Nonparametric Object and Parts Modeling With Lie Group Dynamics
CVPR(2020)
摘要
Articulated motion analysis often utilizes strong prior knowledge such as a known or trained parts model for humans. Yet, the world contains a variety of articulating objects--mammals, insects, mechanized structures--where the number and configuration of parts for a particular object is unknown in advance. Here, we relax such strong assumptions via an unsupervised, Bayesian nonparametric parts model that infers an unknown number of parts with motions coupled by a body dynamic and parameterized by SE(D), the Lie group of rigid transformations. We derive an inference procedure that utilizes short observation sequences (image, depth, point cloud or mesh) of an object in motion without need for markers or learned body models. Efficient Gibbs decompositions for inference over distributions on SE(D) demonstrate robust part decompositions of moving objects under both 3D and 2D observation models. The inferred representation permits novel analysis, such as object segmentation by relative part motion, and transfers to new observations of the same object type.
更多查看译文
关键词
Lie group dynamics,articulated motion analysis,unsupervised Bayesian nonparametric parts model,inference procedure,point cloud,2D observation models,object segmentation,nonparametric object modeling,Gibbs decompositions,parts modeling,3D observation models
AI 理解论文
溯源树
样例
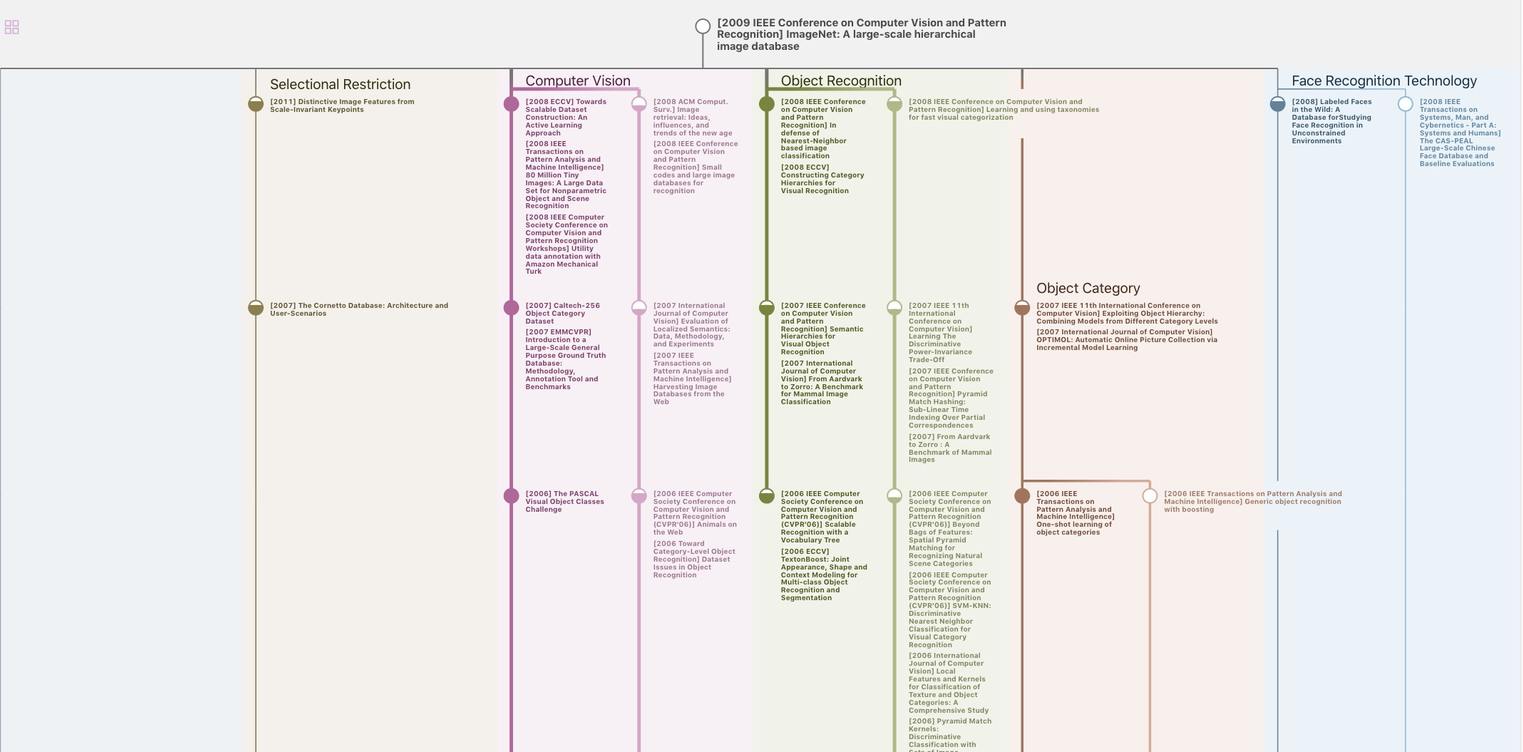
生成溯源树,研究论文发展脉络
Chat Paper
正在生成论文摘要