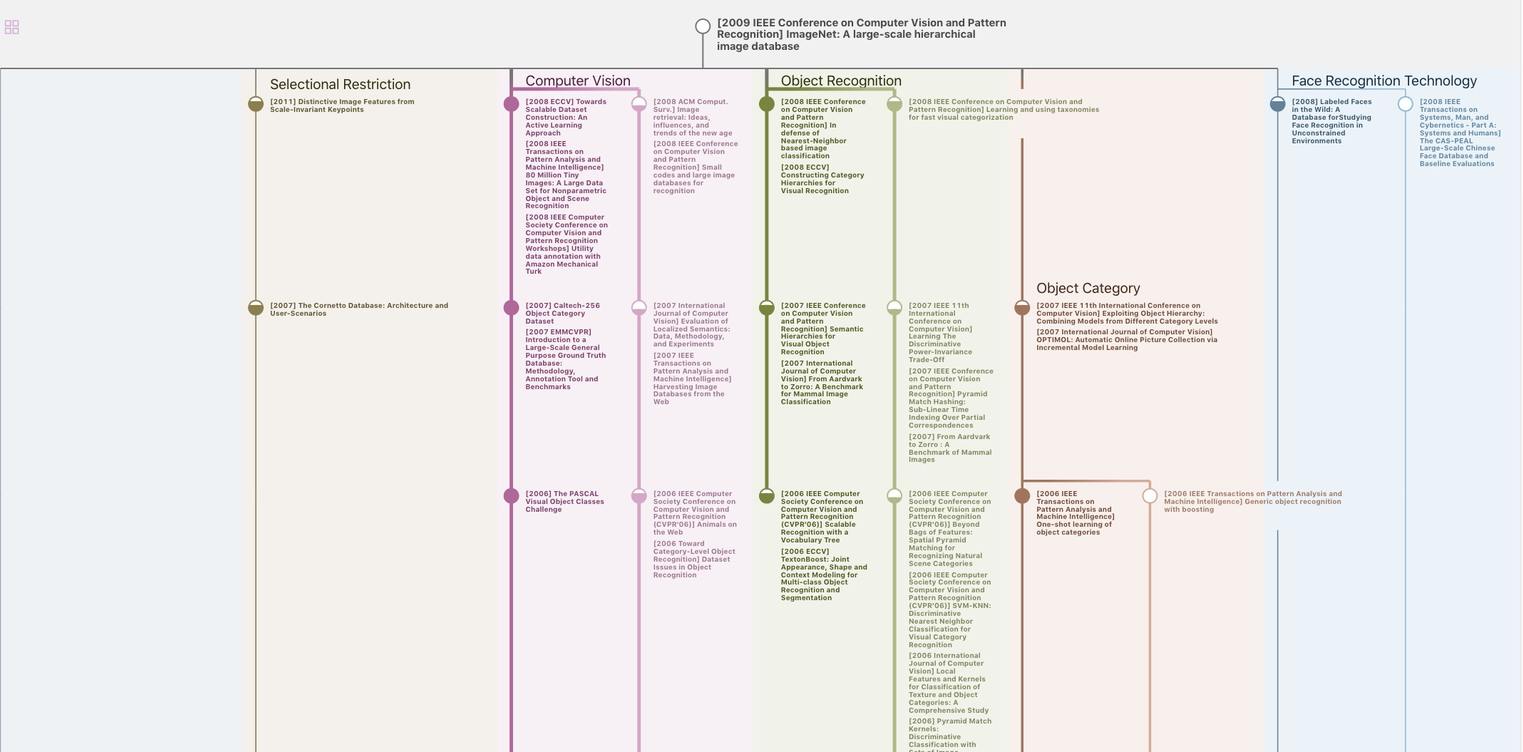
生成溯源树,研究论文发展脉络
Chat Paper
正在生成论文摘要