Self-Supervised Learning of Interpretable Keypoints From Unlabelled Videos
CVPR(2020)
摘要
We propose a new method for recognizing the pose of objects from a single image that for learning uses only unlabelled videos and a weak empirical prior on the object poses. Video frames differ primarily in the pose of the objects they contain, so our method distils the pose information by analyzing the differences between frames. The distillation uses a new dual representation of the geometry of objects as a set of 2D keypoints, and as a pictorial representation, i.e. a skeleton image. This has three benefits: (1) it provides a tight \u0027geometric bottleneck\u0027 which disentangles pose from appearance, (2) it can leverage powerful image-to-image translation networks to map between photometry and geometry, and (3) it allows to incorporate empirical pose priors in the learning process. The pose priors are obtained from unpaired data, such as from a different dataset or modality such as mocap, such that no annotated image is ever used in learning the pose recognition network. In standard benchmarks for pose recognition for humans and faces, our method achieves state-of-the-art performance among methods that do not require any labelled images for training. Project page: http://www.robots.ox.ac.uk/ vgg/research/unsupervised_pose/
更多查看译文
关键词
tight geometric bottleneck,learning process,geometry,photometry,image-to-image translation networks,skeleton image,pictorial representation,dual representation,distillation,method distils,video frames,single image,unlabelled videos,interpretable keypoints,supervised learning,labelled images,recognition network,annotated image
AI 理解论文
溯源树
样例
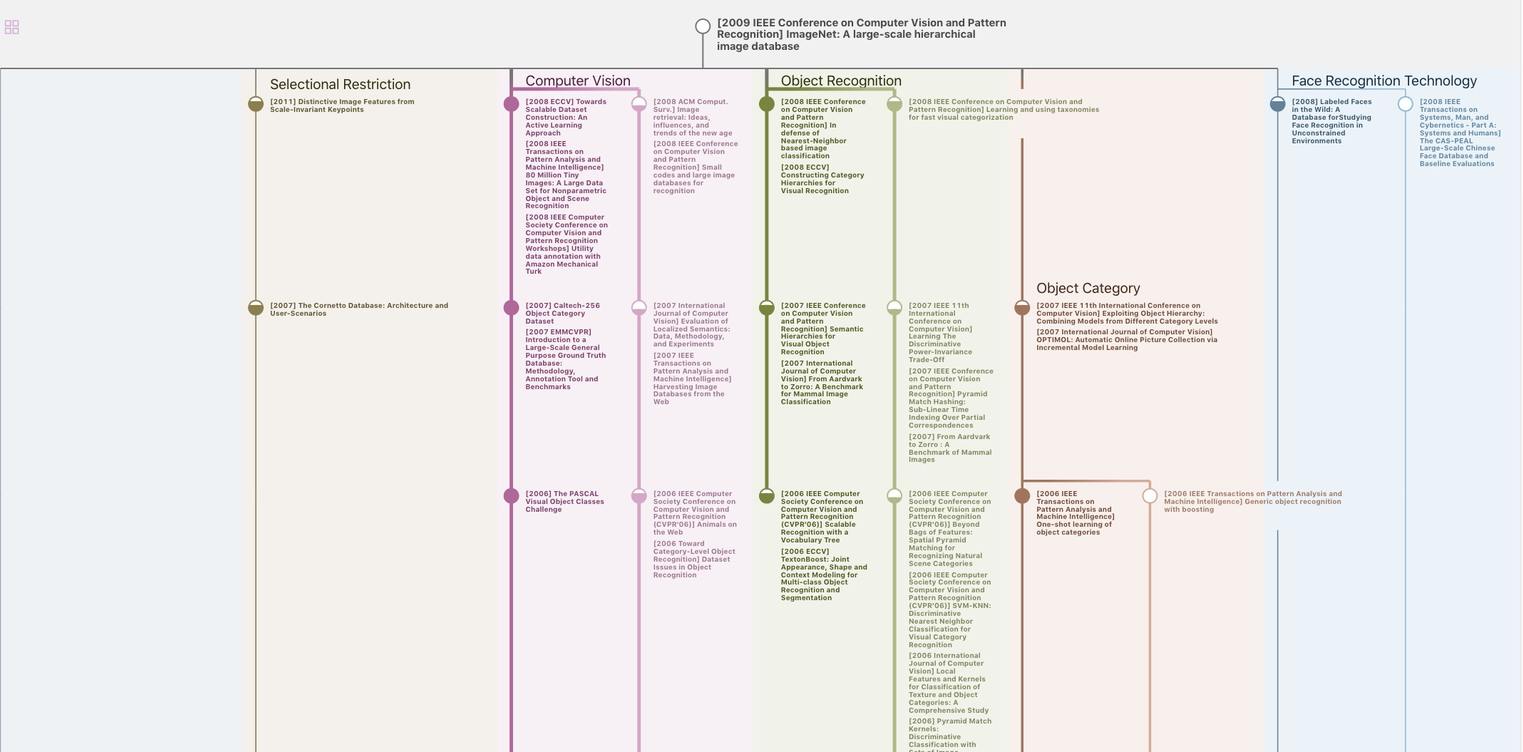
生成溯源树,研究论文发展脉络
Chat Paper
正在生成论文摘要