Diverse Image Generation via Self-Conditioned GANs
CVPR(2020)
摘要
We introduce a simple but effective unsupervised method for generating realistic and diverse images. We train a class-conditional GAN model without using manually annotated class labels. Instead, our model is conditional on labels automatically derived from clustering in the discriminator's feature space. Our clustering step automatically discovers diverse modes, and explicitly requires the generator to cover them. Experiments on standard mode collapse benchmarks show that our method outperforms several competing methods when addressing mode collapse. Our method also performs well on large-scale datasets such as ImageNet and Places365, improving both image diversity and standard quality metrics, compared to previous methods.
更多查看译文
关键词
generation,image,self-conditioned
AI 理解论文
溯源树
样例
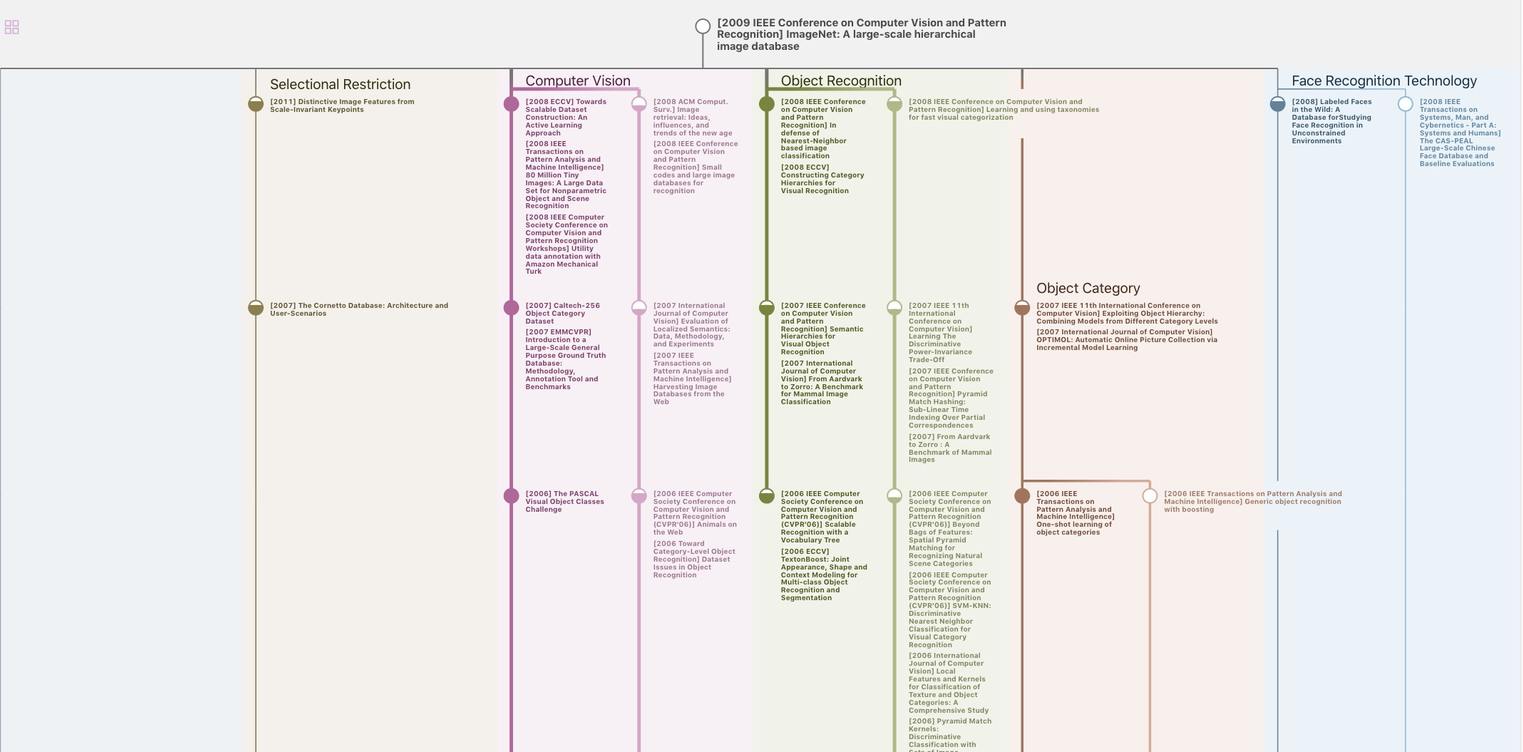
生成溯源树,研究论文发展脉络
Chat Paper
正在生成论文摘要